Elementary Statistics: A Step By Step Approach
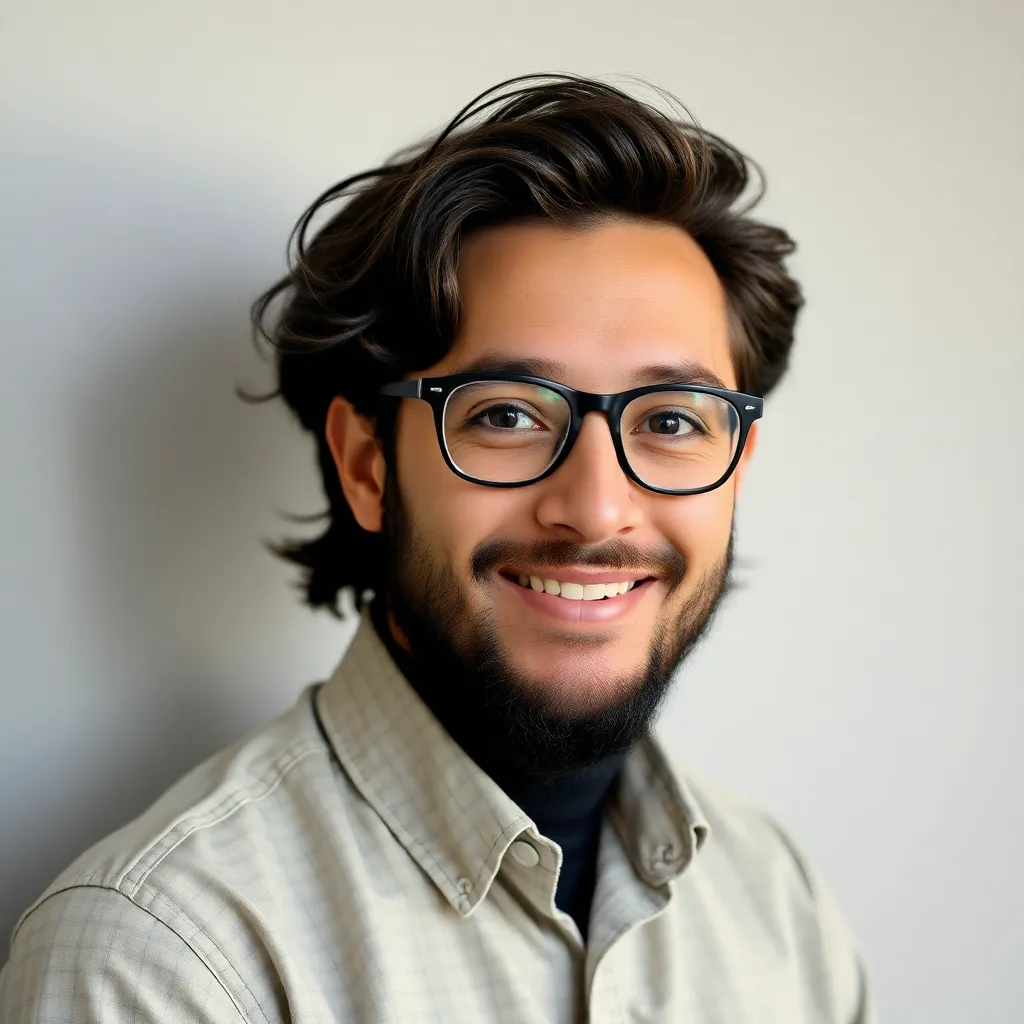
Breaking News Today
Apr 23, 2025 · 7 min read

Table of Contents
Elementary Statistics: A Step-by-Step Approach
Understanding statistics can feel daunting, especially when faced with complex formulas and jargon. However, the core principles of elementary statistics are surprisingly accessible and incredibly useful in everyday life. This comprehensive guide provides a step-by-step approach to understanding and applying fundamental statistical concepts, making the subject less intimidating and more engaging. We'll cover everything from descriptive statistics to inferential statistics, equipping you with the tools to analyze data effectively.
I. Descriptive Statistics: Summarizing and Presenting Data
Descriptive statistics are methods used to summarize and present data in a meaningful way. They provide a concise overview of the dataset, highlighting key features and trends without drawing conclusions about a larger population. Let's explore the key elements:
A. Measures of Central Tendency
These statistics describe the "center" or "typical" value of a dataset. The most common measures are:
-
Mean: The average of all values. Calculated by summing all values and dividing by the number of values. This is sensitive to outliers (extreme values).
-
Median: The middle value when the data is arranged in ascending order. Less sensitive to outliers than the mean. For an even number of data points, the median is the average of the two middle values.
-
Mode: The value that appears most frequently in the dataset. A dataset can have multiple modes or no mode at all.
Example: Consider the dataset: 2, 4, 4, 6, 8, 10.
- Mean: (2 + 4 + 4 + 6 + 8 + 10) / 6 = 5.67
- Median: (4 + 6) / 2 = 5
- Mode: 4
B. Measures of Dispersion (Variability)
These statistics describe the spread or variability of the data. How spread out are the values?
-
Range: The difference between the highest and lowest values in the dataset. Simple but highly sensitive to outliers.
-
Variance: The average of the squared differences from the mean. It measures the average squared deviation from the mean. A higher variance indicates greater dispersion.
-
Standard Deviation: The square root of the variance. Expressed in the same units as the original data, making it easier to interpret than the variance.
Example (using the same dataset):
- Range: 10 - 2 = 8
- Variance: A more complex calculation, involving squaring the difference between each data point and the mean, summing those squared differences, and then dividing by the number of data points. (Calculation omitted for brevity, but easily performed using statistical software or calculators.)
