How Do Insurers Predict The Increase Of Individual Risks
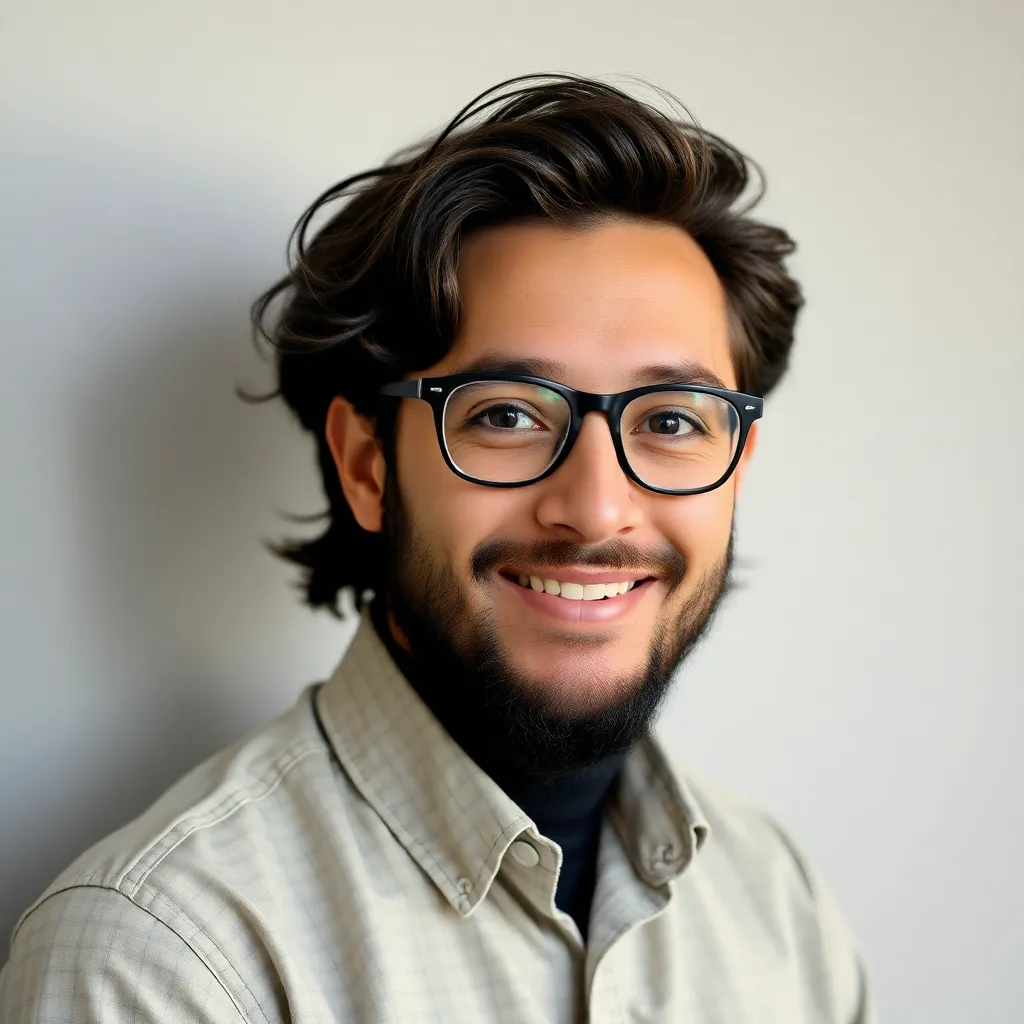
Breaking News Today
Apr 25, 2025 · 6 min read

Table of Contents
How Do Insurers Predict the Increase of Individual Risks?
Insurance companies are in the business of managing risk. To do this effectively, they need to accurately predict the likelihood of future claims. This isn't about predicting individual events with certainty (will this person have a car accident next week?), but rather assessing the probability of events occurring within a large pool of insured individuals. Predicting the increase in individual risks is a crucial aspect of this, allowing insurers to adjust premiums, underwriting policies, and risk management strategies proactively. This involves a complex interplay of data analysis, statistical modeling, and actuarial expertise.
The Foundation: Data is King
The cornerstone of risk prediction is data. Insurers gather vast amounts of information from a variety of sources, both internal and external. The more comprehensive and accurate this data is, the better the predictions will be. Here's a breakdown of key data sources:
1. Internal Data: The Heart of the Operation
-
Claims Data: This is arguably the most valuable data source. It provides a historical record of past claims, including the type of claim, the amount paid, the circumstances surrounding the claim, and the characteristics of the individual who made the claim (age, location, occupation, etc.). Analyzing this data reveals patterns and trends that are crucial for predicting future claims. For example, a spike in claims related to a specific type of injury in a particular geographic area might indicate an emerging risk.
-
Policyholder Data: This includes demographic information (age, gender, marital status, address), driving history (for auto insurance), medical history (for health insurance), and policy details (coverage type, deductible, premium paid). This data allows insurers to segment their customer base into risk groups with similar characteristics.
-
Underwriting Data: This encompasses the information collected during the underwriting process, including credit scores, driving records, and medical examinations. This data helps insurers assess the risk profile of each individual applicant before issuing a policy.
2. External Data: Expanding the Scope
-
Geographic Data: Data on weather patterns, crime rates, natural disaster frequency, and socioeconomic factors in specific geographic locations helps insurers assess the risk associated with different areas. Areas prone to flooding, earthquakes, or high crime rates will have higher insurance premiums.
-
Economic Data: Economic indicators such as inflation, unemployment rates, and interest rates can significantly impact claim frequency and severity. Economic downturns, for example, can lead to an increase in claims related to financial hardship.
