Estimating Population Proportions Lesson 20 Answer Key
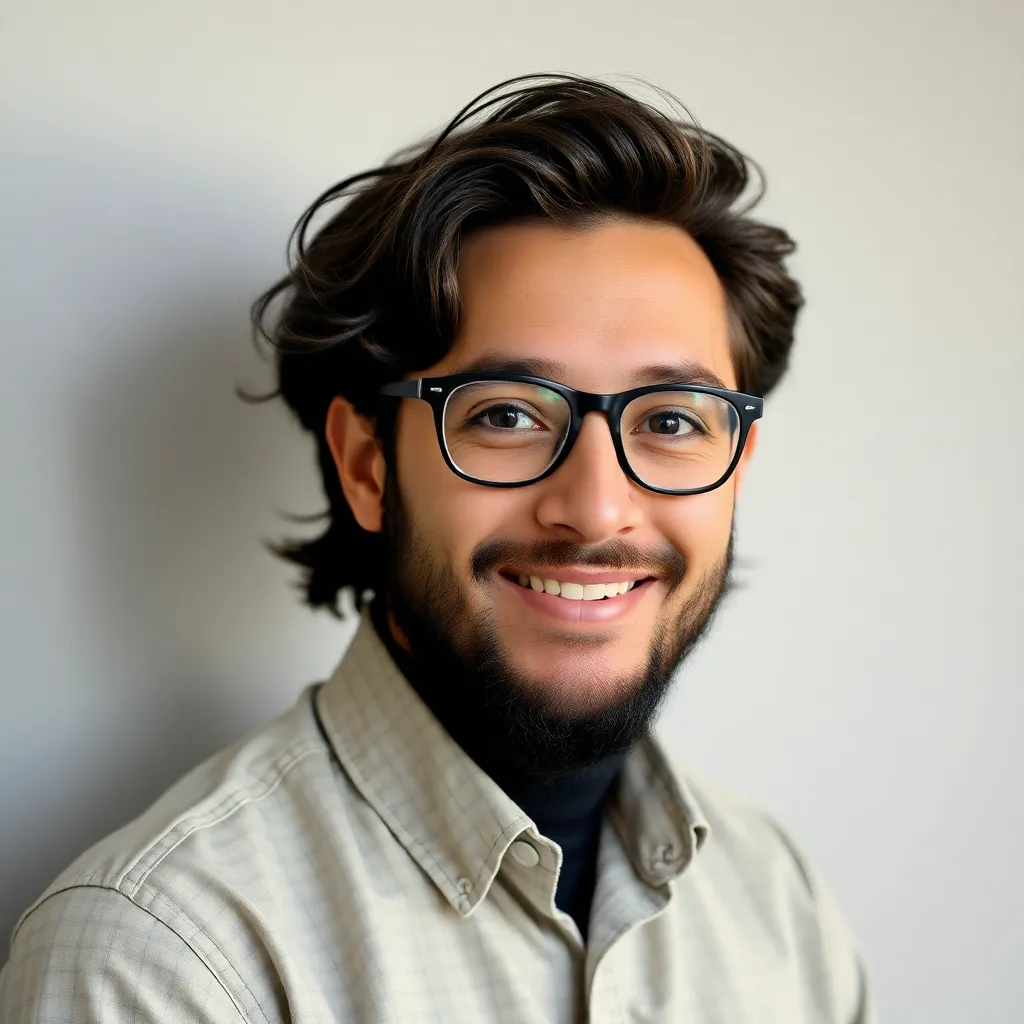
Breaking News Today
May 09, 2025 · 5 min read
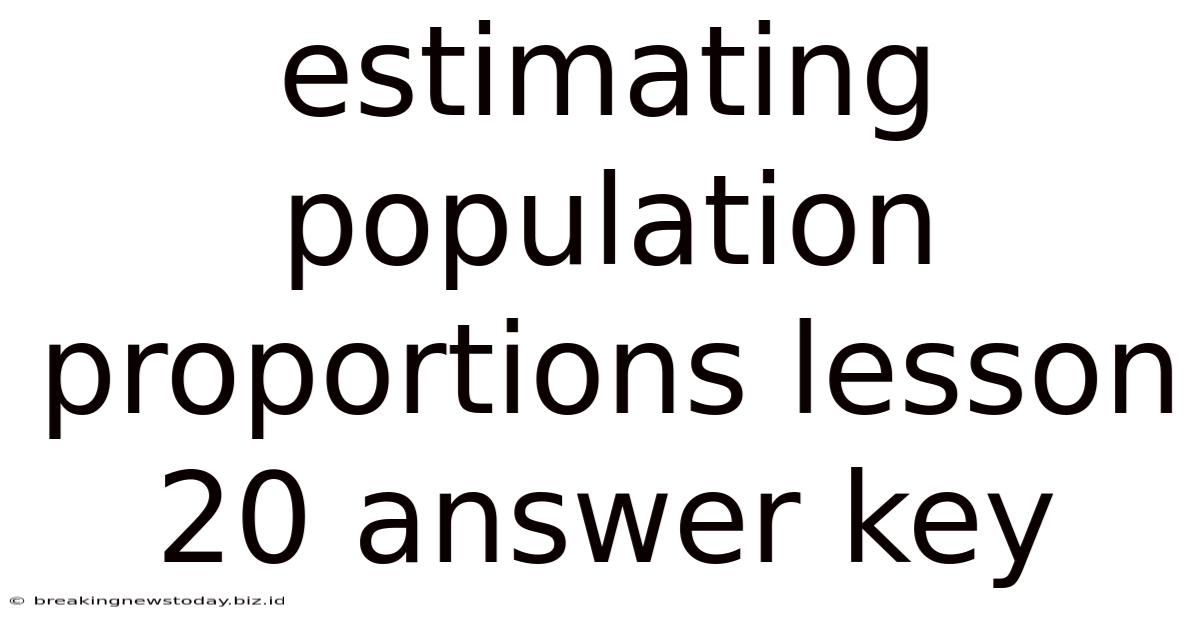
Table of Contents
Estimating Population Proportions: Lesson 20 Answer Key & Deep Dive
This comprehensive guide delves into the intricacies of estimating population proportions, providing not only a detailed "answer key" for a hypothetical Lesson 20 but also a thorough exploration of the underlying statistical concepts and practical applications. We’ll cover key formulas, interpret confidence intervals, examine different sampling methods, and discuss the impact of sample size on the accuracy of estimations.
Understanding Population Proportions
Before diving into the hypothetical Lesson 20, let's solidify our understanding of the core concept: population proportions. A population proportion (often denoted as 'p') represents the fraction of individuals within a population possessing a specific characteristic or attribute. For instance, if we're interested in the proportion of voters who favor a particular candidate, 'p' would represent the true, unknown percentage of the entire voting population supporting that candidate.
Since it's often impossible to survey an entire population, we rely on sample proportions (denoted as 'p-hat'). This is the proportion of individuals exhibiting the characteristic of interest within a randomly selected sample from the population. The goal of inferential statistics is to use this sample proportion to make accurate estimations about the population proportion.
