Based On A Survey Of A Random Sample Of 900
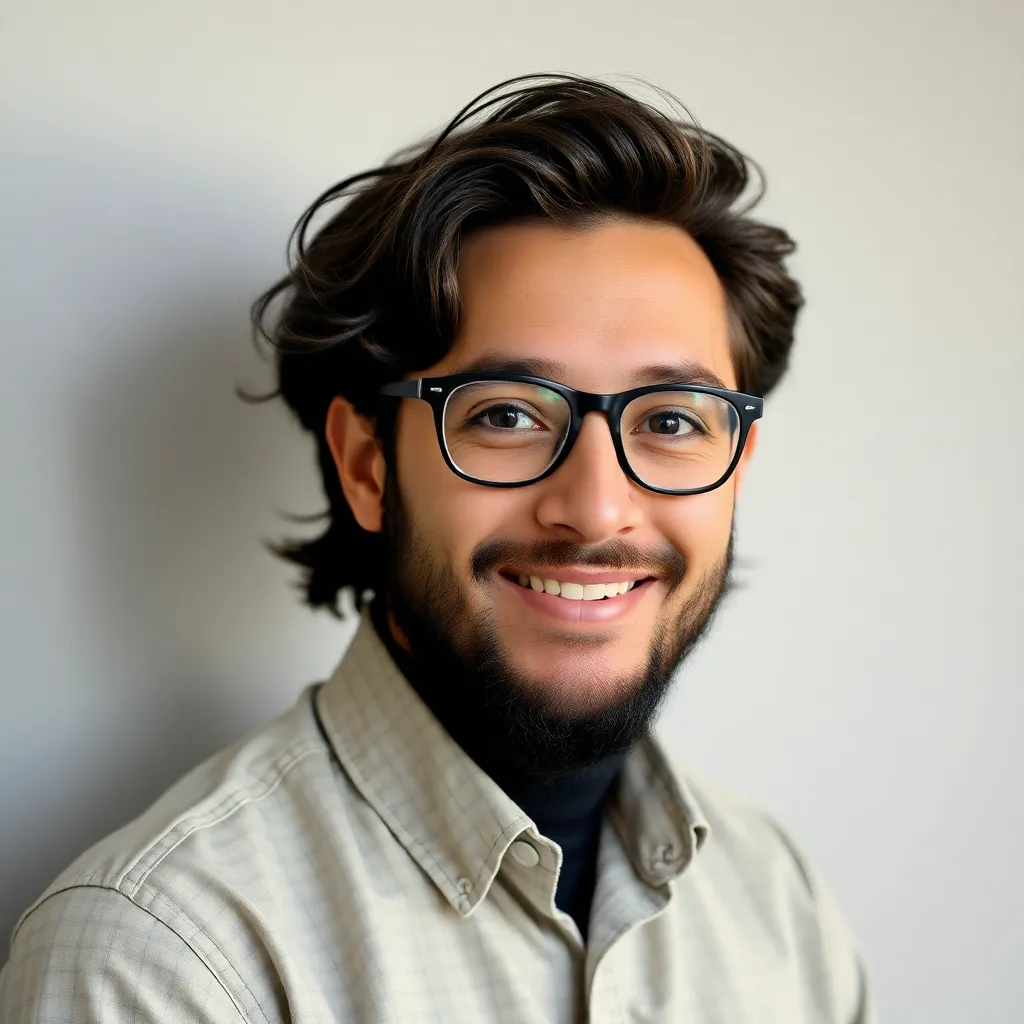
Breaking News Today
Apr 09, 2025 · 8 min read

Table of Contents
Unlocking Insights: Analyzing Survey Data from a Random Sample of 900
Analyzing survey data from a random sample of 900 participants offers a powerful opportunity to glean valuable insights into a population's characteristics, behaviors, and opinions. This article delves into the process of analyzing such data, highlighting key statistical techniques and considerations to ensure accurate and meaningful results. We'll explore everything from data cleaning and preparation to hypothesis testing and drawing reliable conclusions.
