Help Economists Make Forecasts Which Are Also Called Predictions
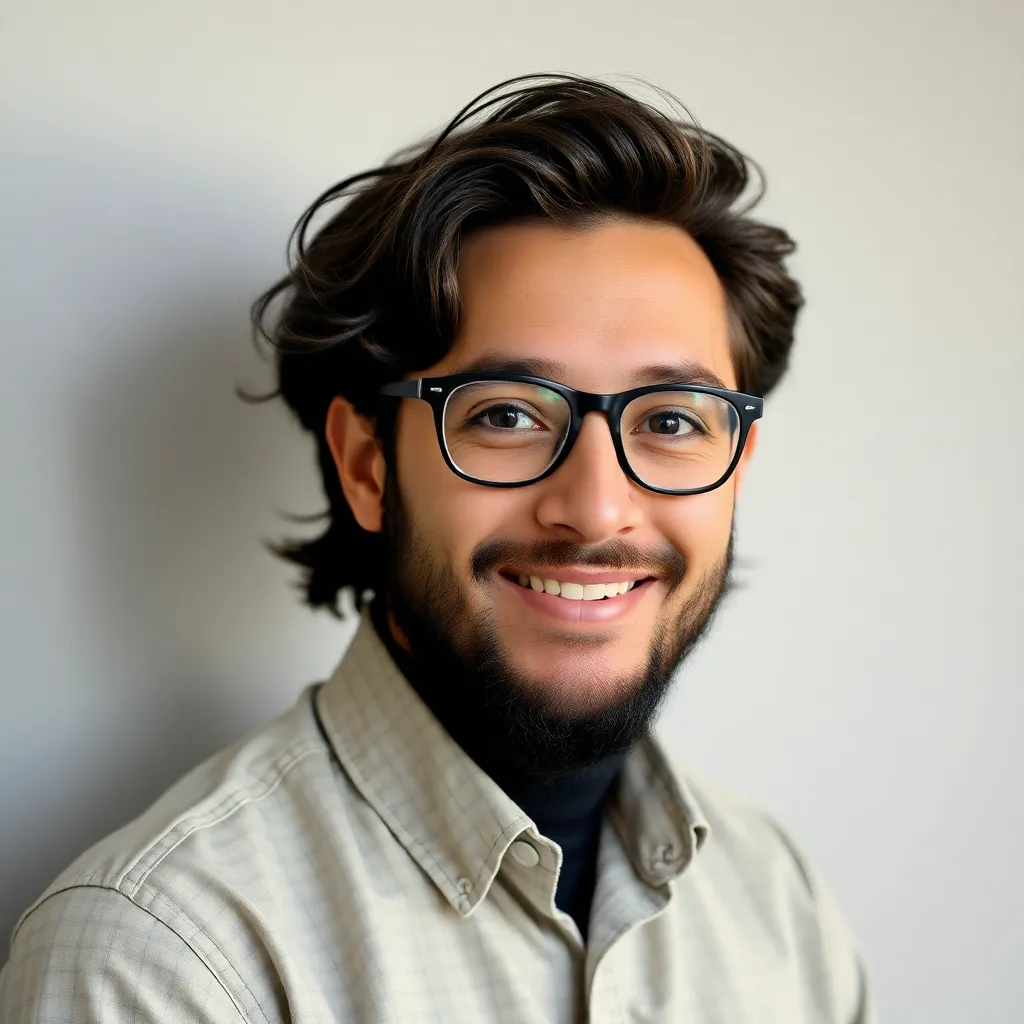
Breaking News Today
May 10, 2025 · 6 min read
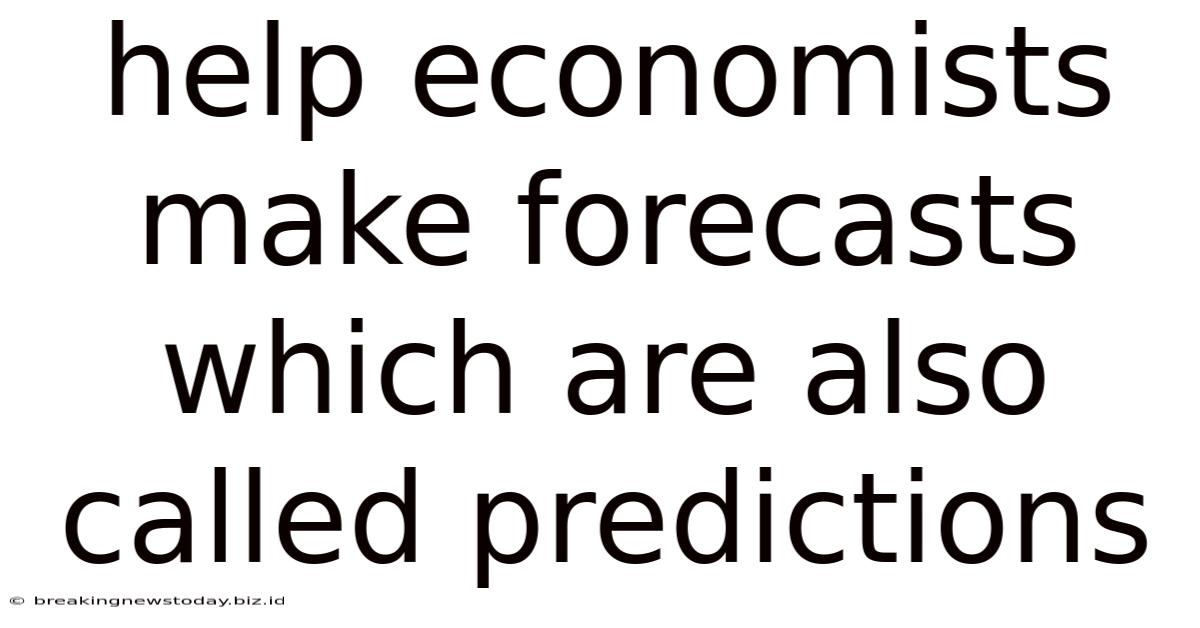
Table of Contents
Helping Economists Make Accurate Forecasts: A Deep Dive into Predictive Modeling
Economic forecasting, or prediction, is the process of estimating the future state of the economy. It's a complex undertaking, crucial for policymakers, businesses, and individuals alike. While perfectly accurate predictions are impossible, advancements in econometrics, data science, and machine learning are continually improving the accuracy and reliability of economic forecasts. This article delves into the multifaceted world of economic forecasting, exploring the challenges, methodologies, and crucial elements for generating more precise and insightful predictions.
The Challenges of Economic Forecasting
Economic systems are inherently complex and dynamic. Numerous intertwined factors – from consumer confidence and technological innovation to geopolitical events and natural disasters – influence economic outcomes. Accurately predicting the interplay of these variables is a monumental task. Here are some of the key challenges:
1. Unpredictability of Human Behavior:
Economic models rely heavily on assumptions about human behavior. However, human actions are often irrational, driven by emotions, and influenced by unforeseen circumstances. Predicting consumer spending, investment decisions, and market sentiment with absolute precision is nearly impossible. Behavioral economics, which acknowledges the limitations of rational actor models, offers valuable insights but still struggles to fully capture the complexity of human decision-making.
2. Data Limitations and Measurement Errors:
Economic data is often incomplete, inaccurate, or subject to revision. Gathering reliable data, especially on informal economies or in developing countries, is a significant hurdle. Moreover, the very act of measuring economic activity can distort the data itself. For example, changes in accounting standards can impact reported GDP figures, creating inconsistencies over time.
