Gathering And Analyzing Data Is Part Of This Phase.
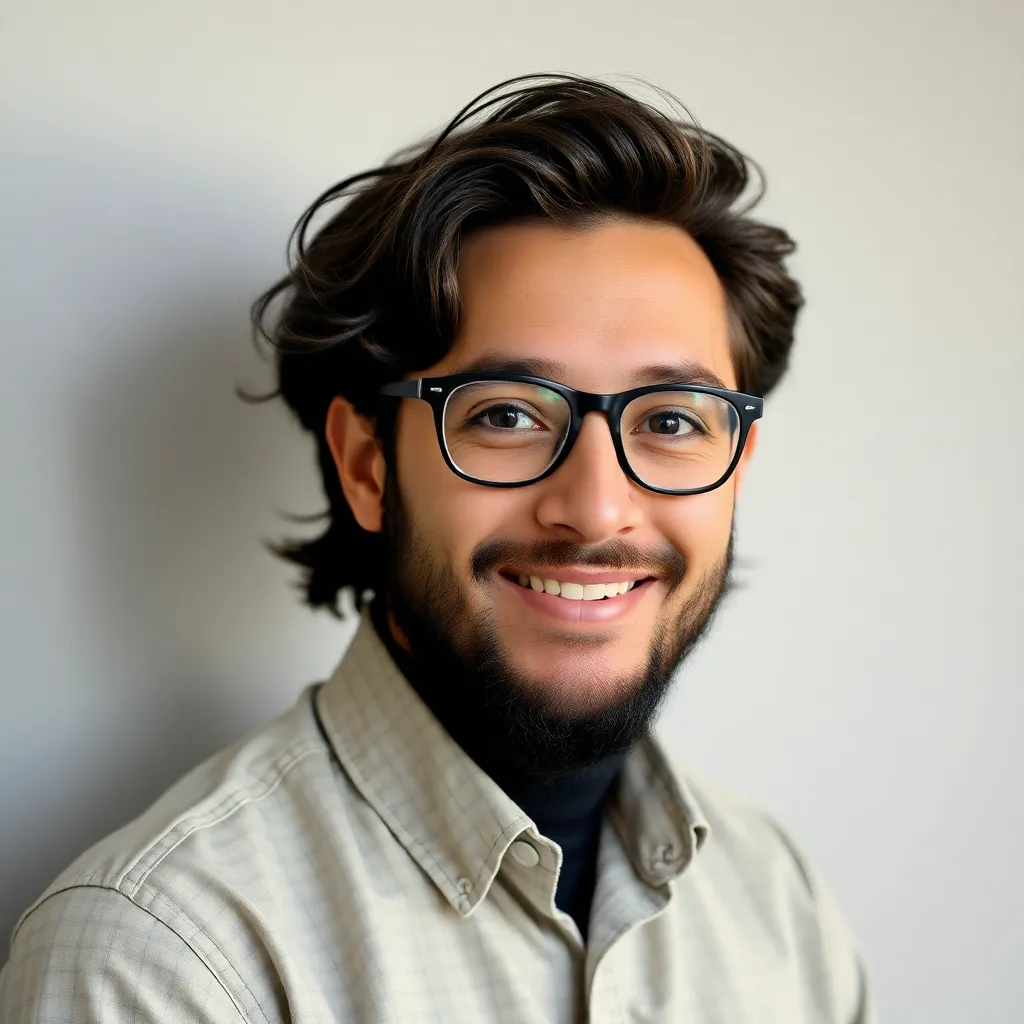
Breaking News Today
May 10, 2025 · 6 min read
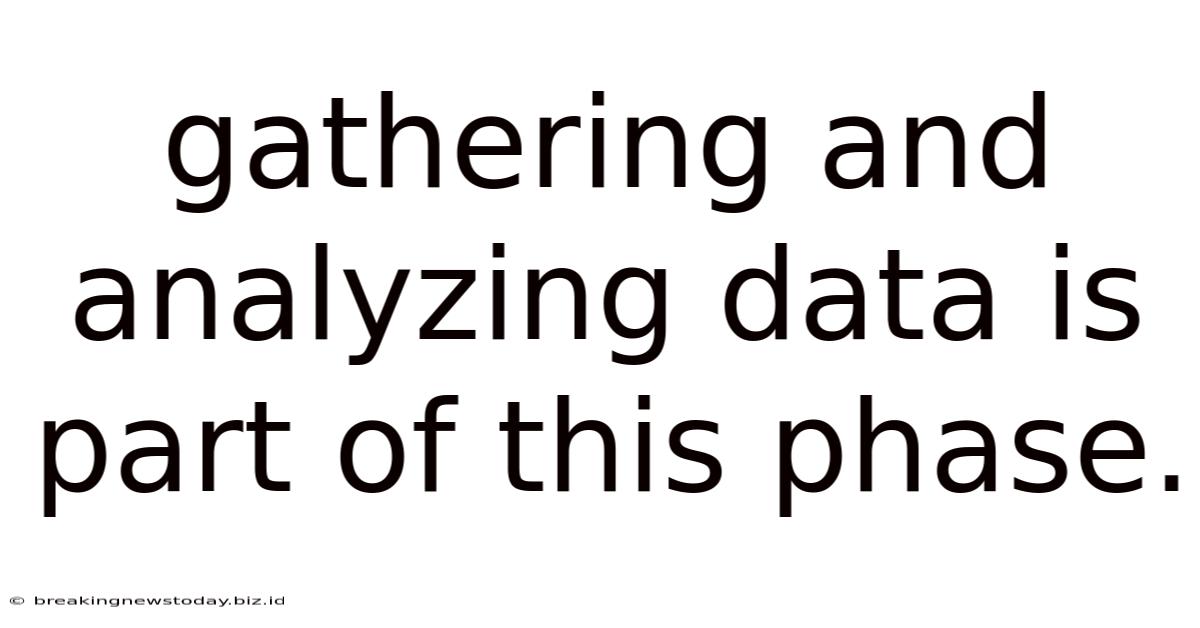
Table of Contents
Gathering and Analyzing Data: A Critical Phase in Any Successful Project
Gathering and analyzing data forms a crucial cornerstone in numerous processes, impacting everything from scientific research and business strategy to social policy and personal decision-making. This phase isn't merely a step; it's the bedrock upon which informed choices are built, efficient strategies are devised, and impactful results are achieved. This comprehensive guide explores the multifaceted nature of data gathering and analysis, encompassing its various stages, associated challenges, and best practices to ensure effective outcomes.
Understanding the Importance of Data in Decision-Making
In today's data-driven world, the ability to effectively collect and analyze data is no longer a luxury, but a necessity. Decisions made without the support of robust data analysis are often speculative, inefficient, and potentially detrimental. By contrast, data-driven decision-making empowers individuals and organizations to:
1. Identify Trends and Patterns:
Data analysis reveals hidden trends and patterns that might be invisible to the naked eye. This insight allows for proactive planning and adaptation to changing circumstances, enabling organizations to stay ahead of the curve.
2. Optimize Processes and Resources:
Analyzing data about operational efficiency, resource allocation, and customer behavior can highlight areas for improvement. This leads to streamlined processes, reduced waste, and optimized resource utilization, boosting overall productivity and profitability.
3. Improve Customer Understanding:
Gathering and analyzing customer data—preferences, purchasing habits, feedback—provides invaluable insights into their needs and expectations. This knowledge is fundamental to developing targeted marketing campaigns, improving products and services, and fostering strong customer relationships.
4. Reduce Risk and Uncertainty:
