How Does A Good Experimental Conclusion Differ From An Inference
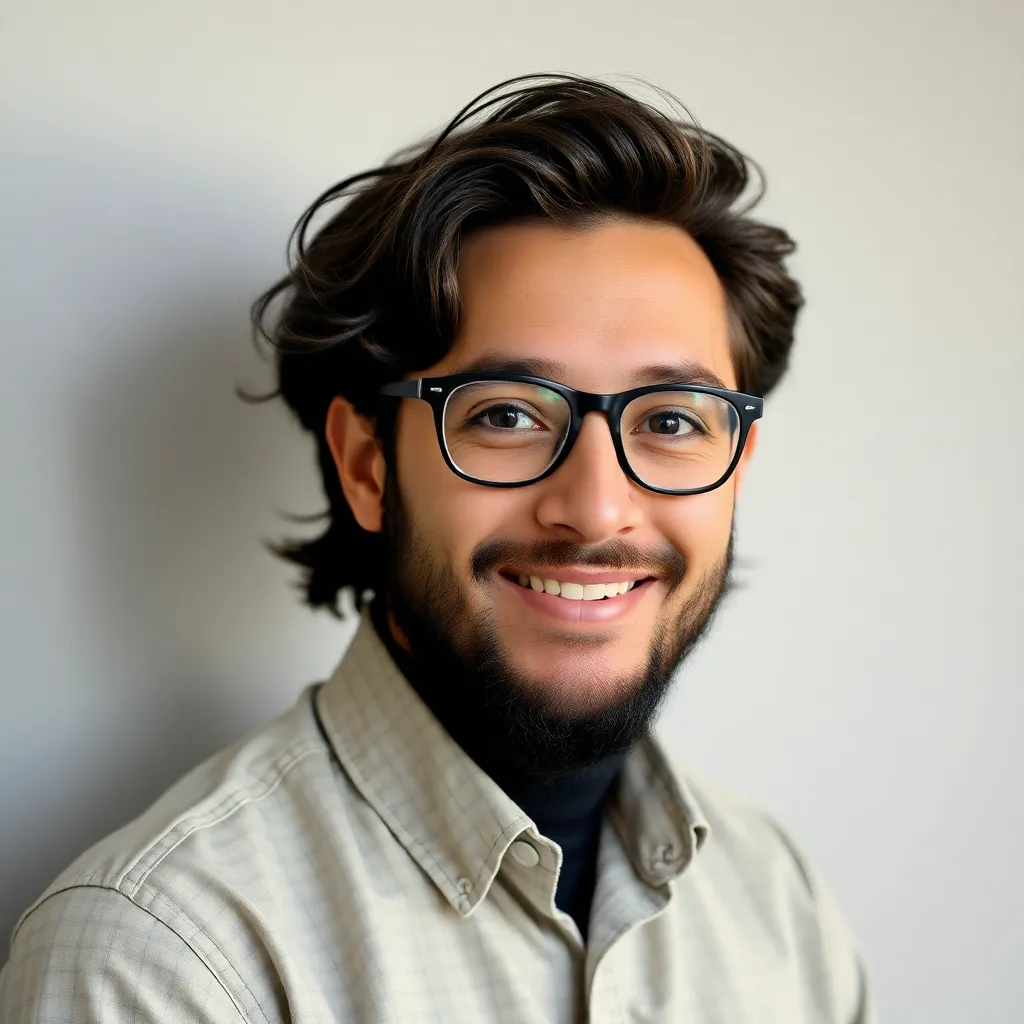
Breaking News Today
May 09, 2025 · 6 min read
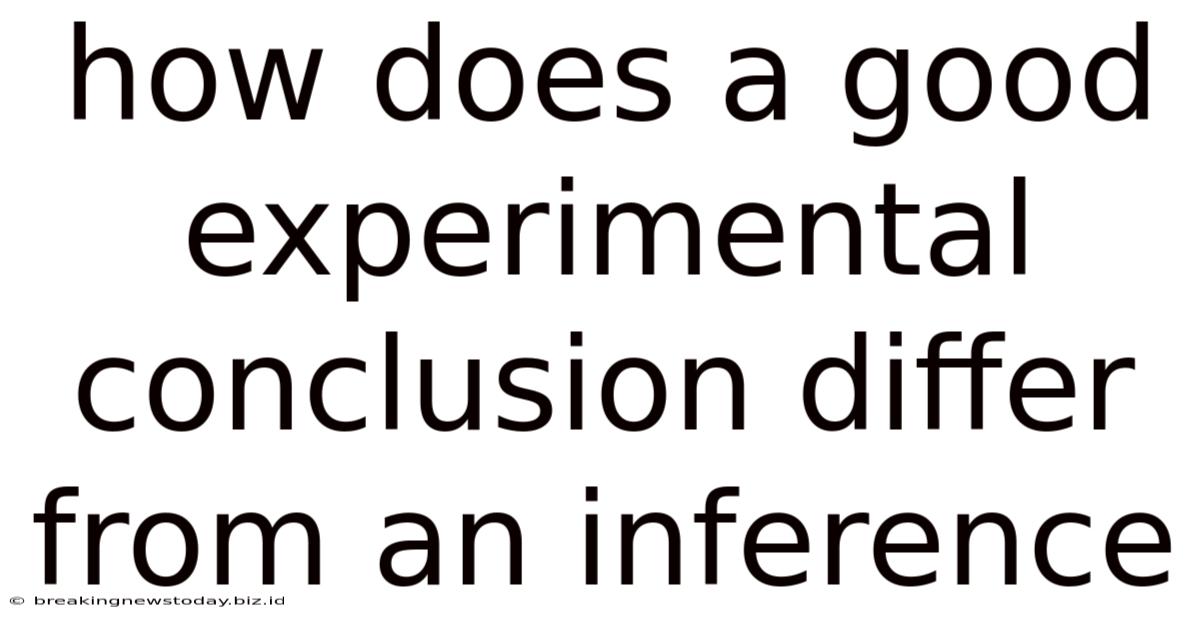
Table of Contents
How Does a Good Experimental Conclusion Differ from an Inference?
Understanding the difference between a conclusion and an inference, particularly within the context of experimental design, is crucial for rigorous scientific practice. While both involve drawing meaning from data, they operate at distinct levels of analysis and certainty. A strong experimental conclusion is a carefully reasoned statement built upon the evidence gathered, while an inference is a tentative interpretation that may or may not be supported by sufficient evidence. This article will explore these distinctions in detail, providing examples and clarifying the crucial elements that separate a well-supported conclusion from a mere inference.
The Foundation of a Solid Experimental Conclusion
A good experimental conclusion is not a haphazard guess or a subjective opinion. It's a carefully constructed statement directly derived from the experimental results and their analysis. This process involves several key steps:
1. Restating the Hypothesis and Objectives:
Before diving into the results, a strong conclusion begins by clearly restating the initial hypothesis and the overall objectives of the experiment. This serves as a crucial point of reference, allowing the reader to immediately understand the context of the findings. For instance, if the hypothesis was "Increased light exposure will lead to faster plant growth," the conclusion should begin by mentioning this hypothesis explicitly.
2. Summarizing the Key Findings:
The core of the conclusion involves summarizing the major findings of the experiment. This summary should be concise, focusing on the most significant data points and statistical analyses. Avoid overwhelming the reader with excessive detail; instead, highlight the patterns and trends that emerged from the data. Graphs, charts, and tables can be effectively used to visually represent these key findings, making the conclusion more accessible and impactful. For example, "The experimental group exposed to increased light showed a 25% increase in height compared to the control group after four weeks," is a concise and impactful summary.