3. Structural Breaks and Regime Changes:
Economic systems are not static. Technological disruptions, policy shifts, and unforeseen events (e.g., pandemics, wars) can lead to "structural breaks" – sudden shifts in the underlying relationships between economic variables. Models calibrated on historical data may become inaccurate when these breaks occur. Identifying and adapting to these regime changes is crucial for maintaining forecast accuracy.
4. Model Uncertainty and Specification Errors:
Economists use various models – from simple regression analysis to sophisticated dynamic stochastic general equilibrium (DSGE) models – to generate forecasts. Each model incorporates specific assumptions and simplifications, introducing inherent uncertainty. The choice of model and its specification can significantly influence the forecast results. Moreover, the limitations of even the most advanced models can lead to significant specification errors.
5. Feedback Loops and Self-Fulfilling Prophecies:
Forecasts themselves can influence the very outcomes they predict. For instance, a widely publicized forecast of a recession can trigger a decline in consumer confidence, leading to reduced spending and potentially fulfilling the initial prediction. This creates a feedback loop that complicates the forecasting process.
Methodologies for Economic Forecasting
Despite the challenges, numerous techniques are employed to generate economic forecasts. These methodologies vary in complexity, data requirements, and the type of predictions they generate.
1. Time Series Analysis:
This approach focuses on analyzing historical data to identify patterns and trends, extrapolating them into the future. Techniques like ARIMA (Autoregressive Integrated Moving Average) models and exponential smoothing are commonly used. While relatively simple to implement, time series models often struggle to capture structural breaks and the impact of external shocks.
