Ap Stats Unit 5 Progress Check Mcq Part B
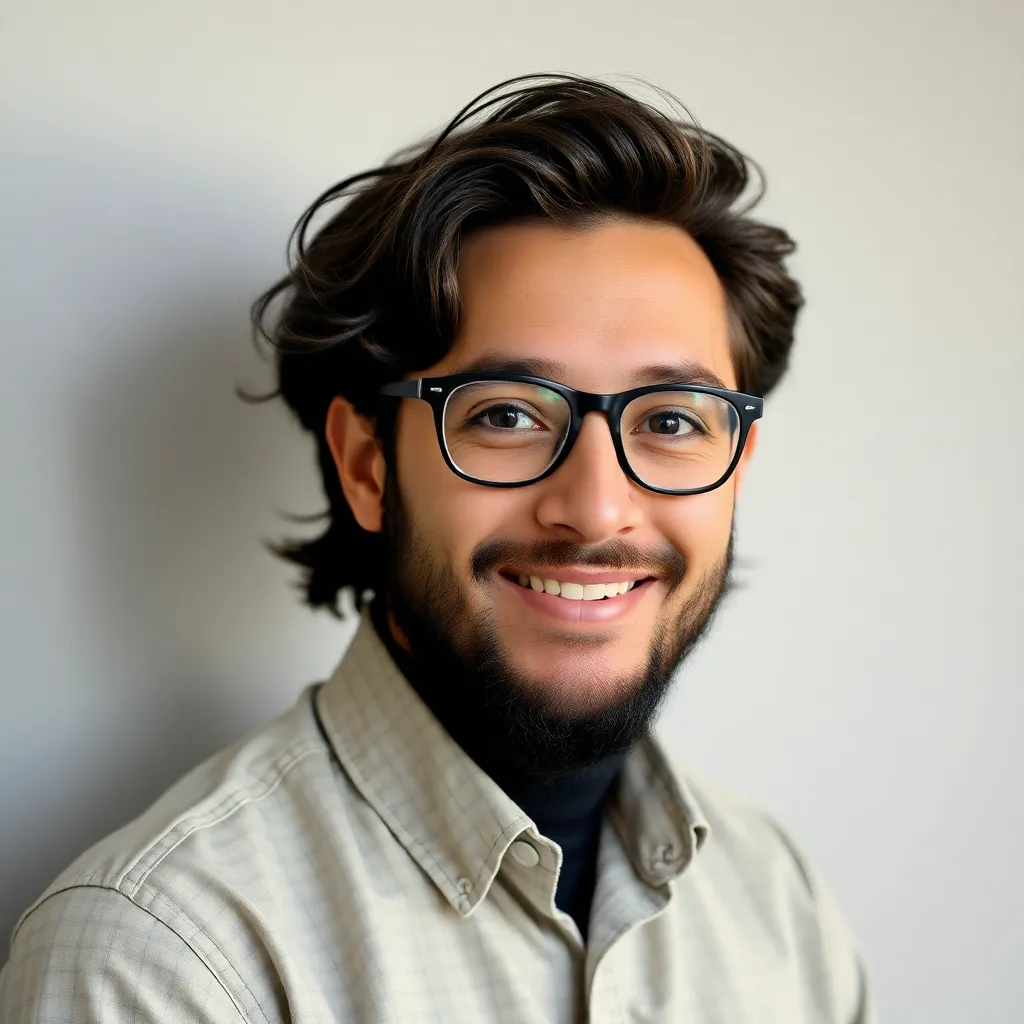
Breaking News Today
Apr 16, 2025 · 6 min read

Table of Contents
AP Stats Unit 5 Progress Check: MCQ Part B – A Deep Dive
Unit 5 of AP Statistics, focusing on sampling distributions, is a crucial component of the course. The Progress Check MCQs, particularly Part B, often challenge students with nuanced questions requiring a solid understanding of concepts like sampling variability, the Central Limit Theorem (CLT), and confidence intervals. This comprehensive guide will dissect the key concepts tested in this section, providing explanations, examples, and strategies to master these challenging questions.
Understanding Sampling Distributions
The foundation of Unit 5 lies in grasping the concept of sampling distributions. A sampling distribution isn't a distribution of a single sample; instead, it's the distribution of a statistic (like the sample mean or sample proportion) calculated from numerous random samples of the same size drawn from a population. Understanding this distinction is paramount.
Key Characteristics of Sampling Distributions
- Center: The center of a sampling distribution of sample means is typically equal to the population mean (µ). For sample proportions, the center is the population proportion (p).
- Spread: The spread, or standard deviation, of a sampling distribution is smaller than the population's standard deviation. This is because averaging multiple samples reduces variability. This standard deviation of the sampling distribution is called the standard error. For means, it's σ/√n; for proportions, it's √(p(1-p)/n), where 'n' is the sample size.
- Shape: The shape of a sampling distribution often approaches a normal distribution, especially as the sample size (n) increases. This is the essence of the Central Limit Theorem (CLT).
The Central Limit Theorem (CLT): A Cornerstone of Inference
The CLT is arguably the most important theorem in inferential statistics. It states that, regardless of the shape of the population distribution, the sampling distribution of the sample mean (x̄) will be approximately normal if the sample size is sufficiently large (generally n ≥ 30). This approximation improves as 'n' increases. For sample proportions, the CLT applies when np ≥ 10 and n(1-p) ≥ 10.
