Fill In The Blanks In Symbol Column Of The Table.
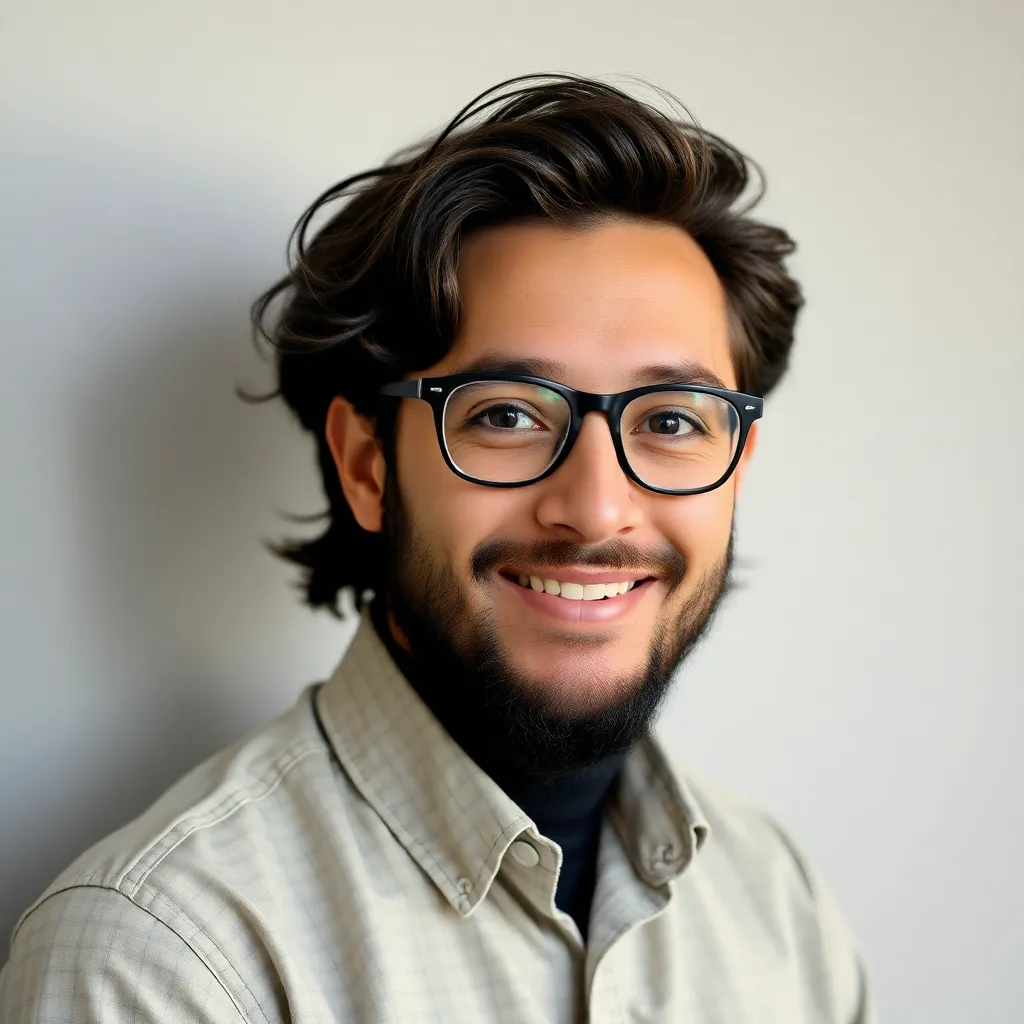
Breaking News Today
Apr 24, 2025 · 6 min read

Table of Contents
Filling in the Blanks: A Comprehensive Guide to Symbol Columns in Tables
Data tables are fundamental to organizing and presenting information clearly. However, sometimes tables contain gaps, specifically in symbol columns. These gaps can hinder analysis and interpretation. This comprehensive guide will explore various strategies and techniques for effectively filling in the blanks in symbol columns of your tables, considering different scenarios and potential challenges. We'll cover everything from simple imputation methods to more advanced statistical approaches, ensuring you can choose the best technique for your specific data.
Understanding Symbol Columns and Missing Data
Before diving into the methods, let's clarify what we mean by "symbol columns" and "missing data" in this context. A symbol column, in a table, utilizes symbols (e.g., +, -, *, #, ✓, ✗) instead of numerical or textual values to represent categorical data, qualitative observations, or specific characteristics. Missing data, or blanks, in this column signify the absence of information for a particular observation or data point. These missing data points can significantly impact the analysis and validity of any conclusions drawn from the table.
The reasons for missing data in symbol columns are diverse:
- Data collection errors: Errors during the data collection process can lead to missing entries. For example, a researcher might have missed recording a specific observation.
- Data entry errors: Mistakes during data entry can also result in missing values. This is especially true if data entry is manual.
- Non-response: Participants or respondents might choose not to answer specific questions, resulting in missing data.
- Data limitations: Some data simply might not be available. This might be due to limitations in the technology used, or the nature of the data itself.
Methods for Filling in Blank Spaces in Symbol Columns
Addressing missing data in symbol columns requires careful consideration. The optimal method depends heavily on the context of the data, the reason for the missing values, and the desired outcome of your analysis. Here are some strategies, ranging from simple to more sophisticated:
1. Manual Inspection and Filling
The most straightforward method involves carefully reviewing the data and attempting to infer the missing symbols based on existing information in the table. This is best suited for situations with few missing values and where contextual clues can guide the completion.
