Agrarian Technology Developed Based On The Use Of
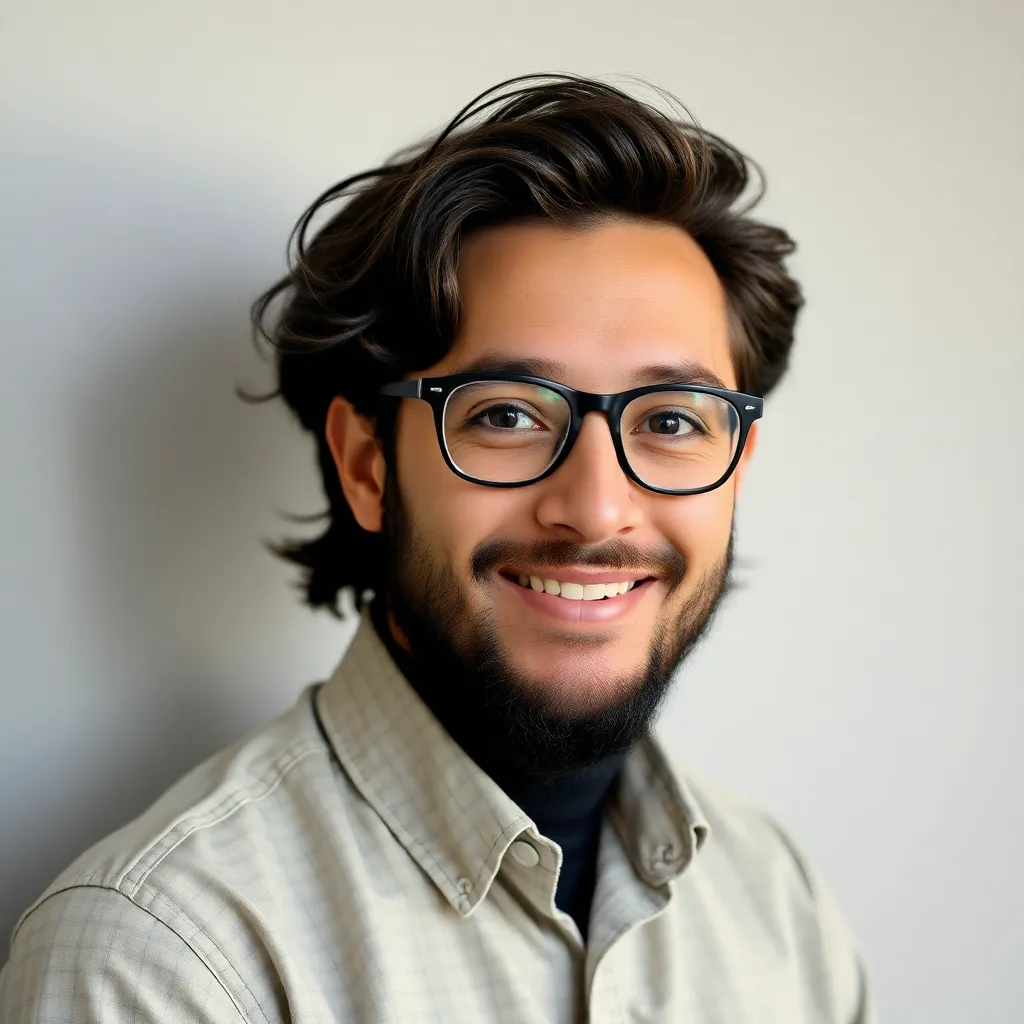
Breaking News Today
May 10, 2025 · 7 min read
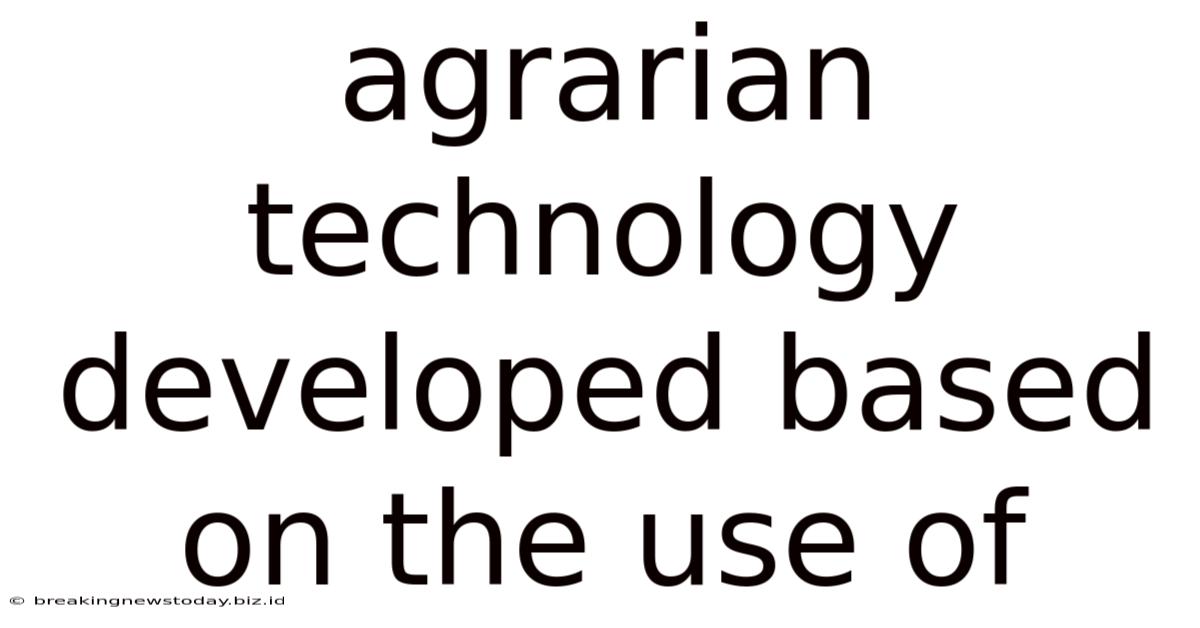
Table of Contents
Agrarian Technology Developed Based on the Use of AI and Machine Learning
The agricultural sector is undergoing a dramatic transformation, driven by advancements in technology. Artificial intelligence (AI) and machine learning (ML) are at the forefront of this revolution, offering innovative solutions to optimize farming practices, increase yields, and ensure sustainability. This article delves into the various agrarian technologies developed based on the use of AI and ML, exploring their applications and the impact they are having on the global food system.
AI and ML in Precision Farming: Optimizing Resource Utilization
Precision farming, a data-driven approach to agriculture, is significantly enhanced by AI and ML. These technologies analyze vast datasets from various sources – sensors, drones, satellites, and farm management systems – to provide farmers with actionable insights. This allows for highly targeted interventions, optimizing the use of resources and maximizing efficiency.
1. Variable Rate Technology (VRT): Tailoring Inputs to Specific Needs
VRT leverages AI and ML to apply inputs such as fertilizers, pesticides, and water at varying rates across a field. Instead of blanket application, which can lead to waste and environmental damage, VRT identifies areas with specific needs based on soil composition, crop health, and other factors. This targeted approach minimizes input costs, reduces environmental impact, and improves crop yields.
Benefits of VRT:
- Reduced Input Costs: By applying only the necessary amount of inputs, farmers significantly reduce expenses.
- Environmental Protection: Minimizes chemical runoff and soil degradation.
- Improved Crop Yields: Optimizing nutrient and water delivery leads to healthier plants and higher yields.
- Enhanced Crop Quality: Targeted application of inputs can improve crop quality characteristics like size, color, and taste.