Data analysis helps quantify risks and uncertainties associated with various decisions. This enables more informed risk management strategies, mitigating potential negative outcomes and maximizing opportunities for success.
5. Enhance Innovation and Creativity:
Data can inspire innovation by revealing untapped market opportunities, unmet needs, and new potential applications for existing products or services. By combining data-driven insights with creative thinking, organizations can develop truly innovative solutions.
The Stages of Data Gathering and Analysis
The process of data gathering and analysis is typically divided into several distinct stages:
1. Defining Objectives and Research Questions:
Before embarking on data collection, it is crucial to clearly define the objectives of the analysis and formulate specific research questions. This ensures that the data gathered is relevant, focused, and directly addresses the problem at hand. A poorly defined objective can lead to wasted resources and inconclusive results. Clearly articulated research questions guide every subsequent step.
2. Data Collection Methods:
The choice of data collection method depends heavily on the research questions and the nature of the data being sought. Common methods include:
-
Surveys: These can be administered online, via mail, or in person, and are useful for gathering quantitative and qualitative data on attitudes, behaviors, and opinions. Careful design is crucial to avoid bias.
-
Interviews: Interviews, structured or unstructured, allow for in-depth exploration of topics, offering valuable qualitative data. They are particularly useful for understanding complex issues or exploring nuanced perspectives.
-
Observations: Systematic observation of behaviors or phenomena in natural settings can provide rich data, particularly when studying human interaction or environmental processes.
-
Experiments: Controlled experiments allow researchers to manipulate variables and observe their effects, establishing cause-and-effect relationships. They are powerful but require careful design and execution.
-
Existing Data Sources: Leveraging existing datasets (e.g., government statistics, company records, online databases) can be a cost-effective and time-saving approach, but data quality and relevance must be carefully assessed.
