Factor Analysis Has Been Used To Identify The Most Basic
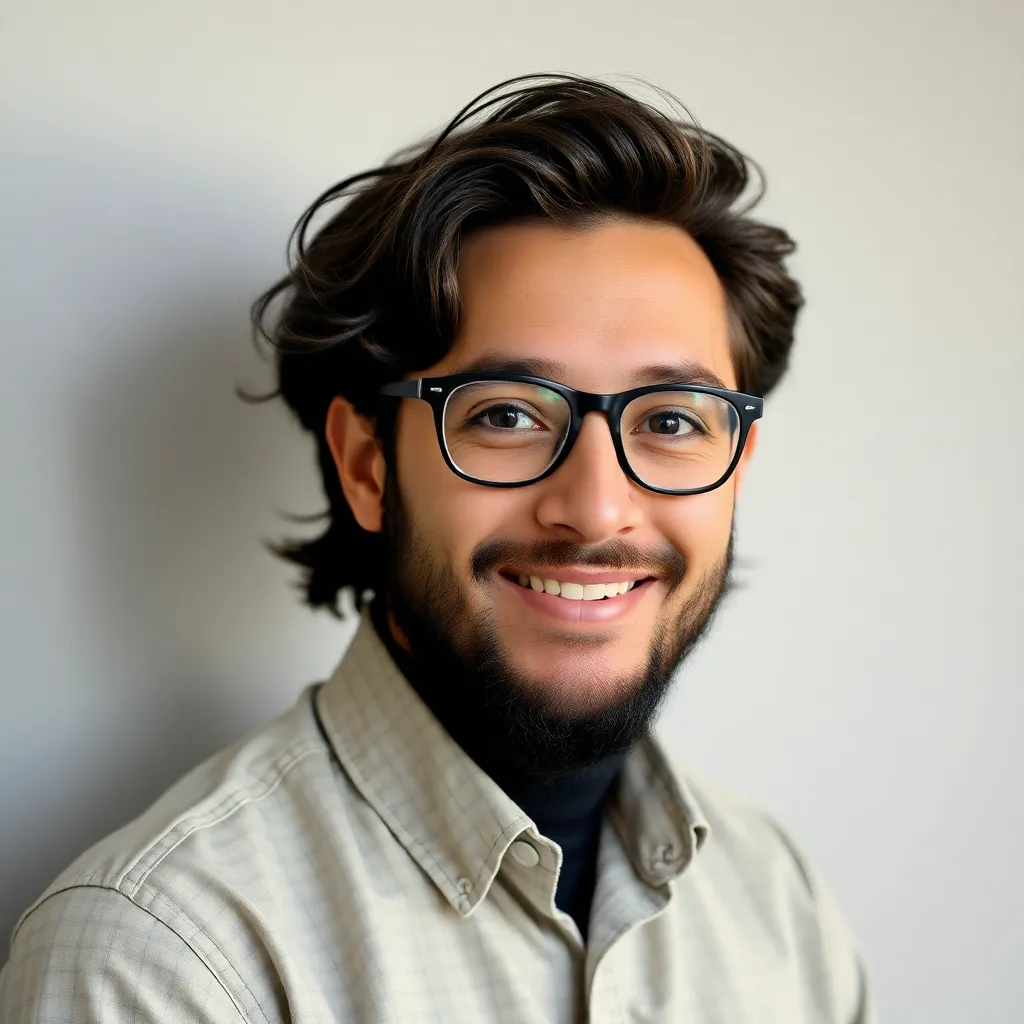
Breaking News Today
Apr 04, 2025 · 6 min read

Table of Contents
Factor Analysis: Unveiling the Underlying Structure of Complex Data
Factor analysis is a powerful statistical method used to uncover the underlying structure of a large dataset. It's a technique that simplifies complex information by identifying a smaller number of latent factors that explain the correlations among a larger set of observed variables. Instead of dealing with numerous individual variables, researchers can focus on a smaller set of factors, making the data easier to interpret and understand. This article delves deep into the application of factor analysis, exploring its various types, assumptions, steps involved, interpretation of results, and common applications. We'll examine how factor analysis has been used to identify the most basic, underlying factors driving complex phenomena across various fields.
Understanding the Core Concept: Latent Variables and Factors
At the heart of factor analysis lies the concept of latent variables. These are unobserved variables that are presumed to influence the observed variables in a dataset. Think of it like this: you might observe various symptoms (observed variables) of a disease, but the underlying disease itself (latent variable) is not directly observable. Factor analysis aims to identify these latent variables, which are then termed factors. These factors represent the common underlying dimensions that explain the correlations between the observed variables.
For example, consider a study investigating consumer preferences for different brands of coffee. Researchers might collect data on various attributes, such as taste, aroma, price, and caffeine content. Factor analysis could reveal that these attributes cluster into two main factors: "overall quality" (combining taste and aroma) and "value for money" (combining price and caffeine content). These two factors are the latent variables driving the observed preferences.
Types of Factor Analysis
There are two main types of factor analysis:
1. Exploratory Factor Analysis (EFA)
EFA is used when there's limited or no prior knowledge about the underlying structure of the data. Its primary goal is to discover the latent factors and their relationships with the observed variables. EFA is a data-driven approach that allows researchers to explore the data without pre-conceived notions. The number of factors to extract is often determined through techniques like eigenvalues greater than one, scree plots, or parallel analysis. Rotation techniques, such as varimax or oblimin, are employed to simplify the interpretation of the factor loadings.