-
Medical Data and Research: For health insurance, access to broader medical data and research on emerging health trends allows for improved risk assessment. This data helps predict the rise in certain diseases or conditions, leading to more accurate risk scoring and premium adjustments.
-
Social Media and Public Data: While still nascent in its application, data from social media and other publicly available sources can offer insights into individual behaviors and trends. However, ethical considerations and data privacy regulations must be strictly adhered to.
Analyzing the Data: Statistical Modeling and Machine Learning
Raw data alone is not enough. Insurers employ sophisticated statistical models and machine learning algorithms to analyze the data and predict future risk increases.
1. Traditional Statistical Modeling: Established Methods
-
Regression Analysis: This is a widely used statistical technique to model the relationship between dependent variables (e.g., claim frequency) and independent variables (e.g., age, location, driving history). By identifying significant relationships, insurers can predict how changes in independent variables might affect claim frequency.
-
Time Series Analysis: This method is used to analyze data collected over time to identify trends and seasonality. For example, analyzing past claims data over several years can reveal seasonal patterns in claims (e.g., more car accidents during holidays).
-
Survival Analysis: This technique is particularly useful for analyzing the duration of insurance policies before a claim occurs. This helps predict the likelihood of claims occurring within specific timeframes.
2. Machine Learning: Advanced Predictive Capabilities
Machine learning algorithms excel at identifying complex patterns and relationships in large datasets that might be missed by traditional statistical methods. Several techniques are commonly used:
-
Decision Trees and Random Forests: These algorithms create a tree-like structure to classify individuals into different risk categories based on their characteristics. Random forests combine multiple decision trees to improve prediction accuracy.
-
Gradient Boosting Machines (GBM): GBM algorithms build a sequence of decision trees, where each tree corrects the errors of the previous ones. This leads to highly accurate predictions, but requires careful tuning to prevent overfitting.
-
Neural Networks: These complex algorithms are capable of learning intricate relationships in data and can achieve high prediction accuracy, but require significant computational resources and careful training.
Identifying and Interpreting Increases in Risk
Once the data has been analyzed, the next step is to identify and interpret increases in risk. This involves comparing current data to historical trends, identifying significant deviations, and assessing potential contributing factors. This could involve:
-
Trend Analysis: Examining the change in claim frequency or severity over time to detect upward trends.
-
Cohort Analysis: Comparing the risk profiles of different groups of policyholders (e.g., age groups, geographic locations) to see if specific groups are experiencing a disproportionate increase in risk.
-
Scenario Planning: Modeling the potential impact of various future scenarios (e.g., changes in weather patterns, economic downturns) on claim frequency and severity.
Responding to Predicted Increases in Risk
Identifying an increase in risk is only the first step. Insurers must then develop strategies to mitigate the increased risk. These strategies can include:
-
Premium Adjustments: Increasing premiums for policyholders in higher-risk categories.
-
Underwriting Changes: Tightening underwriting criteria to reduce the number of high-risk individuals accepted for insurance.
-
Risk Mitigation Programs: Implementing programs to encourage policyholders to adopt safer behaviors (e.g., driver safety courses, health and wellness programs).
-
Reinsurance: Transferring some of the risk to reinsurers to reduce the insurer's potential losses.
-
Product Development: Developing new insurance products that cater to specific risk profiles or that offer innovative risk management solutions.
Challenges and Ethical Considerations
Predicting the increase of individual risks is not without its challenges. Insurers must grapple with:
-
Data Quality: The accuracy of predictions depends heavily on the quality of the data. Incomplete, inaccurate, or biased data can lead to flawed predictions.
-
Data Privacy: Collecting and using personal data raises ethical and legal concerns. Insurers must comply with data privacy regulations and ensure the ethical handling of sensitive information.
-
Model Explainability: Some machine learning models are "black boxes," making it difficult to understand how they arrive at their predictions. This lack of transparency can make it challenging to identify biases or errors.
-
Bias and Discrimination: AI models can inherit and amplify biases present in the data they are trained on. This can lead to unfair or discriminatory outcomes, such as charging higher premiums to certain demographic groups without proper justification.
The Future of Risk Prediction
The field of risk prediction is constantly evolving. Advances in technology, particularly in areas such as big data analytics, machine learning, and the Internet of Things (IoT), are enabling insurers to collect and analyze even more data, leading to more accurate and sophisticated predictions. The use of telematics, wearable technology, and other data-collecting devices provides insurers with real-time information on the behavior of their policyholders, enabling more dynamic risk assessment and personalized pricing. However, responsible data usage and algorithmic fairness remain paramount to ensure ethical and equitable outcomes. The future of insurance hinges on effectively leveraging these advancements while mitigating potential biases and upholding data privacy rights. The ultimate goal remains to accurately assess and manage risk, while offering fair and affordable insurance products to a wide range of individuals.
Latest Posts
Latest Posts
-
A Newborn Has Received Face Mask Ventilation But Is Not Improving
Apr 25, 2025
-
How Long Must Paperwork From Residue Turn In Be Kept
Apr 25, 2025
-
Who Has The Right Of Way At An Uncontrolled Intersection
Apr 25, 2025
-
Classify These Molecules As Polar Or Nonpolar
Apr 25, 2025
-
It Is Unusual For A Company To Sell
Apr 25, 2025
Related Post
Thank you for visiting our website which covers about How Do Insurers Predict The Increase Of Individual Risks . We hope the information provided has been useful to you. Feel free to contact us if you have any questions or need further assistance. See you next time and don't miss to bookmark.