Lesson 20 Hypothetical Scenario & Answer Key
Let's imagine Lesson 20 presents the following problem:
Scenario: A researcher wants to estimate the proportion of adults in a city who regularly recycle. A random sample of 400 adults is surveyed, and 280 report that they regularly recycle. Construct a 95% confidence interval for the population proportion.
Answer Key:
-
Calculate the sample proportion (p-hat): p-hat = 280/400 = 0.7
-
Determine the critical value (z):* For a 95% confidence interval, the critical z-value is 1.96 (this is a standard value found in most statistical tables or calculated using statistical software).
-
Calculate the standard error: The standard error (SE) measures the variability of the sample proportion. The formula is:
SE = sqrt[ (p-hat * (1 - p-hat)) / n ]
Where 'n' is the sample size (400 in this case).
SE = sqrt[ (0.7 * 0.3) / 400 ] ≈ 0.0229
-
Calculate the margin of error (ME): The margin of error represents the range of error around the sample proportion.
ME = z* * SE = 1.96 * 0.0229 ≈ 0.0449
-
Construct the 95% confidence interval: The confidence interval provides a range of values within which the true population proportion is likely to fall.
Confidence Interval = p-hat ± ME = 0.7 ± 0.0449
Therefore, the 95% confidence interval is approximately (0.6551, 0.7449).
