Creating Phylogenetic Trees From Dna Sequences Answer Key
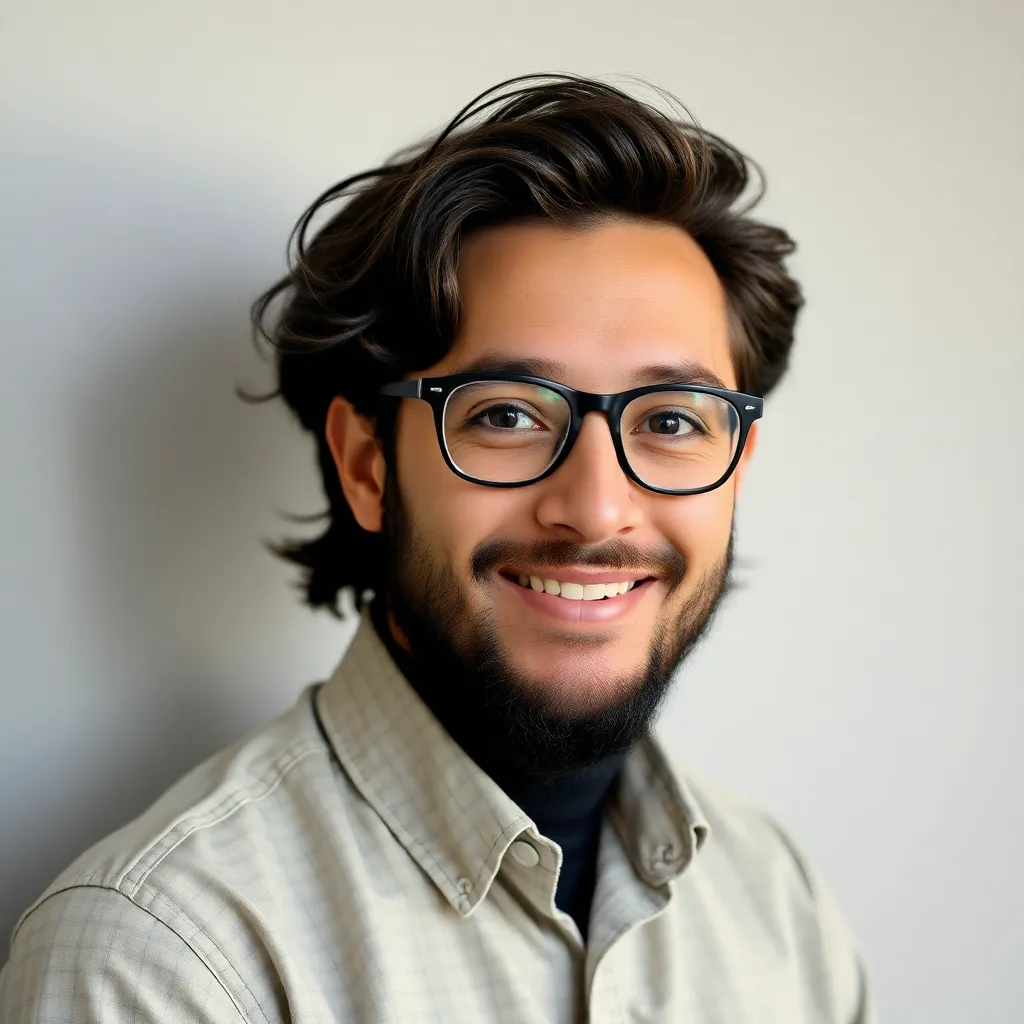
Breaking News Today
May 09, 2025 · 8 min read
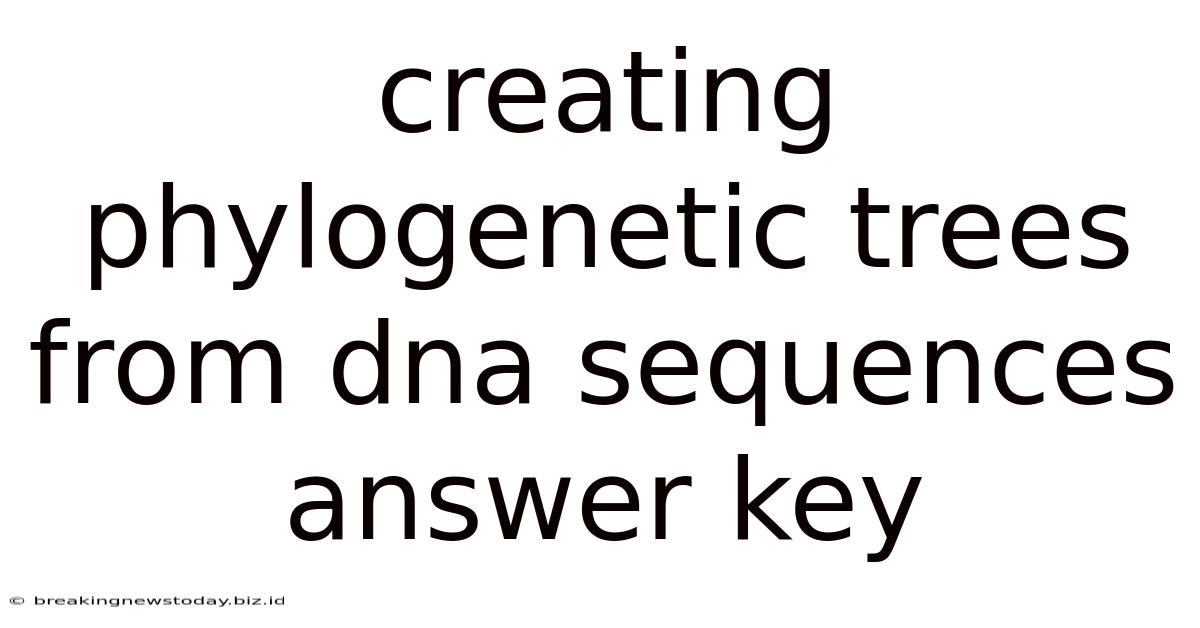
Table of Contents
Creating Phylogenetic Trees from DNA Sequences: A Comprehensive Guide
Phylogenetic trees, also known as phylogenies or evolutionary trees, are branching diagrams that depict the evolutionary relationships among various biological species or other entities based on their shared characteristics. Constructing these trees from DNA sequence data has revolutionized our understanding of the evolutionary history of life on Earth. This comprehensive guide will walk you through the process, from initial data preparation to tree visualization and interpretation. We'll explore different methods, their strengths and weaknesses, and offer practical tips for creating robust and informative phylogenetic trees.
1. Data Acquisition and Preparation: The Foundation of Phylogenetic Analysis
The journey begins with acquiring high-quality DNA sequence data. This typically involves selecting appropriate genes or genomic regions, depending on the research question and the taxa being studied. Choosing informative markers is crucial; regions with high variability are ideal for resolving relationships among closely related species, while more conserved regions are better suited for studying broader evolutionary patterns.
1.1 Data Sources and Selection
Data can be sourced from various databases like GenBank (NCBI), EMBL, and DDBJ. Consider factors like:
- Taxonomic representation: Include a diverse range of taxa, encompassing both ingroups (the species under study) and outgroups (more distantly related species used as a reference point).
- Sequence length and quality: Longer sequences generally provide greater phylogenetic resolution. Ensure high-quality sequences with minimal ambiguities or missing data.
- Gene choice: The choice of gene should align with the evolutionary question. Mitochondrial genes (e.g., Cytochrome c oxidase subunit I, COI) are commonly used for animal studies due to their rapid evolution, while nuclear genes are often preferred for resolving deeper evolutionary relationships.
1.2 Data Alignment: Essential for Accurate Comparisons
Before phylogenetic analysis, raw DNA sequences must be aligned. Alignment arranges sequences so that homologous positions (sites derived from a common ancestor) are vertically aligned. This process is crucial because misalignments can lead to inaccurate inferences of evolutionary relationships.
