Frequency Data Is Useless Without A Timeframe.
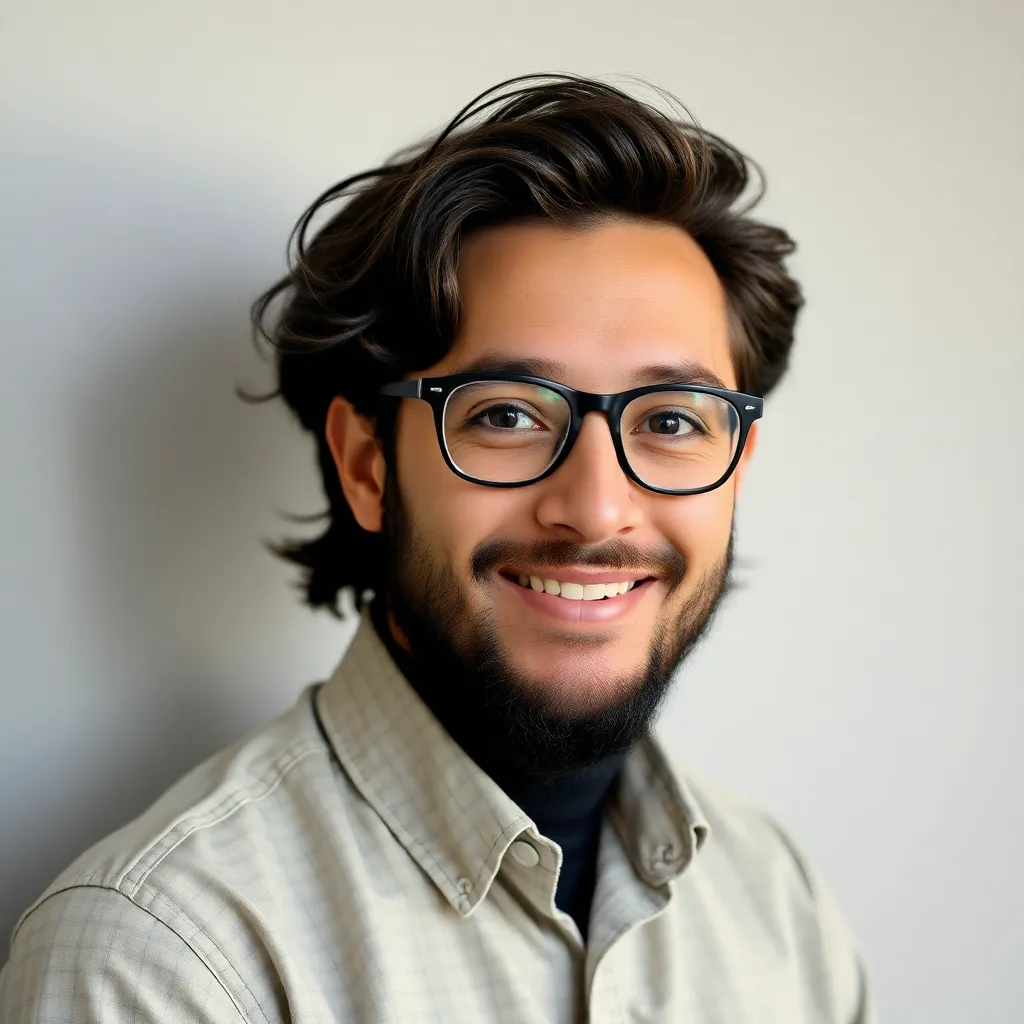
Breaking News Today
May 10, 2025 · 6 min read
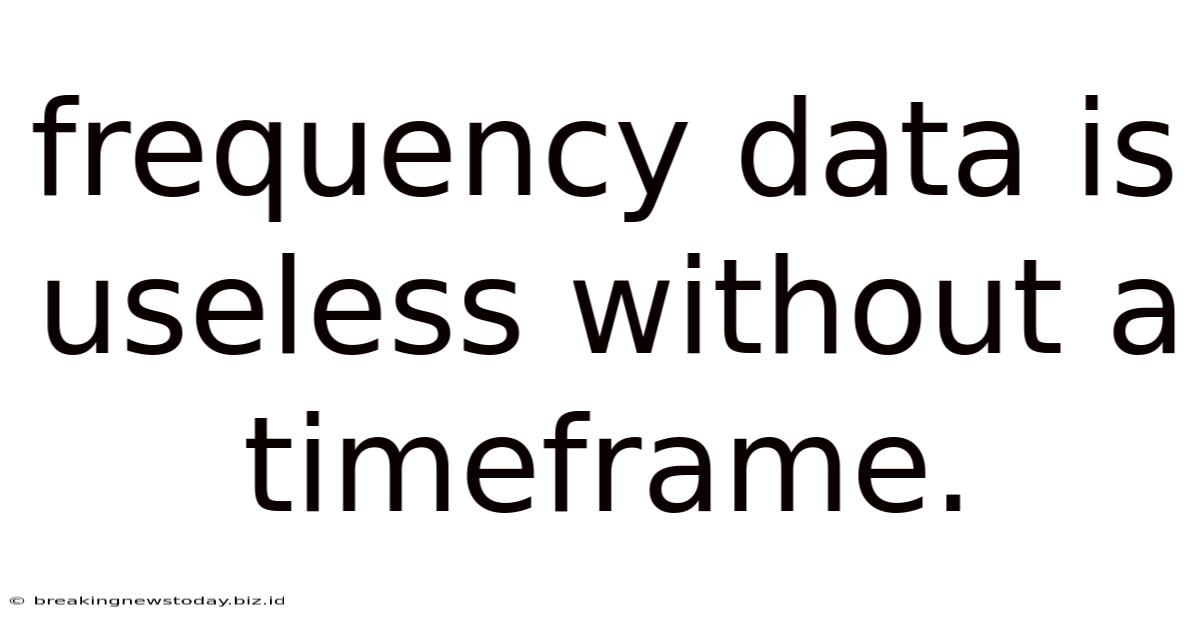
Table of Contents
Frequency Data is Useless Without a Timeframe: Understanding the Crucial Role of Temporal Context
Frequency data, representing the rate of occurrence of an event, is a cornerstone of data analysis across numerous fields. From analyzing website traffic to understanding disease outbreaks, frequency counts provide valuable insights. However, frequency data devoid of a timeframe is fundamentally useless. It's like having a snapshot of a moving car – you see where it is, but you have no idea where it's going or how fast it's moving. Without the temporal dimension, the data loses its context, rendering it incapable of revealing meaningful trends, patterns, or predictions.
The Significance of Temporal Context
The true power of frequency data lies in its ability to illustrate change over time. A simple count of occurrences might indicate a high frequency, but without knowing the time period over which those occurrences happened, the number is meaningless. Consider these examples:
-
Website traffic: Knowing that a website received 10,000 visits is only half the story. Was this in a day, a week, or a year? The timeframe drastically alters the interpretation of the data. 10,000 visits in a day suggests a highly popular site, while 10,000 visits over a year indicates significantly lower traffic.
-
Sales figures: A company reporting 1 million dollars in sales sounds impressive. But if that was achieved over a decade, it's far less impressive than achieving the same figure in a single quarter. The timeframe directly impacts the assessment of the company's performance and growth.
-
