Ap Stats Unit 7 Progress Check Mcq Part B
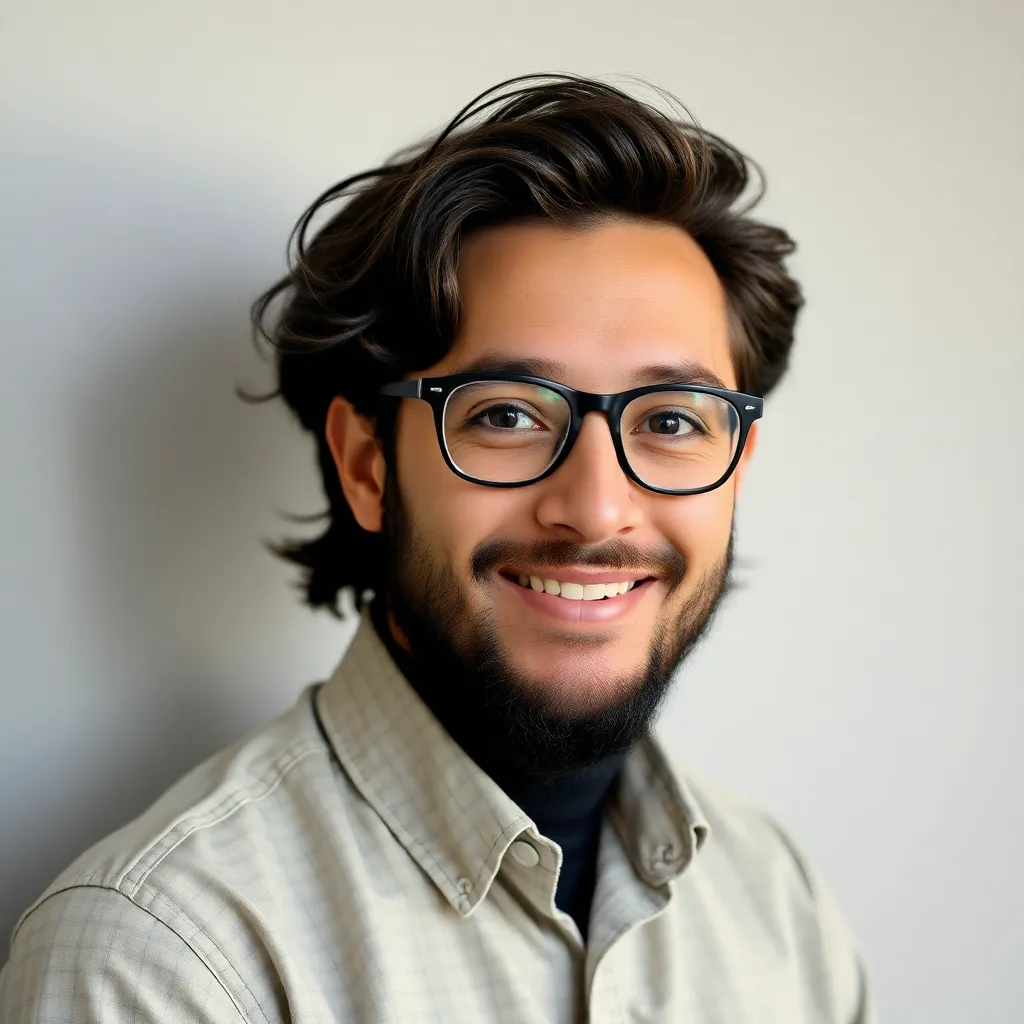
Breaking News Today
May 11, 2025 · 7 min read
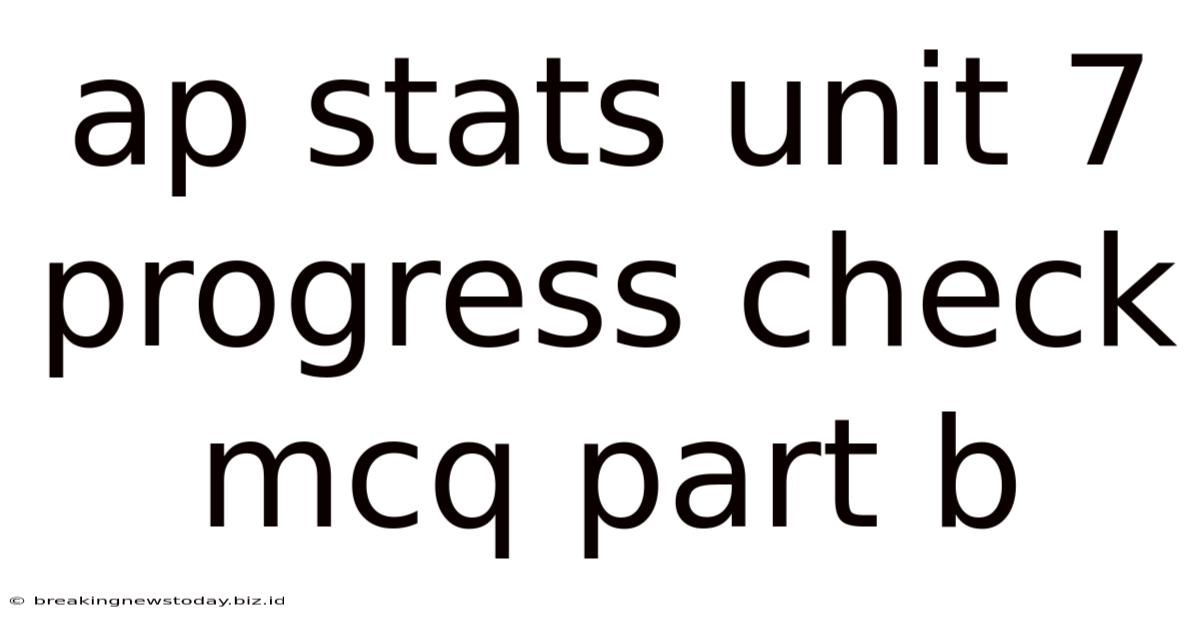
Table of Contents
AP Stats Unit 7 Progress Check: MCQ Part B – A Deep Dive
Unit 7 of AP Statistics delves into the fascinating world of inference for categorical data. Progress Check MCQ Part B tests your understanding of concepts like chi-square tests, goodness-of-fit tests, and tests of homogeneity and independence. This comprehensive guide will break down the key concepts, provide example problems, and offer strategies for tackling these challenging multiple-choice questions.
Understanding the Big Picture: Inference for Categorical Data
Before diving into the specifics of Part B, let's solidify our understanding of the core concepts in Unit 7. We're moving beyond numerical data and focusing on categorical data – data that can be divided into categories or groups. The key inferential procedures used here revolve around the chi-square distribution.
Key Concepts to Master:
-
Chi-Square Distribution: This probability distribution is used to analyze categorical data. It's characterized by its right-skew and dependence on degrees of freedom (df). Understanding its properties is crucial for interpreting chi-square tests.
-
Expected Counts: These are the counts we expect to observe in each category under the assumption of the null hypothesis. Calculating these accurately is vital for the chi-square test statistic.
-
Observed Counts: These are the actual counts observed in the data. Comparing these to the expected counts is the foundation of the chi-square test.
-
Chi-Square Test Statistic: This statistic measures the difference between the observed and expected counts. A larger chi-square value indicates a greater discrepancy between the observed and expected, suggesting evidence against the null hypothesis.