Interpretation: We are 95% confident that the true proportion of adults in the city who regularly recycle lies between 65.51% and 74.49%.
Delving Deeper: Key Concepts & Considerations
This hypothetical example showcases the core steps. However, a complete understanding necessitates exploring several crucial concepts:
1. Sampling Methods: The Foundation of Accurate Estimation
The reliability of our population proportion estimate hinges critically on the sampling method. A random sample, where each member of the population has an equal chance of selection, is essential to minimize bias. Other sampling methods, such as stratified sampling (dividing the population into strata and sampling from each) or cluster sampling (sampling clusters of individuals), can also be employed, but careful consideration of their potential biases is crucial.
2. Sample Size and Margin of Error: A Delicate Balance
The sample size (n) plays a pivotal role in determining the margin of error. Larger sample sizes generally lead to smaller margins of error, resulting in more precise estimates. However, increasing sample size also increases the cost and time investment. Statisticians often use power analysis to determine the optimal sample size needed to achieve a desired level of precision.
3. Confidence Level and Confidence Intervals: Understanding the Uncertainty
The confidence level expresses the degree of certainty associated with the confidence interval. A 95% confidence level, as in our example, means that if we were to repeat the sampling process many times, 95% of the resulting confidence intervals would contain the true population proportion. Higher confidence levels (e.g., 99%) lead to wider intervals, reflecting increased certainty but reduced precision.
4. Assumptions and Limitations: Recognizing the Constraints
The accuracy of estimating population proportions relies on certain assumptions, including:
- Random Sampling: The sample must be truly random to avoid bias.
- Independence: The observations within the sample should be independent of each other.
- Large Sample Size: While there are methods for smaller samples, a sufficiently large sample size (often considered np-hat ≥ 10 and n(1-p-hat) ≥ 10) ensures the validity of the normal approximation used in calculating the confidence interval.
5. Beyond the Basics: More Advanced Techniques
For more complex situations, more advanced techniques may be necessary:
- Finite Population Correction: When the sample size is a significant proportion of the population, a finite population correction factor is needed to adjust the standard error.
- Hypothesis Testing: Instead of just estimating the proportion, we might want to test a specific hypothesis about the population proportion (e.g., Is the proportion of recycling adults greater than 70%? ).
- Proportions from Different Groups: Comparing proportions between different groups (e.g., comparing recycling rates between men and women) requires more sophisticated methods like two-proportion z-tests.
Practical Applications: Real-World Uses of Estimating Population Proportions
Estimating population proportions finds wide-ranging applications across diverse fields:
- Market Research: Determining consumer preferences, brand awareness, or the potential market size for a new product.
- Public Opinion Polls: Gauging public sentiment on political issues, social trends, or policy decisions.
- Medical Research: Estimating the prevalence of diseases, the effectiveness of treatments, or the incidence of adverse events.
- Quality Control: Assessing the proportion of defective items in a manufacturing process.
- Environmental Studies: Estimating the proportion of a population affected by pollution or other environmental hazards.
Conclusion: Mastering the Art of Estimation
Estimating population proportions is a fundamental skill in statistics, with far-reaching implications across various disciplines. By understanding the underlying principles, mastering the calculations, and critically evaluating the assumptions and limitations, you can effectively use sample data to draw reliable inferences about the characteristics of a larger population. While this "answer key" provides a starting point, the true power of this statistical method lies in its application to real-world problems, demanding a deep understanding of both the statistical mechanics and the context of the problem at hand. Remember to always consider the sampling method employed, critically evaluate the margin of error and confidence level, and be mindful of the limitations inherent in any statistical estimation process.
Latest Posts
Latest Posts
-
A Can Be Used To Douse A Fire
May 10, 2025
-
The Medical Term Meaning Blood Within A Joint Is
May 10, 2025
-
Label The Phases Of The Cell Cycle
May 10, 2025
-
What Are The Products Of The Light Dependent Reactions
May 10, 2025
-
Which Of The Following Is An Unbiased Strategy
May 10, 2025
Related Post
Thank you for visiting our website which covers about Estimating Population Proportions Lesson 20 Answer Key . We hope the information provided has been useful to you. Feel free to contact us if you have any questions or need further assistance. See you next time and don't miss to bookmark.