Circuit Training Inference For Proportions Answer Key
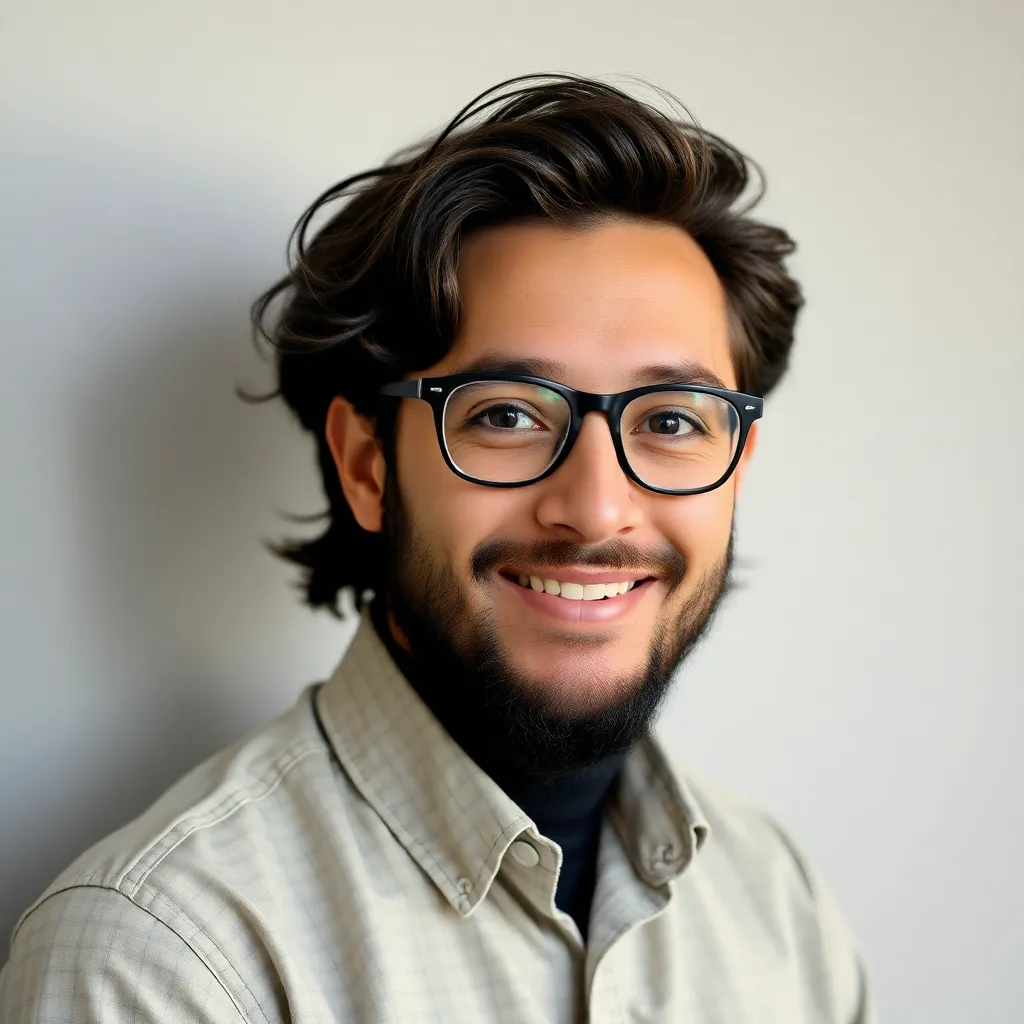
Breaking News Today
Apr 22, 2025 · 7 min read

Table of Contents
Circuit Training Inference for Proportions: Answer Key & Deep Dive
Circuit training, a popular fitness method, can also be a powerful tool for reinforcing learning concepts, particularly in statistics. This article provides a comprehensive guide to circuit training inference for proportions, including an answer key and a deep dive into the underlying statistical principles. We'll explore various problem types, highlighting the crucial steps involved in hypothesis testing and confidence interval construction. Mastering these techniques will bolster your understanding of statistical inference and empower you to tackle more complex problems with confidence.