Implications of the CLT
- Confidence Intervals: The CLT justifies the use of normal distributions to construct confidence intervals for population means and proportions. We can be confident that a certain percentage of our intervals will contain the true population parameter.
- Hypothesis Testing: The CLT underpins many hypothesis tests, allowing us to determine if sample data provides enough evidence to reject a null hypothesis about a population parameter.
Tackling MCQ Part B: Common Question Types
Part B of the Unit 5 Progress Check often presents scenarios that test your understanding of the above concepts in various ways. Here are some common question types and strategies to address them:
1. Calculating Standard Error
Many questions require calculating the standard error for the sample mean or sample proportion. Ensure you understand the formulas and when to use each one. Pay close attention to whether the question provides the population standard deviation (σ) or the sample standard deviation (s). Using 's' instead of 'σ' when calculating the standard error is a common mistake.
Example: A random sample of 100 light bulbs has a mean lifespan of 800 hours with a standard deviation of 50 hours. What is the standard error of the sample mean?
Solution: Since we have the sample standard deviation (s = 50), we use the formula s/√n = 50/√100 = 5 hours.
2. Interpreting Sampling Distributions
Questions may present graphs or descriptions of sampling distributions and ask you to interpret them. This could involve identifying the center, spread, or shape of the distribution and relating it to the population parameter and sample size. Be prepared to analyze graphical representations of sampling distributions.
Example: A graph shows the sampling distribution of sample means for samples of size 50. The distribution is approximately normal with a mean of 100 and a standard deviation of 5. What can be inferred about the population mean?
Solution: Based on the CLT, the mean of the sampling distribution (100) is a good estimate of the population mean.
3. Applying the Central Limit Theorem
Many questions test your understanding of when the CLT applies and its implications. Remember that the CLT doesn't require the population to be normally distributed; the sampling distribution becomes approximately normal for large sample sizes.
Example: A population is highly skewed. Can we still use the CLT to construct a confidence interval for the population mean if we have a sample size of 100?
Solution: Yes, the CLT states that for large sample sizes (n ≥ 30), the sampling distribution of the sample mean will be approximately normal, regardless of the population's shape.
4. Confidence Intervals and Margin of Error
Understanding confidence intervals is crucial. Questions might ask you to calculate a confidence interval or interpret the meaning of a given confidence interval. Remember that a confidence interval provides a range of plausible values for the population parameter, and the margin of error reflects the uncertainty in this estimate.
Example: A 95% confidence interval for the population mean is (50, 70). What does this mean?
Solution: We are 95% confident that the true population mean lies between 50 and 70.
5. Hypothesis Testing within the Context of Sampling Distributions
Some questions might subtly introduce elements of hypothesis testing, relating it to the properties of sampling distributions. Understanding the null hypothesis, alternative hypothesis, p-values, and significance levels is vital here. Remember that a small p-value provides evidence against the null hypothesis.
Example: A researcher wants to test if the population mean is different from 100. They take a sample and calculate a sample mean significantly different from 100. How does this relate to the sampling distribution?
Solution: If the sample mean is significantly different from 100, it falls far from the center of the sampling distribution (assuming the null hypothesis is true), indicating a small p-value and suggesting we reject the null hypothesis.
Advanced Concepts and Nuances
Some questions in Part B might delve into more advanced topics:
1. Sampling Variability
This concept emphasizes the inherent variation between different samples from the same population. Understanding that different samples will yield different statistics is crucial for interpreting sampling distributions.
2. Finite Population Correction Factor
When sampling without replacement from a small finite population, a finite population correction factor is applied to adjust the standard error. Be aware of when this adjustment is necessary.
3. Stratified Random Sampling vs. Simple Random Sampling
Understanding the differences between these sampling methods and their impact on the precision of estimates is important.
Strategies for Success
- Practice, Practice, Practice: Work through numerous practice problems, focusing on understanding the underlying concepts rather than memorizing formulas. Utilize past AP Statistics exams and practice materials.
- Master the Formulas: Know the formulas for standard error for means and proportions.
- Visualize: Draw diagrams and visualize the sampling distributions. This can greatly aid understanding.
- Understand the Context: Pay close attention to the wording of the problem. Understanding the context is crucial for correct interpretation.
- Review the CLT thoroughly: The CLT is the backbone of many questions. Ensure you deeply understand its implications.
By carefully studying these concepts, practicing diligently, and mastering the associated formulas, you can confidently approach and successfully answer the challenging MCQs in AP Stats Unit 5 Progress Check Part B. Remember that understanding the underlying principles is more valuable than rote memorization. Good luck!
Latest Posts
Latest Posts
-
Mandy Will Need To Extend Her Ladder To 60 Feet
May 09, 2025
-
Southern And Eastern Asia Physical Features Map
May 09, 2025
-
Which Is A Result Of Island Hopping
May 09, 2025
-
The Core Element Of Every Play Is
May 09, 2025
-
During The Meuse Argonne Offensive Of 1918 The Americans Helped
May 09, 2025
Related Post
Thank you for visiting our website which covers about Ap Stats Unit 5 Progress Check Mcq Part B . We hope the information provided has been useful to you. Feel free to contact us if you have any questions or need further assistance. See you next time and don't miss to bookmark.