1. The Importance of a Random Sample
Before diving into the analysis, it's crucial to understand the significance of using a random sample. A random sample ensures that each member of the population has an equal chance of being selected. This minimizes sampling bias, a crucial factor in obtaining results that accurately represent the broader population. Without a random sample, any conclusions drawn from the survey might be skewed and unreliable, making the entire analysis process largely ineffective. The size of the sample (900 in this case) also contributes to the reliability of the results, reducing the margin of error.
2. Data Cleaning and Preparation: Laying the Foundation
Raw survey data is rarely perfect. Before any analysis can commence, a thorough cleaning and preparation phase is essential. This involves several critical steps:
-
Identifying and Handling Missing Data: Missing responses are common in surveys. Strategies for dealing with missing data include imputation (estimating missing values based on available data), exclusion (removing participants with missing data), or using statistical methods designed for handling incomplete data sets. The chosen method should be justified and documented.
-
Data Validation: This step involves checking for inconsistencies, errors, and outliers in the data. For example, age values that are negative or impossibly high need to be reviewed and corrected or removed. Similarly, inconsistent responses across different questions might indicate data entry errors.
-
Data Transformation: Raw data often requires transformation to make it suitable for analysis. This might involve recoding categorical variables (e.g., converting "yes/no" responses into 1/0), creating new variables by combining existing ones, or standardizing numerical variables (e.g., using z-scores).
-
Data Coding: Assign numerical values to categorical responses to allow for efficient statistical analysis. For example, "Strongly Agree," "Agree," "Neutral," "Disagree," and "Strongly Disagree" could be coded as 5, 4, 3, 2, and 1 respectively. This allows for calculations of means, standard deviations and other meaningful statistics.
3. Descriptive Statistics: Summarizing the Data
Once the data is clean and prepared, descriptive statistics are used to summarize the key features of the dataset. This provides a foundational understanding of the data before moving on to more complex analyses. Key descriptive statistics include:
-
Measures of Central Tendency: These describe the "typical" value in the dataset. This includes the mean (average), median (middle value), and mode (most frequent value). The choice of measure depends on the distribution of the data. For example, the median is less sensitive to outliers than the mean.
-
Measures of Dispersion: These describe the spread or variability of the data. Key measures include the range (difference between the highest and lowest values), variance (average squared deviation from the mean), and standard deviation (square root of the variance). A larger standard deviation suggests greater variability.
-
Frequency Distributions: These show the number of times each value or category occurs in the dataset. Frequency distributions are particularly useful for categorical data, providing insights into the prevalence of different responses. Visual representations like bar charts and pie charts can effectively illustrate frequency distributions.
-
Cross-tabulations: These examine the relationship between two or more categorical variables. They show the frequency of each combination of categories, allowing for the identification of potential associations.
4. Inferential Statistics: Drawing Conclusions about the Population
Descriptive statistics summarize the sample data. However, the ultimate goal is often to draw inferences about the larger population from which the sample was drawn. This requires the use of inferential statistics. Key inferential techniques include:
-
Hypothesis Testing: This involves formulating a hypothesis about the population (e.g., "The average age of respondents is 35") and testing it using the sample data. Statistical tests (such as t-tests, ANOVA, chi-square tests) determine the probability of observing the sample results if the hypothesis were true. If the probability is low (typically below a significance level of 0.05), the hypothesis is rejected.
-
Confidence Intervals: These provide a range of values within which the true population parameter (e.g., the population mean) is likely to fall with a certain level of confidence (e.g., 95%). A narrower confidence interval indicates greater precision in the estimate.
-
Regression Analysis: This technique examines the relationship between a dependent variable and one or more independent variables. It allows for the prediction of the dependent variable based on the values of the independent variables. Linear regression is a common type used when the relationship is linear, while other regression models handle more complex relationships.
-
Correlation Analysis: This explores the strength and direction of the linear relationship between two continuous variables. The correlation coefficient (r) ranges from -1 to +1, with values closer to -1 indicating a strong negative relationship, values closer to +1 indicating a strong positive relationship, and a value of 0 indicating no linear relationship.
5. Choosing the Right Statistical Tests
The choice of statistical test depends on several factors, including:
-
The type of data: Different tests are appropriate for categorical (nominal or ordinal) and continuous (interval or ratio) data.
-
The number of groups being compared: Tests like t-tests compare two groups, while ANOVA compares three or more groups.
-
The research question: The specific question being asked will dictate the most appropriate statistical approach.