Public health emergencies: Tracking the number of confirmed cases of a disease is critical. However, understanding the rate of increase or decrease over days, weeks, or months is crucial for implementing effective control measures. A sudden surge in cases over a short period necessitates urgent action, while a slow, steady increase might allow for a more measured response.
-
Social media analytics: The number of likes, shares, or comments on a post provides a superficial view of engagement. Knowing the time elapsed since posting allows a more nuanced understanding of engagement trends—whether it’s trending upward, plateauing, or declining.
Types of Timeframes and Their Implications
The choice of timeframe significantly influences the analysis and interpretation of frequency data. Different timeframes reveal different patterns:
1. Short-Term Timeframes (e.g., hourly, daily, weekly)
These are ideal for capturing short-term fluctuations and trends. For example, monitoring website traffic on an hourly basis might reveal peak usage times, allowing for optimized resource allocation. Daily sales figures can highlight daily sales patterns, potentially influenced by external factors like weather or promotional campaigns. Analyzing weekly data can reveal weekly cycles, allowing businesses to plan accordingly.
2. Medium-Term Timeframes (e.g., monthly, quarterly, yearly)
These timeframes are suitable for identifying seasonal trends and longer-term growth patterns. Monthly sales data might reveal seasonal peaks and dips, while quarterly earnings reports provide insights into a company's financial performance over a longer period. Analyzing yearly data can reveal broader trends in market growth or economic conditions.
3. Long-Term Timeframes (e.g., decadal, multi-decadal)
These are useful for understanding long-term historical trends and patterns. Analyzing climate data over decades reveals long-term changes in temperature and precipitation, while studying population growth over multiple decades provides insights into demographic shifts.