2. Confirmatory Factor Analysis (CFA)
CFA, on the other hand, is used when researchers have a pre-defined hypothesis about the structure of the data. It tests a specific model, confirming or rejecting whether the hypothesized factors explain the observed data. CFA uses statistical tests to assess the goodness of fit of the model, providing a quantitative measure of how well the model fits the data. This method is more rigorous than EFA and provides stronger evidence for the underlying structure.
Assumptions of Factor Analysis
Before conducting factor analysis, it's crucial to ensure the data meets certain assumptions:
- Linearity: The relationships between the variables should be approximately linear.
- Normality: The variables should be approximately normally distributed. While minor deviations are acceptable, significant departures can affect the results.
- Sample Size: A sufficiently large sample size is necessary for reliable results. General guidelines suggest a minimum ratio of cases to variables, often 5:1 or 10:1.
- Absence of Multicollinearity: High correlations between variables can lead to instability in the factor analysis.
- No Outliers: Outliers can significantly influence the results, and their presence should be investigated and addressed.
Steps Involved in Conducting Factor Analysis
The process of conducting factor analysis typically involves several key steps:
- Data Collection: Gather the necessary data on the observed variables.
- Data Preparation: This includes checking for missing data, outliers, and assessing the assumptions of factor analysis.
- Correlation Matrix: Compute the correlation matrix to examine the relationships between the variables.
- Factor Extraction: Determine the number of factors to extract using techniques like eigenvalues greater than one, scree plots, or parallel analysis.
- Factor Rotation: Rotate the factors to improve interpretability using methods like varimax (orthogonal) or oblimin (oblique).
- Factor Interpretation: Name the factors based on the variables with high loadings on each factor.
- Factor Scores: Calculate factor scores to represent each case's position on the identified factors.
Interpreting the Results of Factor Analysis
Interpreting factor analysis results requires careful examination of several key outputs:
- Eigenvalues: Eigenvalues represent the variance explained by each factor. Factors with larger eigenvalues explain more variance.
- Eigenvectors (Loadings): Loadings indicate the correlation between each variable and each factor. High loadings suggest a strong relationship.
- Communalities: Communalities represent the proportion of variance in each variable explained by the extracted factors.
- Factor Scores: Factor scores represent each case's score on each identified factor.
Applications of Factor Analysis Across Diverse Fields
Factor analysis has proven to be an invaluable tool in a wide range of disciplines, including:
1. Psychology:
- Personality assessment: Identifying underlying personality traits from a large number of observed behaviors. The widely used Big Five personality traits (openness, conscientiousness, extraversion, agreeableness, and neuroticism) were identified using factor analysis.
- Psychopathology: Identifying underlying dimensions of psychological disorders.
2. Marketing Research:
- Brand perception: Understanding consumer perceptions of brands based on various attributes.
- Market segmentation: Grouping consumers based on their shared preferences and attitudes.
- Product development: Identifying key product attributes that are important to consumers.
3. Education:
- Assessment of learning outcomes: Identifying underlying constructs measured by different test items.
- Curriculum development: Identifying key skills and knowledge areas that contribute to student success.
4. Finance:
- Portfolio management: Identifying underlying factors that drive returns in the financial markets.
- Risk assessment: Identifying factors contributing to financial risk.
5. Social Sciences:
- Social attitudes: Identifying underlying dimensions of social attitudes and opinions.
- Political behavior: Understanding the factors influencing voting behavior and political participation.
6. Health Sciences:
- Quality of life assessment: Identifying key dimensions of quality of life related to health conditions.
- Health behaviors: Understanding the factors that influence health behaviors, such as smoking and exercise.
Advantages and Limitations of Factor Analysis
Advantages:
- Data reduction: Simplifies complex data by reducing the number of variables.
- Identification of latent variables: Uncovers underlying factors that are not directly observable.
- Improved interpretation: Makes the data easier to interpret and understand.
- Hypothesis testing: Allows for the testing of hypotheses about the underlying structure of the data (in CFA).
Limitations:
- Subjectivity: The interpretation of factors can be subjective.
- Assumptions: Requires the data to meet certain assumptions.
- Sensitivity to sample size: Requires a sufficiently large sample size for reliable results.
- Over-interpretation: Can lead to over-interpretation if not carefully applied.
Conclusion
Factor analysis is a powerful technique for uncovering the underlying structure of complex data. Its applications span diverse fields, providing valuable insights into the relationships between variables and identifying latent factors that drive observed phenomena. By understanding the principles, assumptions, and interpretation of factor analysis, researchers can effectively use this tool to simplify complex data and gain deeper insights into the phenomena under study. However, it’s crucial to carefully consider the assumptions and limitations of the method, interpret results cautiously, and avoid over-interpretation to ensure the validity and reliability of the findings. The judicious application of factor analysis, particularly in conjunction with other statistical methods, can be a powerful means to advance knowledge and understanding across a wide range of disciplines.
Latest Posts
Latest Posts
-
The Emergent Care Clinic Hesi Case Study
Apr 11, 2025
-
What Motivates Odysseus To Tell A Lie To The Cyclops
Apr 11, 2025
-
Tammy Has A Positive View Of Challenges
Apr 11, 2025
-
What Is The First Step To Preparing An Effective Speech
Apr 11, 2025
-
Preguntas Y Respuestas Del Examen De Manejo De New Jersey
Apr 11, 2025
Related Post
Thank you for visiting our website which covers about Factor Analysis Has Been Used To Identify The Most Basic . We hope the information provided has been useful to you. Feel free to contact us if you have any questions or need further assistance. See you next time and don't miss to bookmark.