Several alignment tools exist, including:
- Multiple Sequence Alignment (MSA) programs: CLUSTAL Omega, MUSCLE, MAFFT. These algorithms employ different approaches to optimize alignment accuracy. Experimentation with different tools might be necessary to find the best alignment for your specific dataset.
- Manual adjustment: While automated tools are efficient, manual adjustments might be required to correct obvious errors in the alignment. This step is often crucial for accurate tree construction.
1.3 Data Cleaning and Filtering: Ensuring Data Integrity
After alignment, data cleaning is necessary to remove problematic regions. This involves:
- Removing ambiguous characters: Characters like "N" (unknown base) can negatively impact phylogenetic analyses. Consider removing columns with a high percentage of such characters.
- Handling gaps: Gaps (-), representing insertions or deletions, can be treated differently depending on the analytical method. Some methods penalize gaps more heavily than others.
- Partitioning the data: For datasets containing multiple genes, partitioning the data into separate gene alignments can improve the accuracy of the resulting tree, as different genes can evolve at different rates.
2. Phylogenetic Inference Methods: Choosing the Right Approach
Several methods are available for inferring phylogenetic trees from DNA sequence data. Each method has its own strengths and weaknesses, and the choice often depends on the dataset's characteristics and the research question.
2.1 Distance-based Methods: Simple and Fast but with Limitations
These methods first calculate a pairwise distance matrix, representing the genetic distance between each pair of sequences. Then, a tree is constructed that best reflects these distances. Examples include:
- Neighbor-Joining (NJ): A fast and widely used method, particularly suited for large datasets. However, it can be less accurate than other methods, especially when evolutionary rates vary significantly across lineages.
- UPGMA (Unweighted Pair Group Method with Arithmetic Mean): Similar to NJ, but assumes a constant rate of molecular evolution (molecular clock).
Limitations: Distance methods can be sensitive to the chosen distance metric and can be less accurate than character-based methods when evolutionary rates vary among lineages.
2.2 Character-based Methods: More Accurate but Computationally Intensive
These methods analyze the characters (nucleotides in DNA sequences) directly without first calculating distances. They are often more accurate than distance-based methods, particularly when evolutionary rates are heterogeneous.
- Maximum Parsimony (MP): Finds the tree that requires the fewest evolutionary changes (mutations) to explain the observed data. It's relatively simple to understand but can be computationally intensive for large datasets and might be prone to finding multiple equally parsimonious trees.
- Maximum Likelihood (ML): Finds the tree that has the highest probability of generating the observed data, given a specific model of molecular evolution. It's statistically more rigorous than MP but can be computationally demanding.
- Bayesian Inference (BI): A probabilistic method that estimates the posterior probability of different trees, considering prior information and the likelihood of the data. It provides a measure of the uncertainty associated with the inferred tree. BI often offers strong support values for nodes in the resulting phylogeny.
Advantages: Character-based methods generally provide more accurate and reliable results, especially when evolutionary rates vary among lineages or when there's substantial homoplasy (convergent or parallel evolution).
2.3 Choosing the Right Method
The best method depends on several factors:
- Dataset size: Distance methods are generally faster for large datasets, while character-based methods are preferred for smaller, more complex datasets.
- Evolutionary rate heterogeneity: Character-based methods (especially ML and BI) are better suited for datasets with varying evolutionary rates.
- Computational resources: ML and BI can be computationally intensive, requiring significant processing power and time.
3. Tree Visualization and Interpretation: Communicating Evolutionary Relationships
Once a phylogenetic tree is inferred, visualizing and interpreting it is crucial. Several software packages can create visually appealing and informative trees. These often allow customizing features such as:
- Branch lengths: Represent the amount of evolutionary change along each branch.
- Node labels: Identify the taxa represented by each branch tip.
- Node support values: Indicate the confidence in the branching pattern (e.g., bootstrap values for MP and ML, posterior probabilities for BI).
- Tree layouts: Different layouts (e.g., cladogram, dendrogram, phylogram) can enhance interpretability.