Analyzing Frequency Data with Timeframes: Techniques and Tools
Effectively analyzing frequency data requires selecting appropriate timeframes and employing suitable analytical techniques. This involves:
-
Data aggregation: Combining data points within specific time intervals to create summary statistics. For example, aggregating hourly website visits into daily totals or weekly averages.
-
Data visualization: Employing charts and graphs to represent frequency data over time. Line graphs are particularly effective for showing trends, while bar charts are suitable for comparing frequencies across different time periods.
-
Time series analysis: A specialized statistical technique used to analyze data points collected over time. It helps identify patterns, seasonality, and trends within the data. Techniques like moving averages, exponential smoothing, and ARIMA models are commonly employed.
-
Statistical process control (SPC): Used to monitor processes over time and identify deviations from expected patterns. Control charts help visualize data fluctuations and identify potential issues.
Many tools can facilitate this analysis. Popular options include:
-
Spreadsheet software (Excel, Google Sheets): Suitable for basic data aggregation and visualization.
-
Statistical software (R, SPSS, SAS): Powerful tools for advanced time series analysis and statistical modeling.
-
Data visualization tools (Tableau, Power BI): Enable the creation of interactive and insightful visualizations of frequency data over time.
The Importance of Data Quality and Completeness
Accurate and complete data are crucial for reliable analysis. Missing data or inconsistencies in the timeframe can lead to flawed interpretations. Careful data cleaning and preprocessing are necessary to ensure data quality and reliability. This involves:
-
Identifying and handling missing data: Appropriate methods for dealing with missing data include imputation (estimating missing values) or exclusion (removing data points with missing values).
-
Addressing outliers: Outliers, or unusual data points, can skew the analysis. Identifying and handling outliers appropriately is crucial for obtaining reliable results.
-
Data consistency: Ensuring consistent data formats and units of measurement across the dataset is crucial for accurate analysis.
Practical Applications Across Diverse Fields
The integration of timeframes with frequency data significantly improves analytical capabilities across many fields:
1. Business Analytics:
- Sales forecasting: Analyzing past sales data with timeframes helps predict future sales.
- Marketing campaign effectiveness: Tracking website traffic and conversions over time assesses campaign performance.
- Inventory management: Analyzing sales frequency over time optimizes inventory levels.
2. Healthcare:
- Disease surveillance: Monitoring disease outbreaks using frequency data with timeframes is vital for public health responses.
- Patient monitoring: Tracking vital signs over time aids in early detection of health issues.
- Clinical trial analysis: Analyzing treatment efficacy over time assesses clinical outcomes.
3. Finance:
- Risk management: Analyzing market volatility over time helps manage financial risks.
- Investment strategies: Tracking investment performance over time informs investment decisions.
- Fraud detection: Monitoring financial transactions over time identifies suspicious activity.
4. Environmental Science:
- Climate change analysis: Analyzing climate data over time reveals long-term trends.
- Pollution monitoring: Tracking pollution levels over time identifies pollution sources.
- Biodiversity monitoring: Tracking species populations over time assesses conservation efforts.
5. Social Sciences:
- Public opinion polling: Analyzing opinion polls over time reveals shifts in public sentiment.
- Crime analysis: Tracking crime rates over time helps identify crime hotspots and patterns.
- Social media sentiment analysis: Monitoring social media activity over time reveals changing public attitudes.
Conclusion: Time is of the Essence in Data Analysis
In summary, frequency data is utterly meaningless without a defined timeframe. The temporal context provides the necessary dimension for understanding trends, patterns, and changes. By choosing appropriate timeframes, employing effective analytical techniques, and ensuring data quality, we unlock the true potential of frequency data for insightful analysis and informed decision-making across numerous disciplines. Neglecting the temporal aspect renders frequency data a mere collection of numbers, devoid of its inherent power to inform and illuminate. Therefore, incorporating time as an integral part of data analysis is not just advisable but absolutely essential for extracting meaningful conclusions and making accurate predictions.
Latest Posts
Latest Posts
-
Workplace Violence Is Defined As Threats Physical Assaults Muggings And
May 10, 2025
-
Two Lines Intersecting At A Right Angle
May 10, 2025
-
You Must Encrypt Files With Any Of These Extensions
May 10, 2025
-
What Kind Of Conflict Is Typical Of Multicultural Literature
May 10, 2025
-
Is A Measure Of The Energy In A Wave
May 10, 2025
Related Post
Thank you for visiting our website which covers about Frequency Data Is Useless Without A Timeframe. . We hope the information provided has been useful to you. Feel free to contact us if you have any questions or need further assistance. See you next time and don't miss to bookmark.