2. Predictive Analytics: Forecasting Crop Yields and Potential Risks
AI and ML algorithms can analyze historical data, weather patterns, soil conditions, and pest infestations to predict future crop yields and potential risks. This allows farmers to make informed decisions regarding planting, harvesting, and resource allocation. Early warnings about potential problems like disease outbreaks or drought conditions provide opportunities for timely interventions, mitigating potential losses.
Applications of Predictive Analytics:
- Yield Prediction: Accurate yield forecasts help farmers plan for storage, transportation, and marketing.
- Risk Assessment: Identifying potential risks allows for proactive measures to minimize losses.
- Optimized Resource Allocation: Predictive models can guide decisions on water management, fertilization, and pest control.
- Improved Decision Making: Data-driven insights empower farmers to make more informed and efficient decisions.
AI-Powered Robotics and Automation: Increasing Efficiency and Reducing Labor Costs
The integration of AI and robotics is revolutionizing agricultural practices, automating tasks that were previously labor-intensive and time-consuming. AI-powered robots can perform various tasks with greater efficiency and precision than human workers.
1. Autonomous Tractors and Sprayers: Precision and Efficiency in Field Operations
Self-driving tractors and sprayers equipped with AI-powered navigation systems can operate autonomously, performing tasks such as planting, spraying, and harvesting with remarkable precision. These machines use GPS, sensors, and computer vision to navigate fields, avoid obstacles, and optimize their operations.
Advantages of Autonomous Machinery:
- Increased Efficiency: Reduces labor costs and improves operational speed.
- Improved Precision: Enhances accuracy in applying inputs and performing other field operations.
- Reduced Labor Shortages: Addresses the growing challenge of finding and retaining agricultural labor.
- 24/7 Operation: Autonomous machines can operate around the clock, maximizing productivity.
2. Robotic Harvesting: Addressing Labor Shortages and Improving Efficiency
Robotic harvesting systems are being developed for various crops, offering solutions to labor shortages and improving the efficiency of harvesting processes. These systems use computer vision, AI, and robotics to identify ripe fruits and vegetables, carefully picking them without damaging the plant.
Benefits of Robotic Harvesting:
- Reduced Labor Costs: Automated harvesting reduces reliance on manual labor.
- Improved Efficiency: Robots can work faster and more consistently than human workers.
- Reduced Waste: Minimizes damage to crops during harvesting.
- Improved Quality: Careful handling by robots can enhance the quality of harvested produce.
AI in Crop Monitoring and Disease Detection: Early Intervention and Prevention
AI and ML algorithms are proving invaluable in monitoring crop health and detecting diseases at early stages. This enables timely interventions, preventing widespread outbreaks and minimizing crop losses.
1. Computer Vision for Disease and Pest Detection: Early Warning Systems
Computer vision systems, powered by AI, can analyze images and videos captured by drones or ground-based sensors to identify signs of diseases and pests. This allows for early detection, enabling farmers to take prompt action, such as targeted pesticide application or other remedial measures.
Advantages of Computer Vision:
- Early Disease Detection: Enables timely intervention to prevent widespread outbreaks.
- Improved Accuracy: AI algorithms can detect diseases at early stages, often before they are visible to the naked eye.
- Reduced Pesticide Use: Early detection allows for targeted application of pesticides, minimizing environmental impact.
- Enhanced Monitoring Capabilities: Provides comprehensive monitoring of large areas.
2. AI-Powered Diagnostics: Accurate Identification and Treatment Recommendations
AI algorithms can analyze data from various sources – images, sensor readings, and historical data – to diagnose crop diseases and provide treatment recommendations. This speeds up the diagnostic process and reduces the risk of misdiagnosis, leading to more effective treatment and reduced crop losses.
Benefits of AI-Powered Diagnostics:
- Faster Diagnosis: Speeds up the diagnostic process, leading to quicker treatment.
- Improved Accuracy: Reduces the risk of misdiagnosis, ensuring effective treatment.