Interpreting a phylogenetic tree involves:
- Identifying clades: Clades are groups of organisms that share a common ancestor. Clades are often identified by monophyletic groups where all descendants of a common ancestor are included.
- Understanding branch lengths: Longer branches generally indicate more evolutionary change.
- Assessing node support: Higher support values suggest greater confidence in the branching pattern. Generally, values above 70% (for bootstrap) or 0.95 (for posterior probabilities) are considered strong support.
- Relating the tree to the biological question: The final step is integrating the phylogenetic tree with existing knowledge and the original research question.
4. Software and Tools: Practical Resources for Phylogenetic Analysis
Numerous software packages facilitate phylogenetic analysis. Some popular choices include:
- MEGA X: A user-friendly program that offers a range of phylogenetic methods, including distance-based, parsimony, and maximum likelihood approaches.
- PhyML: A powerful maximum likelihood program known for its speed and accuracy.
- MrBayes: A widely used Bayesian inference program that provides robust statistical support for phylogenetic relationships.
- RAxML: Another popular maximum likelihood program known for its efficiency in handling large datasets.
- PAUP:* A versatile program offering various phylogenetic inference methods and tree manipulation tools.
5. Assessing Tree Reliability: Evaluating the Robustness of Your Phylogeny
No phylogenetic inference method is perfect; all estimations contain some degree of uncertainty. Several techniques help evaluate tree reliability:
- Bootstrap analysis: Resampling the data multiple times and constructing trees from each resample provides a measure of the confidence in each branch. High bootstrap values (generally above 70%) suggest strong support for a particular branching pattern.
- Posterior probability analysis (Bayesian inference): The posterior probability of a node provides a measure of the confidence in that node's placement in the tree. Values above 0.95 generally indicate strong support.
- Consensus trees: Combining results from multiple analyses (e.g., different methods or different datasets) can improve the reliability of the final tree. Consensus trees represent the common elements among multiple individual trees.
6. Beyond the Basics: Advanced Techniques in Phylogenetic Analysis
As you progress, you may explore advanced techniques such as:
- Model selection: Choosing the most appropriate model of molecular evolution can significantly impact the accuracy of phylogenetic inference. Model selection criteria such as AIC (Akaike Information Criterion) and BIC (Bayesian Information Criterion) are commonly used.
- Dealing with horizontal gene transfer (HGT): HGT complicates phylogenetic analyses, especially in prokaryotes. Advanced methods are used to account for HGT and reconstruct accurate phylogenetic trees.
- Phylogenetic networks: These are extensions of phylogenetic trees that can represent reticulate evolutionary events, such as HGT or hybridization.
- Dating phylogenies (molecular clocks): Methods exist to estimate divergence times in a phylogeny. These often rely on calibrating the tree with fossil data or other independent time constraints.
7. Conclusion: Phylogenetic Trees as Powerful Tools in Evolutionary Biology
Constructing phylogenetic trees from DNA sequences is a powerful tool for investigating evolutionary relationships and understanding the history of life. This detailed guide provides a comprehensive overview of the process, from data acquisition and preparation to tree visualization and interpretation. By understanding the different methods, their strengths and weaknesses, and the importance of evaluating tree reliability, researchers can utilize this powerful technique to address a wide range of biological questions. Remember, meticulous data handling and thoughtful method selection are critical for constructing robust and informative phylogenetic trees that significantly contribute to our understanding of the evolutionary processes shaping the biological world.
Latest Posts
Latest Posts
-
Chapter 5 Supply Economics Worksheet Answers Pdf
May 11, 2025
-
Which Is A Cognitive Technique For Stress Management
May 11, 2025
-
What Colonys Founders Believed That Tolerance Was A Great Virtue
May 11, 2025
-
Taking An Iron Supplement During Childhood Can Prevent Blindness
May 11, 2025
-
Which Of The Following Statements Would Apply To Shaquan
May 11, 2025
Related Post
Thank you for visiting our website which covers about Creating Phylogenetic Trees From Dna Sequences Answer Key . We hope the information provided has been useful to you. Feel free to contact us if you have any questions or need further assistance. See you next time and don't miss to bookmark.