3. Statistical Significance and Error Analysis:
A robust experimental conclusion always addresses the statistical significance of the findings. Were the observed differences statistically significant, or could they be attributed to random chance? The conclusion should clearly state the p-value obtained from statistical tests (e.g., t-test, ANOVA) and interpret its implications. Moreover, a discussion of potential sources of error and their impact on the results is crucial. Acknowledging limitations enhances the credibility of the conclusion, demonstrating a thorough and critical approach to the research process. "Statistical analysis revealed a significant difference (p<0.05) between the two groups, indicating that the observed increase in plant height was unlikely due to chance alone. However, variations in soil composition could have introduced some uncontrolled error," exemplifies this.
4. Directly Addressing the Hypothesis:
The conclusion must directly address the initial hypothesis, explicitly stating whether the results support, refute, or partially support it. Avoid ambiguous language; instead, use clear and precise statements. If the hypothesis is supported, the conclusion should explain how the data provides strong evidence for its validity. If the hypothesis is refuted, the conclusion should explain why the results contradict the initial prediction and suggest possible reasons for this discrepancy. "Therefore, the results strongly support the hypothesis that increased light exposure leads to faster plant growth," or, conversely, "These findings refute the initial hypothesis; further investigation into the potential influence of other factors, such as nutrient levels, is warranted," are strong and unambiguous statements.