Understanding Inference for Proportions
Before diving into the circuit training exercises, let's solidify our understanding of inference for proportions. This branch of statistics deals with estimating and testing hypotheses about population proportions (often denoted as 'p') based on sample data. We use sample proportions (denoted as 'p-hat') to make inferences about the population.
Key Concepts:
- Population Proportion (p): The true proportion of individuals in the population possessing a specific characteristic. This is often unknown and what we aim to estimate.
- Sample Proportion (p-hat): The proportion of individuals in a sample possessing the characteristic. This is calculated from the sample data and used as an estimate of p.
- Hypothesis Testing: A formal procedure used to determine whether there is enough evidence to reject a null hypothesis (a statement about the population proportion). This involves setting up null and alternative hypotheses, calculating a test statistic, and determining a p-value.
- Confidence Interval: A range of values within which we are confident (with a specified level of confidence, e.g., 95%) that the true population proportion lies. The width of the interval reflects the precision of our estimate.
- Significance Level (α): The probability of rejecting the null hypothesis when it is actually true (Type I error). Common values are 0.05 or 0.01.
- Margin of Error: Half the width of a confidence interval. It represents the uncertainty associated with our point estimate.
The Circuit Training Approach
Our circuit training will involve a series of problems, each focusing on a different aspect of inference for proportions. Each problem will be followed by a detailed solution. This iterative approach will build your confidence and understanding gradually.
Remember: Always clearly state your hypotheses, show your calculations, and interpret your results in the context of the problem.
Circuit Training Problems & Answer Key
Here's a series of problems designed to test your understanding of inference for proportions. Work through them step-by-step, referring to the explanations provided below.
Problem 1: Hypothesis Test for a Single Proportion
A researcher wants to determine if the proportion of adults who support a new policy is greater than 0.6. A random sample of 100 adults reveals that 68 support the policy. Test the hypothesis at a significance level of 0.05.
Answer Key:
-
State Hypotheses:
- Null Hypothesis (H₀): p ≤ 0.6
- Alternative Hypothesis (H₁): p > 0.6 (one-tailed test)
-
Calculate Test Statistic:
- p-hat = 68/100 = 0.68
- Standard Error (SE) = sqrt[(0.6 * 0.4)/100] = 0.049
- Z-statistic = (0.68 - 0.6) / 0.049 = 1.63
-
Determine P-value: Using a Z-table or statistical software, the p-value for a Z-score of 1.63 (one-tailed) is approximately 0.0516.
-
Make a Decision: Since the p-value (0.0516) is greater than the significance level (0.05), we fail to reject the null hypothesis.
-
Conclusion: There is not enough evidence to conclude that the proportion of adults who support the new policy is greater than 0.6.
Problem 2: Confidence Interval for a Single Proportion
A survey of 500 college students found that 300 prefer online learning. Construct a 95% confidence interval for the true proportion of college students who prefer online learning.
Answer Key:
-
Calculate Sample Proportion: p-hat = 300/500 = 0.6
-
Calculate Standard Error: SE = sqrt[(0.6 * 0.4)/500] = 0.0219
-
Find Critical Z-value: For a 95% confidence interval, the critical Z-value is 1.96.
-
Calculate Margin of Error: Margin of Error = 1.96 * 0.0219 = 0.0429
-
Construct Confidence Interval: Confidence Interval = 0.6 ± 0.0429 = (0.5571, 0.6429)
-
Conclusion: We are 95% confident that the true proportion of college students who prefer online learning lies between 0.5571 and 0.6429.
Problem 3: Hypothesis Test for the Difference Between Two Proportions
Two groups of patients are treated with different medications. In Group A (n=150), 80 patients show improvement, while in Group B (n=200), 110 show improvement. Test whether there's a significant difference in the proportion of patients who improve between the two groups at α = 0.01.