-
Degrees of Freedom (df): The degrees of freedom depend on the structure of the data (e.g., number of categories, number of rows and columns in a contingency table). It influences the shape of the chi-square distribution.
-
P-value: The probability of observing a chi-square statistic as extreme as (or more extreme than) the one calculated, assuming the null hypothesis is true. A small p-value (typically less than 0.05) provides evidence against the null hypothesis.
-
Goodness-of-Fit Test: Used to determine if a sample distribution matches a hypothesized distribution.
-
Test of Homogeneity: Used to compare the distributions of a categorical variable across different populations.
-
Test of Independence: Used to determine if two categorical variables are independent of each other.
Deconstructing the MCQ Part B Questions:
Part B questions will typically involve analyzing contingency tables, calculating expected counts, interpreting chi-square test results, and understanding the conditions for conducting these tests. Let's look at some common question types and strategies for solving them.
Type 1: Calculating Expected Counts
These questions will often present a contingency table with observed counts and ask you to calculate the expected counts under the assumption of independence (for a test of independence) or homogeneity (for a test of homogeneity).
Example:
A researcher is studying the relationship between gender and preference for coffee or tea. The observed counts are:
Coffee | Tea | Total | |
---|---|---|---|
Male | 40 | 60 | 100 |
Female | 30 | 70 | 100 |
Total | 70 | 130 | 200 |
Calculate the expected count of males who prefer coffee.