- Personalized Treatment Recommendations: Provides tailored treatment plans based on specific crop needs.
- Reduced Crop Losses: Early and accurate diagnosis minimizes crop damage and yield losses.
AI and ML for Sustainable Agriculture: Optimizing Resource Use and Environmental Impact
AI and ML are instrumental in promoting sustainable agriculture practices, minimizing the environmental footprint of farming operations.
1. Precision Irrigation: Optimizing Water Use and Reducing Water Stress
AI-powered irrigation systems use sensors, weather data, and soil moisture information to optimize water usage. This reduces water waste, minimizes water stress on crops, and contributes to water conservation efforts.
Benefits of Precision Irrigation:
- Reduced Water Consumption: Minimizes water waste and improves water use efficiency.
- Improved Crop Yields: Ensures adequate water supply to plants without overwatering.
- Reduced Environmental Impact: Contributes to water conservation and reduces water pollution.
- Cost Savings: Reduces water bills and minimizes the need for extensive irrigation systems.
2. AI-Driven Weed Management: Reducing Herbicide Use and Promoting Biodiversity
AI-powered weed management systems use computer vision to identify and target weeds, allowing for precise weed control without the need for blanket herbicide application. This reduces herbicide use, minimizes environmental impact, and promotes biodiversity.
Advantages of AI-Driven Weed Management:
- Reduced Herbicide Use: Minimizes the use of harmful chemicals.
- Improved Environmental Sustainability: Reduces the impact of herbicides on the environment.
- Enhanced Biodiversity: Preserves beneficial insects and other organisms.
- Cost Savings: Reduces the costs associated with herbicide application.
The Future of AI in Agriculture: Emerging Trends and Challenges
The application of AI and ML in agriculture is constantly evolving, with new technologies and approaches emerging at a rapid pace. Some of the key future trends include:
- Increased use of IoT devices: The integration of more sophisticated sensors and IoT devices will provide richer datasets for AI algorithms.
- Advanced data analytics: Sophisticated AI algorithms will be able to analyze complex datasets to provide more accurate predictions and insights.
- Development of more specialized AI models: AI models will be developed for specific crops, regions, and farming practices.
- Greater integration of AI with other technologies: AI will be integrated with other technologies like robotics, drones, and blockchain to create more comprehensive solutions.
However, challenges remain:
- Data availability and quality: The effectiveness of AI depends on the availability of high-quality data. Data scarcity, especially in developing countries, poses a significant challenge.
- Computational resources: Training and deploying sophisticated AI models require significant computational resources.
- Accessibility and affordability: Making AI-powered solutions accessible and affordable to farmers, particularly smallholder farmers, is crucial.
- Ethical considerations: Addressing ethical concerns related to data privacy, algorithmic bias, and job displacement is crucial.
Conclusion: AI-Driven Transformation of the Agricultural Sector
AI and ML are driving a significant transformation in the agricultural sector, offering innovative solutions to improve productivity, sustainability, and efficiency. These technologies are empowering farmers to make more informed decisions, optimize resource utilization, and enhance their overall operations. While challenges remain, the future of agriculture is undoubtedly intertwined with the continued development and adoption of AI-powered technologies. By addressing the challenges and harnessing the potential of AI, we can ensure a more sustainable, efficient, and resilient global food system for the future.
Latest Posts
Latest Posts
-
Paper Based Field Service Records Were Replaced With What Database
May 11, 2025
-
You Have A Classified Document You Would Like To Share
May 11, 2025
-
Population Growth And Ecological Data Answer Key
May 11, 2025
-
Service Learning Programs For Teens Result In
May 11, 2025
-
Which Choice Is The Best Definition Of A Performance Appraisal
May 11, 2025
Related Post
Thank you for visiting our website which covers about Agrarian Technology Developed Based On The Use Of . We hope the information provided has been useful to you. Feel free to contact us if you have any questions or need further assistance. See you next time and don't miss to bookmark.