- Standard Deviation: The square root of the variance.
C. Data Visualization
Presenting data visually is crucial for understanding patterns and trends. Common methods include:
-
Histograms: Show the frequency distribution of a continuous variable.
-
Bar Charts: Show the frequency distribution of a categorical variable.
-
Pie Charts: Show the proportion of each category in a whole.
-
Box Plots (Box and Whisker Plots): Display the median, quartiles, and range of a dataset, highlighting outliers. Excellent for comparing distributions across different groups.
Effective visualization makes complex datasets easily digestible and helps identify key features that might be missed when looking only at numerical summaries.
II. Probability: Understanding Uncertainty
Probability is the foundation of inferential statistics. It deals with the likelihood of events occurring. Key concepts include:
A. Basic Probability Concepts
-
Experiment: A process that produces a well-defined outcome.
-
Sample Space: The set of all possible outcomes of an experiment.
-
Event: A subset of the sample space.
-
Probability: The likelihood of an event occurring, expressed as a number between 0 and 1 (0% to 100%). A probability of 0 means the event is impossible, while a probability of 1 means the event is certain.
B. Types of Probability
-
Classical Probability: Assumes all outcomes are equally likely. Calculated as the ratio of favorable outcomes to the total number of possible outcomes.
-
Empirical Probability: Based on observed data. Calculated as the ratio of the number of times an event occurred to the total number of trials.
-
Subjective Probability: Based on personal judgment or belief.
C. Probability Rules
Understanding probability rules is crucial for more advanced statistical analysis. Key rules include:
-
Addition Rule: Used to find the probability of either of two events occurring.
-
Multiplication Rule: Used to find the probability of two events both occurring.
-
Conditional Probability: The probability of an event occurring given that another event has already occurred.
III. Inferential Statistics: Drawing Conclusions from Data
Inferential statistics uses sample data to make inferences about a larger population. This involves estimating population parameters and testing hypotheses.
A. Sampling Distributions
The sampling distribution is the probability distribution of a sample statistic (e.g., the sample mean). Understanding the sampling distribution is crucial for making inferences about the population. The Central Limit Theorem states that, under certain conditions, the sampling distribution of the mean will be approximately normal, regardless of the shape of the population distribution. This is a cornerstone of many statistical tests.
B. Confidence Intervals
Confidence intervals provide a range of values within which a population parameter (e.g., the population mean) is likely to fall. For example, a 95% confidence interval means that there's a 95% probability that the interval contains the true population parameter. The width of the confidence interval depends on the sample size and the variability of the data. Larger samples generally lead to narrower intervals.
C. Hypothesis Testing
Hypothesis testing is a formal procedure used to determine whether there is enough evidence to reject a null hypothesis. The null hypothesis is a statement about a population parameter (e.g., the population mean is equal to a specific value). The alternative hypothesis is the opposite of the null hypothesis. Hypothesis testing involves calculating a test statistic, determining a p-value (the probability of observing the data if the null hypothesis is true), and comparing the p-value to a significance level (alpha, typically 0.05). If the p-value is less than alpha, we reject the null hypothesis.
D. Common Statistical Tests
Several statistical tests are used to analyze different types of data and answer different research questions. Some common examples include:
-
t-test: Used to compare the means of two groups.
-
ANOVA (Analysis of Variance): Used to compare the means of three or more groups.
-
Chi-square test: Used to analyze categorical data and test for independence between variables.
-
Correlation analysis: Used to measure the strength and direction of the linear relationship between two continuous variables. Correlation does not imply causation. Regression analysis builds upon correlation to model the relationship and make predictions.
IV. Choosing the Right Statistical Method
Selecting the appropriate statistical method depends on several factors:
-
Type of data: Categorical (nominal or ordinal) or continuous (interval or ratio).
-
Research question: What are you trying to find out? Are you comparing groups, measuring associations, or making predictions?
-
Assumptions of the statistical test: Many statistical tests have assumptions about the data (e.g., normality, independence). It’s crucial to check these assumptions before applying the test.
V. Software for Statistical Analysis
Several software packages are available for performing statistical analyses, including:
-
R: A free and open-source software environment for statistical computing and graphics. Powerful and flexible, but can have a steep learning curve.
-
SPSS: A commercial statistical software package widely used in academia and industry. User-friendly interface, but requires a license.
-
Python with libraries like SciPy and Statsmodels: Python's versatility, combined with these powerful libraries, offers a robust and flexible approach to statistical analysis, particularly suitable for integrating statistics with other programming tasks.
VI. Conclusion
Elementary statistics provides a powerful toolkit for understanding and interpreting data. By mastering the fundamental concepts of descriptive and inferential statistics, you can gain valuable insights from data in various contexts. Remember to choose appropriate statistical methods, carefully consider assumptions, and use visualization to communicate your findings effectively. While the initial learning curve might feel steep, the ability to analyze and interpret data is a highly valuable skill in today's data-driven world. Continuous practice and exploration are key to solidifying your understanding and becoming proficient in applying these methods. Remember to always strive for clarity and accuracy in your analyses and interpretations.
Latest Posts
Latest Posts
-
Select All The Descriptions About An Estampie
Apr 23, 2025
-
What Is The Advantage Of Using A Wet Mount
Apr 23, 2025
-
Which Medication Is Responsible For Neonatal Hypoglycemia
Apr 23, 2025
-
Are You Smarter Than A 1st Grader Questions
Apr 23, 2025
-
A Social Problem Is Defined As A Condition That
Apr 23, 2025
Related Post
Thank you for visiting our website which covers about Elementary Statistics: A Step By Step Approach . We hope the information provided has been useful to you. Feel free to contact us if you have any questions or need further assistance. See you next time and don't miss to bookmark.