Advantages: Simple, intuitive, and can leverage specific knowledge about the data.
Disadvantages: Subjective, prone to bias, and not feasible for large datasets with numerous missing values. This method also requires significant domain expertise.
2. Using the Mode (Most Frequent Symbol)
For categorical data represented by symbols, replacing missing values with the mode (most frequently occurring symbol in the column) is a simple imputation method. This approach assumes that the most frequent symbol best represents the likelihood of an unknown value.
Advantages: Simple to implement, computationally inexpensive.
Disadvantages: Can distort the distribution of the data, especially if the mode is significantly more frequent than other symbols. It doesn't account for other contextual information.
3. Conditional Probability and Symbol Relationships
If there are other columns in your table that might be correlated with the symbol column, you can use these to estimate the probability of a missing symbol. For example, if you have a column representing "treatment type" and another column with a symbol indicating "success" or "failure," you can analyze the conditional probability of "success" or "failure" given a specific treatment type. This allows you to fill in missing symbols based on the most likely outcome given the other related information.
Advantages: Leverages relationships between variables for better accuracy.
Disadvantages: Requires understanding of conditional probability and statistical concepts. The accuracy depends heavily on the strength of the correlation between variables.
4. Multiple Imputation Techniques
Multiple imputation is a more sophisticated method that addresses the uncertainty associated with filling in missing data. Instead of replacing each missing value with a single estimate, multiple imputation creates several plausible versions of the dataset, each with different imputed values for the missing symbols. This approach generates multiple completed datasets that can then be analyzed separately, and results are combined to account for the uncertainty introduced by imputation.
Advantages: Considers uncertainty associated with imputation, more statistically robust.
Disadvantages: Computationally intensive, requires specialized statistical software.
5. Machine Learning Approaches
Machine learning algorithms, such as k-Nearest Neighbors (k-NN), can be applied to predict missing symbols. k-NN identifies the k most similar data points (based on other columns in the table) to the data point with the missing symbol, and then uses the symbols of those similar points to estimate the missing symbol. Other algorithms like decision trees or random forests can also be used, depending on the nature of the data and the relationships between variables.
Advantages: Can handle complex relationships between variables, potentially more accurate than simpler methods.
Disadvantages: Requires expertise in machine learning, can be computationally intensive, and model selection is crucial.
Choosing the Right Method: A Practical Guide
The best method for filling in blanks in your symbol column depends on several factors:
- The number of missing values: For a small number of missing values, manual inspection or using the mode might suffice. For a large number, more sophisticated methods like multiple imputation or machine learning are necessary.
- The reason for missing data: If missing data is due to a systematic issue (e.g., a faulty sensor), imputation might introduce bias. Understanding the mechanism of missingness is critical. Missing Completely at Random (MCAR), Missing at Random (MAR), and Missing Not at Random (MNAR) all require different approaches.
- The nature of your data: The type of symbol (categorical, ordinal) and the relationships with other columns influence the choice of method.
- Your analytical goals: The method should align with your goals. If you're interested in descriptive statistics, simpler methods might be sufficient. If inferential statistics are involved, more robust methods are necessary.
- Available resources: Some methods (e.g., multiple imputation, machine learning) require specialized software and expertise.
Addressing Limitations and Potential Biases
It's crucial to acknowledge that any method of imputing missing data introduces some degree of uncertainty and potential bias. The goal is to minimize this bias as much as possible. Always:
- Document your imputation method: Clearly describe the method you used and why you chose it.
- Assess the impact of imputation: Compare results obtained with and without imputation to assess how much the imputation affects your conclusions.
- Consider sensitivity analysis: Try different imputation methods and compare results to assess the robustness of your findings.
- Be transparent about limitations: Acknowledge the limitations of your imputation method in your analysis and interpretation.
Conclusion: Accuracy and Responsible Imputation
Filling in blanks in symbol columns is a crucial aspect of data analysis. Choosing the appropriate method requires careful consideration of several factors. While no imputation method is perfect, understanding the strengths and weaknesses of different approaches allows you to select the most suitable strategy for your specific data and research question. Remember that transparency, careful documentation, and sensitivity analysis are essential to ensure responsible and accurate interpretation of results. Always prioritize methods that minimize bias and acknowledge the limitations inherent in any imputation process. The ultimate goal is to obtain meaningful insights from your data while avoiding misleading conclusions.
Latest Posts
Latest Posts
-
An Attending Physicians Statement Would Be Appropriate
Apr 24, 2025
-
After Recognizing That A Person Is In Cardiac Arrest
Apr 24, 2025
-
How Does A Cancellation Differ From A Disenrollment
Apr 24, 2025
-
Public Schools Charities And Government Agencies Generally
Apr 24, 2025
-
Are Used As A Means For Head And Neck Protection
Apr 24, 2025
Related Post
Thank you for visiting our website which covers about Fill In The Blanks In Symbol Column Of The Table. . We hope the information provided has been useful to you. Feel free to contact us if you have any questions or need further assistance. See you next time and don't miss to bookmark.