2. Econometric Modeling:
Econometric models utilize statistical techniques to establish relationships between different economic variables. These models often incorporate economic theory and are designed to test specific hypotheses. Examples include vector autoregression (VAR) models and cointegration analysis. Econometric models offer a more nuanced understanding of economic relationships but require careful model specification and robust data.
3. Agent-Based Modeling (ABM):
ABM simulates the interactions of individual economic agents (consumers, firms, etc.) to generate aggregate outcomes. This approach is particularly useful in capturing emergent behaviors and complex interactions that might be missed by traditional econometric methods. However, ABM can be computationally intensive and require detailed data on agent behavior.
4. Machine Learning Techniques:
Machine learning algorithms, such as neural networks, support vector machines, and random forests, are increasingly used in economic forecasting. These techniques excel at identifying complex patterns in large datasets and can adapt to changing economic conditions. However, interpreting the results of machine learning models can be challenging, and the "black box" nature of some algorithms raises concerns about transparency and explainability.
5. Bayesian Methods:
Bayesian methods incorporate prior information and uncertainty into the forecasting process. This allows economists to combine historical data with expert knowledge and subjective judgments to generate more informed predictions. Bayesian approaches are particularly useful in situations with limited data or high uncertainty.
Enhancing Forecast Accuracy: Key Considerations
Improving the accuracy of economic forecasts requires a multifaceted approach focusing on data quality, model selection, and incorporating a wider range of information.
1. Data Quality and Preprocessing:
Using high-quality, reliable data is paramount. Careful data cleaning, preprocessing, and handling of missing values are essential. Exploring alternative data sources, such as social media sentiment, satellite imagery, and mobile phone data, can supplement traditional economic indicators and provide a more comprehensive picture.
2. Model Selection and Validation:
Choosing the appropriate model is crucial. The complexity of the model should be commensurate with the available data and the specific forecasting task. Rigorous model validation, including out-of-sample testing and sensitivity analysis, helps assess the robustness of the forecast and identify potential weaknesses.
3. Incorporating Qualitative Information:
Quantitative data alone is insufficient for accurate economic forecasting. Incorporating qualitative information, such as expert opinions, policy announcements, and geopolitical events, can significantly improve forecast accuracy. This can be achieved through expert elicitation techniques or by incorporating narrative information into quantitative models.
4. Scenario Planning and Stress Testing:
Generating forecasts under different scenarios helps assess the sensitivity of the predictions to various assumptions and shocks. Stress testing, which involves simulating extreme events, helps identify potential vulnerabilities and inform risk management strategies.
5. Continuous Monitoring and Model Updating:
Economic forecasts are not static. Regularly monitoring the accuracy of forecasts and updating models in light of new information is crucial. This iterative process, known as model recalibration, allows for continuous improvement and adaptation to changing economic conditions.
The Role of Transparency and Communication
Transparency in the forecasting process is essential for building trust and fostering effective communication. Clearly articulating the underlying assumptions, limitations, and uncertainties associated with the forecasts is critical. Communicating the forecasts effectively to a diverse audience, using clear and accessible language, is crucial for their practical application.
Conclusion
Economic forecasting remains a challenging but indispensable endeavor. While perfect prediction is unattainable, advancements in econometrics, data science, and machine learning, along with a commitment to rigorous methodology and transparent communication, are continually improving the accuracy and reliability of economic forecasts. By addressing the challenges, embracing innovative techniques, and fostering collaboration between economists, data scientists, and policymakers, we can move closer to generating more insightful and impactful economic predictions. The journey towards more accurate economic forecasting is an ongoing process of refinement and innovation, driven by the need for a deeper understanding of the complexities of our economic systems.
Latest Posts
Latest Posts
-
Which Statement Best Describes The Viewpoint Expressed In The Passage
May 10, 2025
-
Human Growth Hormone Supplements Is Shown To Quizlet
May 10, 2025
-
What Are The Three Main Weapons Of Predators
May 10, 2025
-
What Is The Minimum Hot Holding Temperature Requirement For Hot Dogs
May 10, 2025
-
In An Atom Where Are The Electrons Located
May 10, 2025
Related Post
Thank you for visiting our website which covers about Help Economists Make Forecasts Which Are Also Called Predictions . We hope the information provided has been useful to you. Feel free to contact us if you have any questions or need further assistance. See you next time and don't miss to bookmark.