How Is A Sample Related To A Population
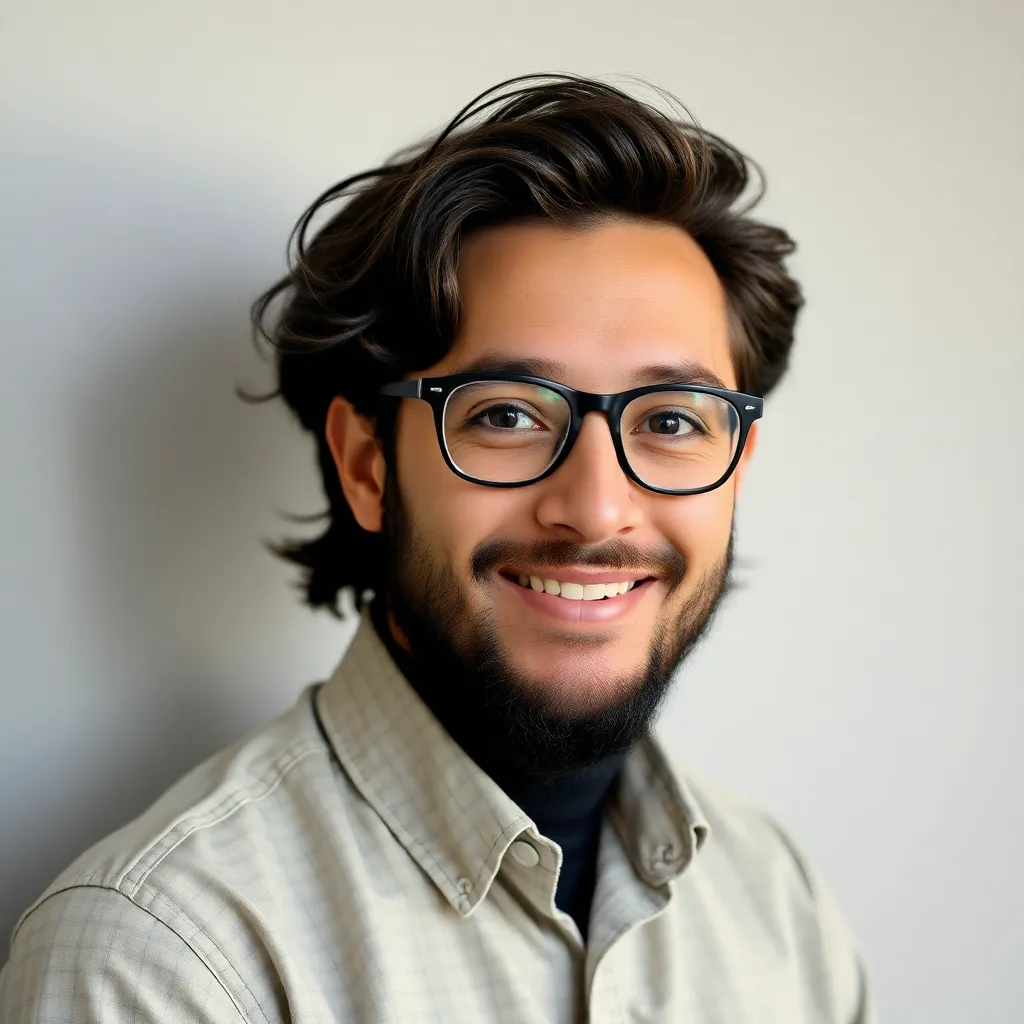
Breaking News Today
May 11, 2025 · 6 min read
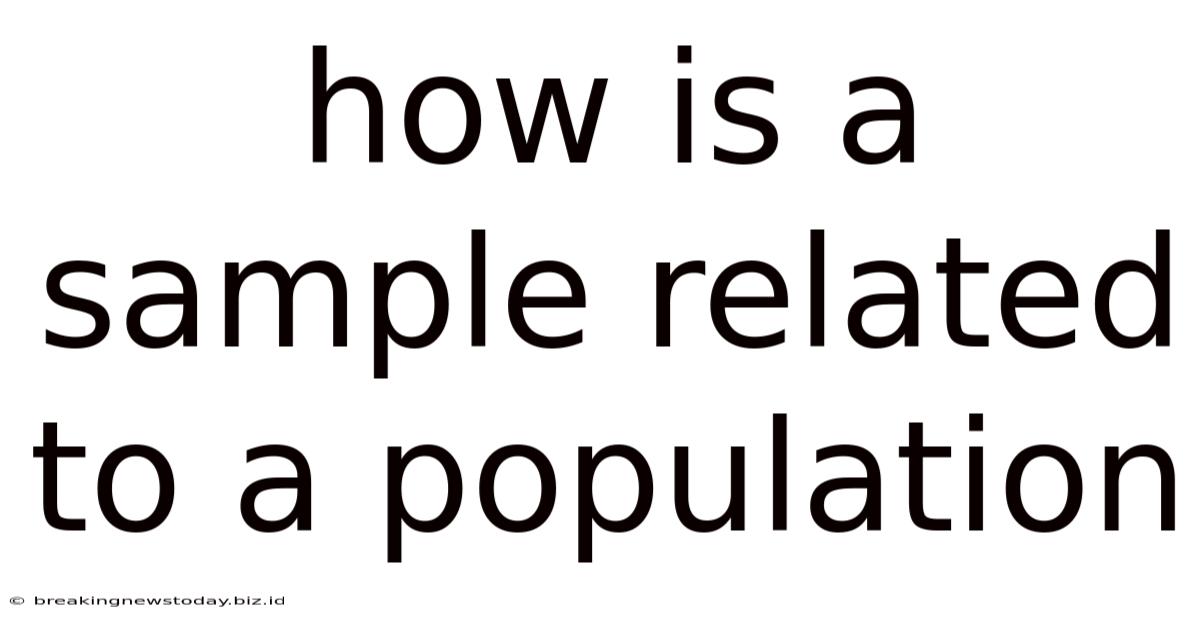
Table of Contents
How is a Sample Related to a Population? Understanding the Fundamentals of Statistical Inference
Statistics is the science of collecting, analyzing, interpreting, presenting, and organizing data. A core concept in statistics involves understanding the relationship between a sample and a population. This article delves deep into this crucial relationship, exploring the methodologies, challenges, and implications of using sample data to draw inferences about a larger population.
What is a Population?
In statistical terms, a population refers to the entire group of individuals, objects, events, or measurements that share a common characteristic of interest. This characteristic is the focus of the statistical investigation. The population can be anything from the entire adult population of a country to all the cars manufactured by a specific company in a particular year, or even all the grains of sand on a specific beach. It's crucial to define the population precisely before initiating any statistical analysis. A clearly defined population ensures that the results are relevant and accurately reflect the intended group.
Examples of Populations:
- All registered voters in the United States: This could be used to study voting patterns or political preferences.
- All students enrolled in a particular university: This could be used to study student performance, satisfaction, or demographic trends.
- All the trees in a specific forest: This could be used to study tree growth, health, or species distribution.
- All the components produced on a specific assembly line: This could be used to assess the quality and defect rate of the products.
What is a Sample?
