A Continuing Process Of Identifying Collecting Analyzing
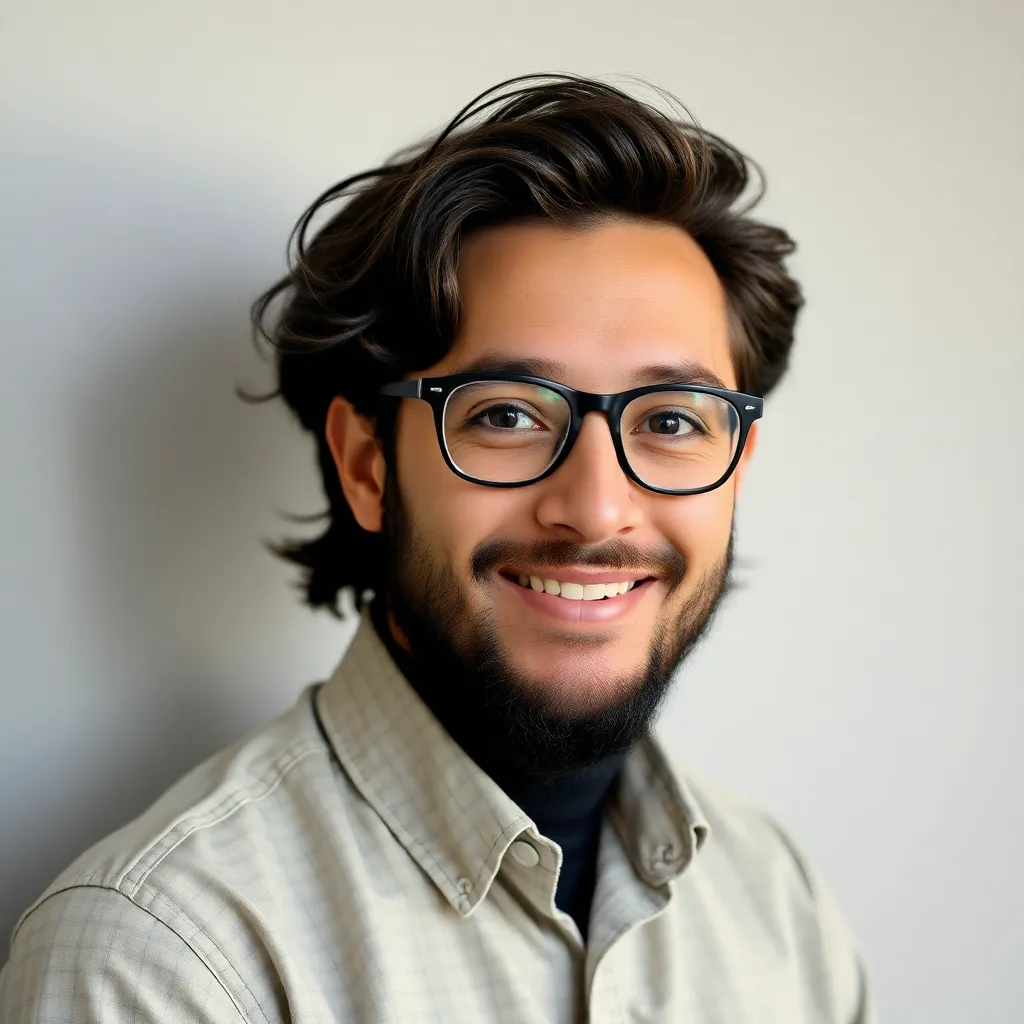
Breaking News Today
May 11, 2025 · 7 min read
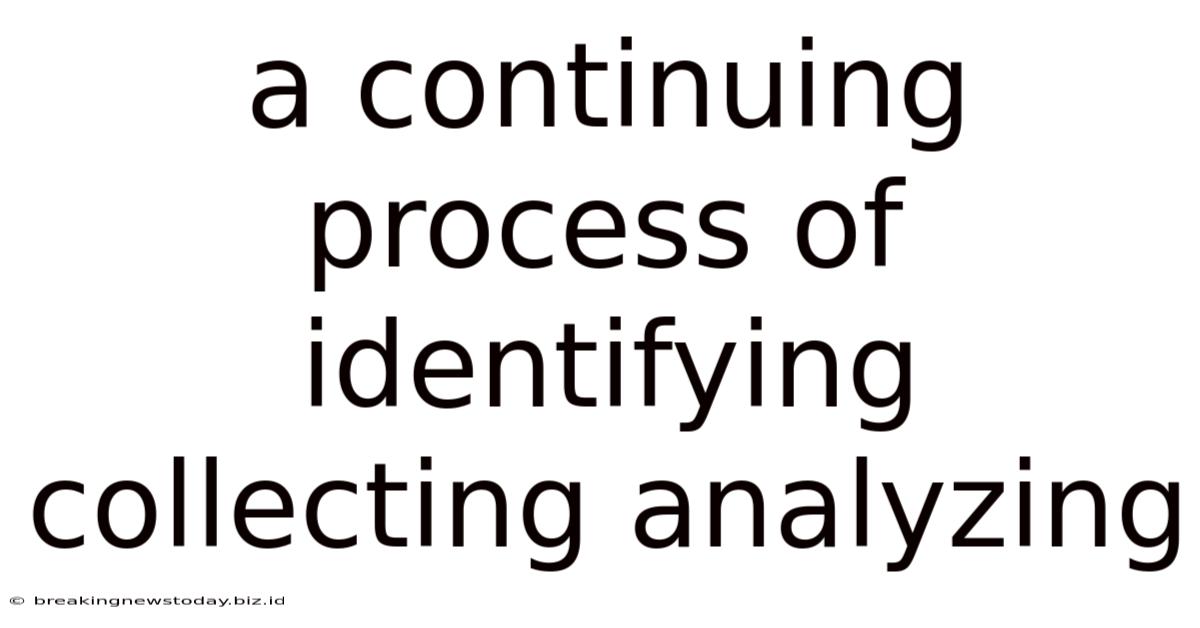
Table of Contents
The Ongoing Symphony of Identify, Collect, Analyze: A Deep Dive into Continuous Improvement
The modern world thrives on data. From optimizing marketing campaigns to predicting equipment failures, the ability to identify, collect, and analyze information forms the bedrock of progress across countless industries. But this isn't a one-time process; it's a continuous loop, a dynamic symphony where each movement informs the next. This article will explore this ongoing process, examining the intricacies of each stage and how their iterative interplay drives innovation and efficiency.
Identifying the Right Data: The Foundation of Insight
The initial step, identification, is crucial. It's not merely about gathering data; it's about strategically selecting the data points that will yield the most valuable insights. This requires a deep understanding of your goals and the questions you're trying to answer. A poorly defined identification phase can lead to wasted resources and ultimately, inaccurate conclusions.
Defining Clear Objectives: The Guiding Star
Before embarking on data collection, clearly articulate your objectives. What are you hoping to achieve? Are you aiming to:
- Improve efficiency? Identifying bottlenecks in your workflow requires different data than, say, improving customer satisfaction.
- Enhance customer experience? Tracking customer feedback, website analytics, and support tickets are crucial here.
- Reduce costs? Analyzing operational expenses, resource allocation, and supply chain data becomes paramount.
- Boost sales? Focusing on marketing campaign performance, sales conversion rates, and customer demographics will be key.
Strong, specific objectives translate into targeted data collection, minimizing noise and maximizing the relevance of your findings. Vague goals lead to unstructured data collection, making analysis difficult and hindering insightful interpretation.
Identifying Relevant Data Sources: The Rich Tapestry of Information
Once your objectives are clear, identify the sources that hold the relevant data. These sources can be diverse and varied, including:
- Internal Systems: CRM databases, ERP systems, sales platforms, and internal communications tools. These provide a rich tapestry of information about internal processes and performance.
- External Sources: Market research reports, competitor analyses, social media listening tools, and customer surveys. These provide insights into the broader market landscape and customer sentiment.
- Machine Data: Sensors, IoT devices, and industrial equipment generate vast amounts of data, offering real-time insights into operational efficiency and potential failures.
- Human Interactions: Customer feedback forms, support tickets, employee surveys, and interviews provide invaluable qualitative data that complements quantitative data.
Careful consideration must be given to the reliability and validity of each source. Data quality is paramount; inaccurate or incomplete data will skew your analysis and lead to flawed conclusions.
Collecting Data: The Art of Accurate Acquisition
Once you've identified the relevant data sources, the next stage involves meticulous data collection. This phase requires careful planning and the implementation of robust data acquisition methods. Accuracy and completeness are paramount; even small errors can significantly impact the final analysis.
Data Acquisition Methods: A Multifaceted Approach
The choice of data acquisition methods depends largely on the type and source of data. Common methods include:
- Automated Data Collection: This involves using software and tools to automatically collect data from various sources. This is particularly efficient for large datasets and repetitive tasks. Examples include web scraping tools, API integrations, and database queries.
- Manual Data Collection: This involves manually entering data, often from surveys, interviews, or physical documents. While time-consuming, it can be necessary for certain types of data.
- Sensor Data Collection: This involves using sensors to collect data from the physical world, such as temperature, pressure, or location. This is increasingly common in the Internet of Things (IoT) and industrial applications.
- API Integration: Connecting to external data sources via APIs allows for seamless, automated data transfer, enhancing efficiency and reducing manual effort.
Choosing the right method is critical for ensuring accurate and efficient data collection. Consider factors such as data volume, data type, data source accessibility, and available resources.
Ensuring Data Quality: Accuracy and Completeness
Data quality is a critical concern during the collection phase. Steps to ensure high-quality data include:
- Data Cleaning: This involves identifying and correcting errors, inconsistencies, and missing values in the dataset. Techniques include data validation, outlier detection, and imputation.
- Data Validation: Implementing checks and balances to verify the accuracy and consistency of collected data.
- Data Transformation: Converting data into a usable format for analysis. This might involve data normalization, standardization, and aggregation.
- Data Governance: Establishing clear rules and procedures for data handling, ensuring consistency and reliability across all stages.
Proactive measures to ensure data quality significantly reduce the time and effort spent on cleaning and correcting errors during the analysis phase.
Analyzing Data: Unlocking the Power of Insights
The final, and arguably most crucial, stage is data analysis. This involves using statistical methods and data visualization techniques to uncover patterns, trends, and insights within the collected data. The quality of your analysis directly impacts the quality of the decisions you make.
Choosing the Right Analytical Techniques: The Power of Statistical Methods
The choice of analytical techniques depends on the type of data, the research questions, and the desired insights. Common methods include:
- Descriptive Statistics: Summarizing and describing the main features of the data, including measures of central tendency (mean, median, mode) and dispersion (variance, standard deviation).
- Inferential Statistics: Making inferences about a population based on a sample of data. This includes hypothesis testing, regression analysis, and ANOVA.
- Predictive Analytics: Using statistical models to predict future outcomes based on historical data. This includes machine learning techniques such as regression, classification, and clustering.
- Data Mining: Discovering patterns and insights from large datasets using sophisticated algorithms.
The careful selection of analytical techniques is crucial for drawing meaningful conclusions from your data. Misapplying statistical methods can lead to inaccurate and misleading results.
Data Visualization: Communicating Insights Effectively
Data visualization plays a critical role in communicating analytical findings. Effective visualizations make complex data easier to understand and interpret, allowing stakeholders to grasp key insights quickly. Common visualization techniques include:
- Bar charts and pie charts: For displaying categorical data.
- Line graphs and scatter plots: For displaying trends and relationships between variables.
- Heat maps and treemaps: For visualizing large datasets and identifying patterns.
- Dashboards and interactive visualizations: For presenting dynamic and interactive views of data.
Visually appealing and informative visualizations are crucial for effective communication and decision-making. Avoid cluttered or misleading visualizations; prioritize clarity and accuracy.
The Continuous Loop: Iteration and Improvement
The process of identifying, collecting, and analyzing data isn't a linear sequence; it's a continuous loop. The insights gained from analysis inform future data collection and identification efforts, leading to ongoing improvement and refinement.
Feedback Loops: The Engine of Continuous Improvement
Feedback loops are essential for continuous improvement. The insights generated from data analysis should inform future data collection and identification strategies. This iterative process allows for:
- Refinement of Objectives: Adjusting research questions based on initial findings.
- Improvement of Data Collection Methods: Addressing gaps and inaccuracies in data collection.
- Development of New Analytical Techniques: Exploring more sophisticated methods to extract deeper insights.
- Enhanced Decision-Making: Using data-driven insights to inform strategic decisions.
Regularly reviewing and refining the process ensures that data analysis remains relevant and effective over time.
Adapting to Change: Staying Ahead of the Curve
The data landscape is constantly evolving. New data sources emerge, existing sources change, and analytical techniques become more sophisticated. To stay ahead of the curve, organizations must:
- Monitor Emerging Trends: Staying informed about new data sources and technologies.
- Embrace Technological Advancements: Adopting new tools and techniques to improve data analysis capabilities.
- Develop Adaptable Processes: Creating flexible frameworks that can accommodate changing data needs.
- Foster a Data-Driven Culture: Creating an environment where data analysis is valued and integrated into decision-making processes.
A proactive approach to adapting to change ensures the ongoing relevance and effectiveness of the identify, collect, analyze process.
Conclusion: The Power of Continuous Data Analysis
The continuous process of identifying, collecting, and analyzing data is the engine of innovation and improvement in today's data-driven world. By meticulously planning each stage, ensuring data quality, and embracing a culture of continuous improvement, organizations can unlock the immense potential of data to enhance efficiency, drive growth, and achieve their strategic goals. The iterative nature of this process, coupled with a commitment to adaptation and refinement, is what empowers businesses and organizations to thrive in a rapidly evolving landscape. The symphony of identify, collect, analyze plays on, constantly evolving and producing new melodies of insight.
Latest Posts
Latest Posts
-
You May Turn Left On A Red Light If
May 12, 2025
-
A Presidents Power Has Largely Depended On
May 12, 2025
-
The Aria Lucevan Le Stelle Is Composed For A An
May 12, 2025
-
Saving Information For Future Use Is Called
May 12, 2025
-
Respuestas Tarjetas De Preguntas De 100 Mexicanos Dijeron
May 12, 2025
Related Post
Thank you for visiting our website which covers about A Continuing Process Of Identifying Collecting Analyzing . We hope the information provided has been useful to you. Feel free to contact us if you have any questions or need further assistance. See you next time and don't miss to bookmark.