3. Data Cleaning and Preparation:
Raw data is rarely ready for analysis. This stage involves cleaning the data to ensure accuracy, consistency, and completeness. This includes:
-
Handling Missing Values: Deciding how to manage missing data points is crucial to prevent bias. Techniques range from simple deletion to sophisticated imputation methods.
-
Identifying and Correcting Errors: Errors can arise from various sources, including data entry mistakes and inconsistencies in measurement. Careful review and correction are essential.
-
Data Transformation: Data often needs to be transformed to facilitate analysis. This can involve recoding variables, creating new variables, or standardizing data formats.
4. Data Analysis:
This stage involves employing appropriate statistical or analytical techniques to extract meaningful insights from the data. The choice of method depends on the type of data and the research questions. Common techniques include:
-
Descriptive Statistics: Summarizing data using measures like mean, median, mode, and standard deviation provides a basic understanding of data distribution.
-
Inferential Statistics: Inferential statistics enables drawing conclusions about a population based on a sample of data. Techniques like t-tests, ANOVA, and regression analysis are widely used.
-
Data Visualization: Visualizing data using charts, graphs, and other visual aids helps to communicate findings effectively and identify patterns that might be missed in numerical analysis.
-
Machine Learning: Advanced techniques like machine learning can uncover complex patterns and relationships in large datasets, particularly useful for predictive modeling and pattern recognition.
5. Interpretation and Reporting:
The final stage involves interpreting the findings in the context of the research questions and objectives. The results should be clearly and concisely communicated through reports, presentations, or other appropriate formats. Effective communication is crucial to ensuring that the insights derived from data analysis are effectively utilized.
Challenges in Data Gathering and Analysis
The process of data gathering and analysis is not without its challenges:
1. Data Quality Issues:
Inaccurate, incomplete, or inconsistent data can lead to flawed conclusions. Maintaining data quality throughout the entire process is paramount.
2. Data Bias:
Bias can arise from various sources, including sampling bias, measurement bias, and response bias. Careful planning and rigorous methodology are needed to minimize bias.
3. Data Security and Privacy:
Protecting the privacy and security of sensitive data is critical, particularly when dealing with personal or confidential information. Appropriate measures must be taken to ensure compliance with relevant regulations.
4. Data Volume and Complexity:
The sheer volume and complexity of data can pose significant challenges, requiring specialized tools and expertise to manage and analyze effectively.
5. Interpretation and Communication:
Interpreting data and communicating findings effectively requires strong analytical skills and clear communication abilities. Misinterpretation or poor communication can lead to incorrect conclusions or ineffective decision-making.
Best Practices for Effective Data Gathering and Analysis
To maximize the effectiveness of data gathering and analysis, consider these best practices:
-
Clear Research Design: A well-defined research design is crucial for ensuring that the data collected is relevant and reliable.
-
Robust Data Collection Methods: Choosing appropriate data collection methods ensures data quality and minimizes bias.
-
Thorough Data Cleaning: Careful data cleaning is essential to prevent errors from propagating through the analysis.
-
Appropriate Analytical Techniques: Selecting appropriate analytical techniques ensures that the data is analyzed correctly and meaningful insights are extracted.
-
Effective Data Visualization: Visualizing data facilitates understanding and communication of findings.
-
Ethical Considerations: Adhering to ethical guidelines ensures responsible data handling and protects individual privacy.
-
Collaboration and Expertise: Involving individuals with relevant expertise can enhance the quality and effectiveness of data gathering and analysis.
Conclusion: Data – The Engine of Informed Decisions
Gathering and analyzing data is an iterative process, requiring careful planning, rigorous execution, and a keen eye for detail. While challenges exist, the rewards of data-driven decision-making are significant, leading to more informed choices, improved efficiency, reduced risk, and enhanced innovation. By mastering the techniques and best practices outlined above, individuals and organizations can harness the power of data to achieve their objectives and drive impactful outcomes. The future belongs to those who can effectively collect, analyze, and act upon data – making it the true engine of progress and informed decisions in every facet of life.
Latest Posts
Latest Posts
-
A Nurse Is Reviewing Protocol In Preparation For Suctioning Secretions
May 11, 2025
-
The Climate And Geography Of Italy Has Led To More
May 11, 2025
-
If Then Statements Are Designed To Prevent Us From Making
May 11, 2025
-
What Are 3 Things Used To Make Glucose In Photosynthesis
May 11, 2025
-
After A Hurricane Warning Came Across The Television
May 11, 2025
Related Post
Thank you for visiting our website which covers about Gathering And Analyzing Data Is Part Of This Phase. . We hope the information provided has been useful to you. Feel free to contact us if you have any questions or need further assistance. See you next time and don't miss to bookmark.