Solution:
Under the assumption of independence, the expected count for males who prefer coffee is:
(Row total for males) * (Column total for coffee) / (Grand total) = (100 * 70) / 200 = 35
Strategy: Remember the formula for calculating expected counts: (Row total * Column total) / Grand total. Pay close attention to the specific row and column you are calculating the expected count for.
Type 2: Interpreting Chi-Square Test Results
These questions will typically give you a chi-square statistic, degrees of freedom, and a p-value and ask you to interpret the results in the context of the problem.
Example:
A chi-square test of independence yields a chi-square statistic of 10.5, with 1 degree of freedom and a p-value of 0.001. What conclusion can you draw?
Solution:
Since the p-value (0.001) is less than the significance level (typically 0.05), we reject the null hypothesis. There is strong evidence to suggest that the two categorical variables are not independent.
Strategy: Always relate your conclusions back to the context of the problem. Don't just state whether you reject or fail to reject the null hypothesis; explain what that means in terms of the variables being studied.
Type 3: Identifying Conditions for Inference
Before performing a chi-square test, it's crucial to check certain conditions. These questions may test your understanding of these conditions.
Example:
Which of the following is NOT a condition for conducting a chi-square test of independence?
a) All expected counts are at least 5. b) The sample is a random sample. c) The variables are categorical. d) The sample size is large.
Solution:
While a large sample size is generally desirable, it's not a strict condition. The crucial condition is that all expected counts are at least 5. Therefore, the answer is (d).
Strategy: Memorize the conditions: random sample, all expected counts ≥ 5, and categorical variables. Be prepared to identify situations where these conditions are violated.
Type 4: Goodness-of-Fit Test Scenarios
These questions will involve scenarios where you are testing whether observed data fits a particular distribution (e.g., uniform, binomial).
Example:
A die is rolled 60 times. The observed counts for each face are: 1 (8), 2 (12), 3 (9), 4 (11), 5 (10), 6 (10). Would a goodness-of-fit test indicate the die is fair?
Solution: You would calculate the expected counts for each face (10 in this case, assuming a fair die). Then conduct a chi-square goodness-of-fit test. A small p-value would suggest the die is not fair.
Strategy: For these scenarios, clearly define your null and alternative hypotheses. Remember to calculate the expected counts under the null hypothesis (e.g., equal probabilities for each face of a fair die).
Type 5: Homogeneity vs. Independence
Distinguishing between tests of homogeneity and tests of independence can be tricky. Questions might ask you to identify the appropriate test given a scenario.
Example:
A researcher compares the proportions of smokers and non-smokers among men and women. Which chi-square test is appropriate?
Solution: A test of homogeneity is appropriate because we are comparing the distributions of one categorical variable (smoker/non-smoker) across different populations (men and women).
Strategy: Remember: Homogeneity compares distributions across different groups; Independence explores the relationship between two variables within a single group.
Advanced Strategies and Tips for Success:
-
Practice, Practice, Practice: Work through numerous practice problems to build your understanding and improve your problem-solving skills.
-
Understand the Context: Always relate your statistical findings back to the context of the problem. Don't just state the statistical results; interpret them in the specific situation.
-
Use Technology: Statistical software or calculators can greatly assist with the calculations. Understanding how to use these tools efficiently can save you time and reduce errors.
-
Review the Formulas: Make sure you are comfortable with the formula for calculating expected counts and understand how the chi-square statistic is calculated.
-
Visualize the Data: Creating a contingency table or other visual representation of the data can help you understand the relationships between variables.
-
Focus on Interpretation: A significant portion of the MCQ Part B will focus on your ability to interpret the results of the chi-square test and draw appropriate conclusions.
By mastering these concepts and practicing extensively, you'll significantly improve your chances of succeeding on the AP Stats Unit 7 Progress Check MCQ Part B. Remember that consistent effort and a strong understanding of the underlying principles are key to success. Good luck!
Latest Posts
Latest Posts
-
What Is The Definition Of Milk Anemia
May 12, 2025
-
Which Army Wide Policy Governs Records Management
May 12, 2025
-
3 05 Quiz A Midsummer Nights Dream C
May 12, 2025
-
It Is A Requirement Under Hipaa That
May 12, 2025
-
Money And The Federal Reserve Mastery Test
May 12, 2025
Related Post
Thank you for visiting our website which covers about Ap Stats Unit 7 Progress Check Mcq Part B . We hope the information provided has been useful to you. Feel free to contact us if you have any questions or need further assistance. See you next time and don't miss to bookmark.