-
Assumptions of the Test: Many statistical tests make assumptions about the data, such as normality (data follows a normal distribution). Violations of these assumptions can affect the validity of the results. Non-parametric tests are available for data that doesn't meet the assumptions of parametric tests.
6. Visualizing the Results: Communicating Insights Effectively
Effective data visualization is crucial for communicating the findings of the survey analysis to a wider audience. Different types of charts and graphs are suitable for different types of data and research questions:
-
Bar charts: Excellent for displaying frequencies or proportions of categorical data.
-
Pie charts: Useful for showing the proportion of each category within a whole.
-
Histograms: Show the distribution of continuous data.
-
Scatter plots: Illustrate the relationship between two continuous variables.
-
Line graphs: Useful for showing trends over time or across different categories.
7. Reporting the Findings: Clarity and Transparency
The final step involves presenting the findings in a clear, concise, and transparent manner. A well-written report should include:
-
Introduction: Clearly states the purpose of the survey and the research questions being addressed.
-
Methodology: Describes the sampling method, data collection procedures, and data analysis techniques used.
-
Results: Presents the key findings using both descriptive statistics and inferential statistics. Visualizations should be included to enhance understanding.
-
Discussion: Interprets the findings in relation to the research questions and existing literature. Limitations of the study should also be acknowledged.
-
Conclusion: Summarizes the main conclusions and implications of the study.
8. Addressing Potential Biases and Limitations
Even with a random sample, biases can still arise. It's crucial to acknowledge and address potential limitations:
-
Sampling Bias: While random sampling minimizes bias, it doesn't eliminate it entirely. Factors such as non-response bias (some individuals may not respond to the survey) can still affect the results.
-
Response Bias: Respondents might answer questions in a way that they perceive as socially desirable or that aligns with what they believe the researcher wants to hear.
-
Question Bias: Poorly worded questions can lead to biased responses. Carefully crafted and pre-tested questions are essential for minimizing bias.
-
Measurement Bias: The way variables are measured can influence the results.
Addressing these potential biases requires careful consideration of the survey design, data collection methods, and analysis techniques. Transparency about limitations is crucial for maintaining the credibility of the findings.
9. Advanced Analytical Techniques
For more complex research questions, advanced analytical techniques may be necessary:
-
Factor Analysis: This statistical method is used to identify underlying latent variables that explain the correlations among a set of observed variables. It's particularly useful for reducing the dimensionality of large datasets.
-
Cluster Analysis: Groups similar observations together based on their characteristics. This technique can be used to identify distinct segments within the population.
-
Multilevel Modeling: Analyzes data with hierarchical structures, such as students nested within schools or employees nested within departments.
-
Structural Equation Modeling (SEM): Tests complex relationships between multiple variables, including both direct and indirect effects.
10. Beyond the Numbers: Context and Interpretation
Analyzing survey data is not simply about crunching numbers; it's about understanding the context and interpreting the results meaningfully. The findings should be placed within the broader context of relevant literature and theory. Furthermore, understanding the limitations of the study is crucial for responsible interpretation. Avoid making claims that go beyond the scope of the data.
By following these steps and applying appropriate statistical methods, researchers can effectively analyze survey data from a random sample of 900 participants, drawing meaningful conclusions about the population of interest and contributing valuable insights to the field of study. Remember that the success of the analysis hinges on careful planning, meticulous data handling, and a rigorous approach to statistical inference and interpretation. The detailed explanations and considerations outlined above provide a robust framework for conducting comprehensive and reliable survey data analysis.
Latest Posts
Latest Posts
-
How Does Excess Air Affect The Vent Gas Co2 Percentage
Apr 18, 2025
-
Elena Finds It Very Difficult To Remember A Long String
Apr 18, 2025
-
What Does Mrs D Cheg Stand For
Apr 18, 2025
-
Respiratory Physiology Is Primarily The Study Of
Apr 18, 2025
-
The Human Arterial And Venous Systems Are Diagrammed
Apr 18, 2025
Related Post
Thank you for visiting our website which covers about Based On A Survey Of A Random Sample Of 900 . We hope the information provided has been useful to you. Feel free to contact us if you have any questions or need further assistance. See you next time and don't miss to bookmark.