Answer Key:
-
State Hypotheses:
- H₀: pA - pB = 0
- H₁: pA - pB ≠ 0 (two-tailed test)
-
Calculate Sample Proportions:
- pA-hat = 80/150 = 0.533
- pB-hat = 110/200 = 0.55
-
Calculate Pooled Proportion: p = (80 + 110) / (150 + 200) = 0.545
-
Calculate Standard Error: SE = sqrt[p(1-p)(1/150 + 1/200)] = 0.052
-
Calculate Z-statistic: Z = (0.533 - 0.55) / 0.052 = -0.33
-
Determine P-value: The p-value for a Z-score of -0.33 (two-tailed) is approximately 0.74.
-
Make a Decision: Since the p-value (0.74) is much greater than the significance level (0.01), we fail to reject the null hypothesis.
-
Conclusion: There is not enough evidence to conclude a significant difference in the proportion of patients who improve between the two groups.
Problem 4: Confidence Interval for the Difference Between Two Proportions
Continuing with Problem 3, construct a 99% confidence interval for the difference in proportions between Group A and Group B.
Answer Key:
-
Calculate Sample Proportions: (As in Problem 3)
-
Calculate Standard Error: (As in Problem 3) Note: For a confidence interval, we don't use the pooled proportion. Instead, we use separate standard errors: SE = sqrt[(pA-hat*(1-pA-hat)/150) + (pB-hat*(1-pB-hat)/200)] ≈ 0.0527
-
Find Critical Z-value: For a 99% confidence interval, the critical Z-value is 2.576.
-
Calculate Margin of Error: Margin of Error = 2.576 * 0.0527 ≈ 0.135
-
Construct Confidence Interval: Confidence Interval = (0.533 - 0.55) ± 0.135 = (-0.252, 0.092)
-
Conclusion: We are 99% confident that the true difference in proportions of patients who improve between Group A and Group B lies between -0.252 and 0.092. Since the interval contains 0, this further supports the conclusion that there is no significant difference.
Deep Dive: Assumptions and Considerations
While the circuit training provides a practical approach, it's vital to understand the underlying assumptions and limitations:
-
Random Sampling: The inferences are valid only if the samples are randomly selected from the populations of interest. Non-random sampling can lead to biased estimates and unreliable conclusions.
-
Independence: Observations within each sample must be independent. This means that the outcome for one individual should not influence the outcome for another.
-
Sample Size: Sufficiently large sample sizes are crucial for the accuracy of the inferences. The Central Limit Theorem ensures that the sampling distribution of the sample proportion is approximately normal for large samples (typically, np ≥ 10 and n(1-p) ≥ 10). For small samples, alternative methods like the exact test or the Wilson score interval might be more appropriate.
-
Population Size: For finite populations, the finite population correction factor may be necessary to adjust the standard error. However, this factor is often negligible if the sample size is small relative to the population size.
Expanding Your Circuit Training
Once you've mastered these foundational problems, consider expanding your circuit training to include:
- More complex hypothesis tests: Incorporate two-tailed tests, tests with different significance levels, and problems involving larger datasets.
- Advanced confidence intervals: Explore different confidence levels and investigate the effect of sample size on interval width.
- Real-world applications: Apply your knowledge to analyze data from various fields, such as healthcare, marketing, and social sciences.
- Software utilization: Learn to use statistical software packages (e.g., R, SPSS, Python) to perform these calculations more efficiently and explore more advanced statistical techniques.
By consistently practicing and deepening your understanding of the underlying principles, you can build a solid foundation in statistical inference for proportions. Remember that the key is not just memorizing formulas but understanding the logic behind them and how to apply them to real-world scenarios. Continuous practice and exploration will transform your ability to interpret and communicate statistical results effectively.
Latest Posts
Latest Posts
-
For Variable Products Underlying Assets Must Be Kept In
Apr 22, 2025
-
Make Sure All Loads Carried By Equipment Are Stable And
Apr 22, 2025
-
Producers Can Generate Instant Sales By Using
Apr 22, 2025
-
What Is The Structure Indicated By Label E
Apr 22, 2025
-
More Than 50 Of Ecosystems Are Currently Being Used Unsustainably
Apr 22, 2025
Related Post
Thank you for visiting our website which covers about Circuit Training Inference For Proportions Answer Key . We hope the information provided has been useful to you. Feel free to contact us if you have any questions or need further assistance. See you next time and don't miss to bookmark.