5. Implications and Future Research:
A strong conclusion extends beyond simply summarizing the results; it also explores the broader implications of the findings and suggests avenues for future research. This demonstrates a forward-thinking approach, highlighting the potential impact of the work and identifying areas that require further investigation. For instance, "This research suggests the importance of optimizing light exposure for maximizing crop yields. Further studies could explore the optimal light intensity and duration for different plant species," shows a clear implication and future direction.
The Nature of Inference: Educated Guesswork
An inference, in contrast to a conclusion, is a logical interpretation or deduction based on available evidence but not directly proven by that evidence. It’s an educated guess or a reasoned assumption, often bridging the gap between observations and a broader understanding. The key difference lies in the level of certainty and the direct link to the experimental data.
Characteristics of Inferences:
- Tentative and Provisional: Inferences are not definitive statements; they are subject to revision as new evidence emerges.
- Based on Indirect Evidence: Inferences often rely on interpreting incomplete or indirect evidence.
- Subject to Bias: Inferences can be influenced by personal biases, preconceived notions, or limited perspectives.
- May Be Plausible But Not Proven: Even a well-reasoned inference remains a possibility rather than a certainty.
Examples of Inferences in Experimental Contexts:
Let's revisit the plant growth experiment. An inference might be: "The observed increase in plant height is likely due to an enhanced rate of photosynthesis resulting from increased light exposure." This statement is plausible, linking the observed increase in height to a known physiological process. However, it is an inference because it is not directly measured in the experiment; the rate of photosynthesis itself wasn't directly assessed. Other factors, such as increased nutrient uptake, could also explain the increased height.
Another inference might be: "This experiment suggests that similar results might be observed in other plant species under comparable light conditions." This extrapolates the findings from one specific plant species to a broader context, which is reasonable but not directly supported by the data.
The Crucial Distinctions: Conclusion vs. Inference
The table below summarizes the key distinctions between a good experimental conclusion and an inference:
Feature | Conclusion | Inference |
---|---|---|
Basis | Direct experimental results and statistical analysis | Indirect evidence, incomplete data, logical reasoning |
Certainty | High, supported by evidence | Low, tentative and subject to revision |
Directness | Directly addresses the hypothesis | Interprets the results, but not directly proven |
Objectivity | Aims for objectivity, minimizes bias | Can be influenced by bias and preconceived notions |
Statement Type | Definitive (within the limitations of the study) | Tentative, suggestive, plausible |
Avoiding Confusion: Best Practices
To avoid confusing conclusions and inferences, consider these best practices:
- Clearly Define Variables and Measurements: Precisely define the variables being measured and the methods used to collect data. This helps establish a clear link between the observed results and the conclusions drawn.
- Use Strong, Specific Language: Avoid vague or ambiguous language when expressing conclusions. Use precise wording that accurately reflects the findings.
- Acknowledge Limitations: Clearly state the limitations of the study and potential sources of error. This demonstrates a critical and self-aware approach to the research process.
- Differentiate between Observed and Inferred: Explicitly distinguish between the directly observed data and any interpretations or deductions made from that data. Label inferences clearly as such.
- Support Inferences with Evidence: Even when making inferences, provide evidence or reasoning to justify them. This makes the inferences more convincing and less speculative.
Conclusion (the Meta-Conclusion!)
Drawing accurate conclusions from experimental data is a critical skill in scientific inquiry. Understanding the subtle but important difference between a well-supported conclusion and a tentative inference is vital for communicating research findings effectively and accurately. By following best practices, researchers can avoid ambiguity and misinterpretations, fostering a greater level of rigor and transparency in scientific communication. Remember, a strong conclusion is the culmination of careful experimentation, meticulous analysis, and insightful interpretation—a far cry from a simple inference. The more meticulously the experiment is designed and the more rigorously the data is analyzed, the stronger and more convincing the conclusions will be.
Latest Posts
Latest Posts
-
Which Of The Following Is Not A Symptom Of Ptsd
May 09, 2025
-
Which Of The Following Does Not Influence Perception
May 09, 2025
-
Select The Account Classification That Matches With The Description
May 09, 2025
-
Unit 8 Test Study Guide Quadratic Equations
May 09, 2025
-
After 4 Minutes Of Rescue Breathing No Pulse
May 09, 2025
Related Post
Thank you for visiting our website which covers about How Does A Good Experimental Conclusion Differ From An Inference . We hope the information provided has been useful to you. Feel free to contact us if you have any questions or need further assistance. See you next time and don't miss to bookmark.