A sample is a subset of the population, a smaller group selected from the population to represent it. Instead of examining every element within the entire population (which is often impractical, costly, or impossible), researchers study a sample to make inferences about the characteristics of the larger population. The accuracy and reliability of these inferences heavily depend on how well the sample represents the population. A representative sample accurately reflects the characteristics of the population from which it is drawn, minimizing bias and increasing the validity of the conclusions.
Examples of Samples:
- A randomly selected group of 1000 registered voters in the United States: Used to predict election outcomes.
- A randomly selected group of 50 students from a university: Used to gauge student satisfaction with university services.
- A randomly selected group of 100 trees from a specific forest: Used to estimate the average tree height.
- A randomly selected group of 50 components from an assembly line: Used to estimate the defect rate.
The Relationship Between Sample and Population: Inference and Generalization
The fundamental relationship between a sample and a population lies in statistical inference. This is the process of drawing conclusions about a population based on information obtained from a sample. We use the characteristics of the sample (e.g., mean, standard deviation, proportions) to estimate the corresponding characteristics of the population. This process involves generalization, extending the findings from the sample to the broader population. The goal is to make accurate generalizations about the population based on the evidence from the sample, with an understanding of the associated uncertainty.
The accuracy of these generalizations depends on several factors, most importantly the representativeness of the sample. A biased sample, one that doesn't accurately reflect the population's characteristics, will lead to inaccurate and misleading conclusions.
Methods of Sampling: Ensuring Representativeness
The method used to select the sample significantly impacts its representativeness. