Accuracy In Forecasting Can Be Measured By
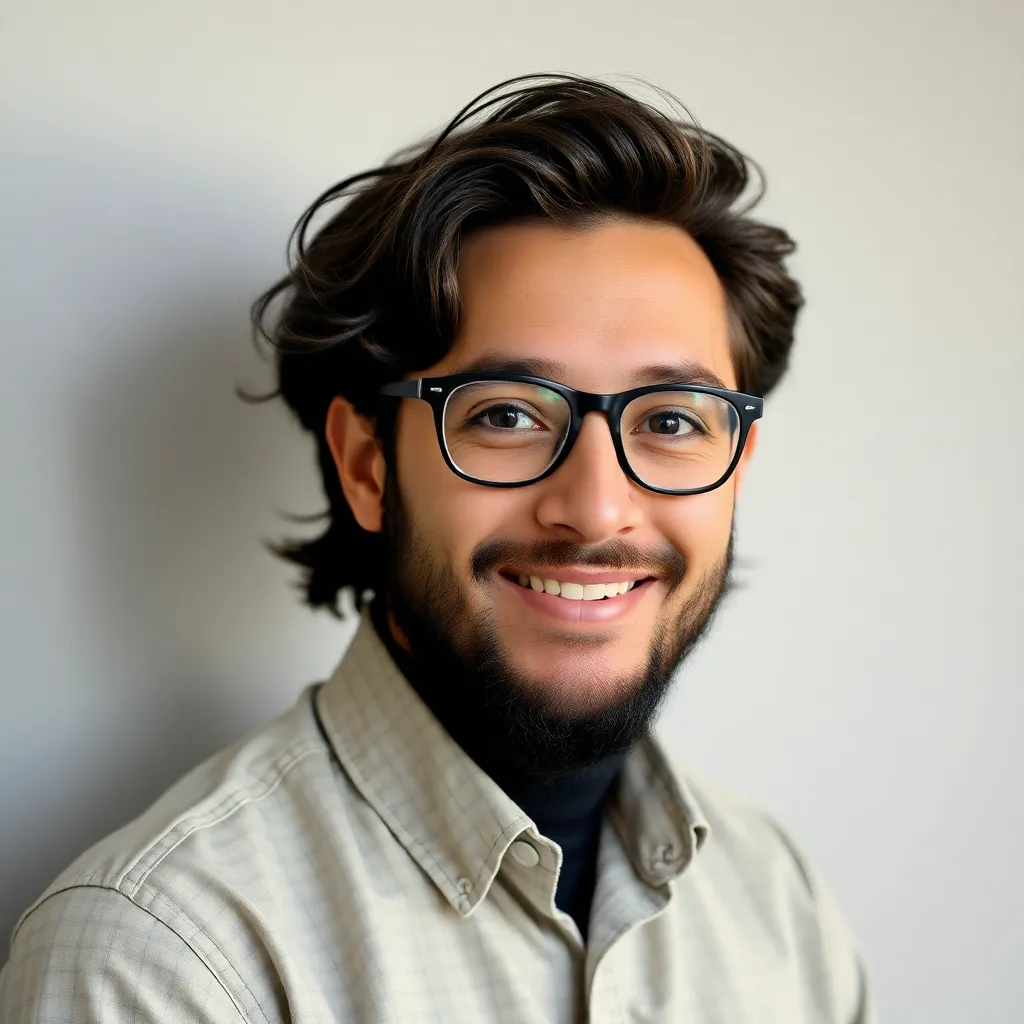
Breaking News Today
Apr 21, 2025 · 5 min read

Table of Contents
Accuracy in Forecasting: Methods of Measurement and Improvement
Forecasting, the process of predicting future outcomes based on available data, is crucial across numerous fields. From predicting stock prices to anticipating weather patterns, accurate forecasting is paramount for informed decision-making. But how do we determine the accuracy of a forecast? This is a critical question, as the value of a forecast is directly tied to its reliability. This article will delve into the various methods used to measure forecasting accuracy and discuss strategies for improving the precision of your predictive models.
Key Metrics for Evaluating Forecast Accuracy
Several quantitative metrics exist to assess the accuracy of a forecast. The choice of metric depends largely on the nature of the data (e.g., continuous, discrete) and the specific goals of the forecasting exercise. Let's explore some of the most commonly used methods:
1. Mean Absolute Deviation (MAD)
MAD measures the average absolute difference between the forecasted and actual values. It's a simple and intuitive metric, easy to calculate and interpret. A lower MAD indicates higher forecast accuracy.
Formula:
MAD = (Σ|Actualᵢ - Forecastᵢ|) / n
where:
- Actualᵢ = the actual value at time i
- Forecastᵢ = the forecasted value at time i
- n = the number of time periods
Advantages:
- Simple to understand and calculate.
- Relatively insensitive to outliers.
Disadvantages:
- Doesn't penalize large errors as heavily as some other metrics.
2. Mean Squared Error (MSE)
MSE calculates the average of the squared differences between the forecasted and actual values. Squaring the errors emphasizes larger errors, making MSE more sensitive to outliers than MAD. A lower MSE suggests better accuracy.
Formula:
MSE = (Σ(Actualᵢ - Forecastᵢ)²) / n
Advantages:
- More sensitive to large errors than MAD.
- Widely used and well-understood.
Disadvantages:
- Can be heavily influenced by outliers.
- The units are squared, making interpretation less intuitive than MAD.
3. Root Mean Squared Error (RMSE)
RMSE is the square root of MSE. Taking the square root returns the error to the original units of the data, making it easier to interpret than MSE. Like MSE, a lower RMSE indicates better accuracy.
Formula:
RMSE = √[(Σ(Actualᵢ - Forecastᵢ)²) / n]
Advantages:
- Interpretable in the original units of the data.
- More sensitive to large errors than MAD.
Disadvantages:
- Still susceptible to the influence of outliers.
4. Mean Absolute Percentage Error (MAPE)
MAPE expresses the average absolute percentage difference between the forecasted and actual values. This metric is particularly useful when comparing forecasts across different datasets with varying scales. A lower MAPE signifies higher accuracy.
Formula:
MAPE = (Σ|Actualᵢ - Forecastᵢ| / Actualᵢ) / n * 100%
Advantages:
- Expresses error as a percentage, facilitating comparison across datasets with different scales.
- Easy to interpret.
Disadvantages:
- Undefined when the actual value is zero.
- Can be misleading when dealing with small actual values.
5. Symmetric Mean Absolute Percentage Error (sMAPE)
sMAPE addresses the undefined nature of MAPE when actual values are zero. It provides a more robust measure when dealing with data containing zero values.
Formula:
sMAPE = (1/n) * Σ [2 * |Forecastᵢ - Actualᵢ| / (|Forecastᵢ| + |Actualᵢ|)] * 100%
Advantages:
- Handles zero values gracefully.
- More robust than MAPE in some cases.
Disadvantages:
- Can still be sensitive to small values.
Choosing the Right Metric
The optimal metric for evaluating forecast accuracy is context-dependent. Consider the following factors:
- Data distribution: If your data has outliers, MAD or RMSE might be preferable to MSE.
- Scale of the data: For comparing forecasts across datasets with different scales, MAPE or sMAPE are suitable choices.
- Business objectives: The metric should align with the specific goals of the forecasting exercise. For instance, minimizing financial risk might necessitate a focus on RMSE.
Beyond Single Metrics: A Holistic Approach
While individual metrics provide valuable insights, relying solely on one can be misleading. A comprehensive evaluation requires considering multiple metrics in conjunction with qualitative assessments. Visualizing the forecast errors using techniques like residual plots can provide further insights into the model's performance and identify potential areas for improvement.
Improving Forecast Accuracy: Strategies and Techniques
Improving forecast accuracy is an iterative process that involves refining both the data and the forecasting model. Here are some key strategies:
1. Data Quality and Preprocessing:
- Data Cleaning: Identify and handle missing values, outliers, and inconsistencies in the data.
- Feature Engineering: Create new variables from existing ones that might improve predictive power. This could involve transformations, aggregations, or interactions.
- Data Transformation: Applying transformations like logarithmic or Box-Cox transformations can stabilize variance and improve model performance.
2. Model Selection and Tuning:
- Explore Multiple Models: Experiment with different forecasting techniques, such as ARIMA, exponential smoothing, machine learning algorithms (e.g., regression, neural networks), to identify the model best suited to your data.
- Hyperparameter Tuning: Optimize the parameters of your chosen model using techniques like cross-validation to maximize its accuracy.
3. Incorporating External Information:
- Economic Indicators: Integrate relevant economic indicators to enhance the model's ability to capture broader trends.
- Seasonality and Trend Analysis: Explicitly model seasonal patterns and trends in the data.
- Qualitative Information: Incorporate expert opinions or qualitative insights to augment quantitative forecasts.
4. Monitoring and Evaluation:
- Regular Monitoring: Continuously monitor the performance of the forecast model and update it as new data becomes available.
- Backtesting: Evaluate the model's performance on historical data to assess its robustness and reliability.
Conclusion
Accurate forecasting is fundamental to informed decision-making in a vast array of fields. Choosing and applying the appropriate accuracy metrics is crucial for objectively evaluating the performance of forecasting models. However, the selection of a suitable metric must be guided by an understanding of the context and objectives of the prediction problem. By using multiple metrics, visualisations, and ongoing monitoring, coupled with careful data preparation and model selection, it’s possible to continually refine forecasting accuracy and enhance the decision-making process. Remember that forecast accuracy is a journey, not a destination – continuous improvement is key to achieving reliable and valuable predictions.
Latest Posts
Latest Posts
-
Raw Shell Eggs Must Be Received In Refrigerated Equipment
Apr 21, 2025
-
Vocabulary Workshop Level E Unit 4 Answers
Apr 21, 2025
-
The Account Type And Normal Balance Of Unearned Revenue Is
Apr 21, 2025
-
Life Insurance Policies Will Normally Pay For Losses Arising From
Apr 21, 2025
-
A Patient Becomes Unresponsive You Are Uncertain
Apr 21, 2025
Related Post
Thank you for visiting our website which covers about Accuracy In Forecasting Can Be Measured By . We hope the information provided has been useful to you. Feel free to contact us if you have any questions or need further assistance. See you next time and don't miss to bookmark.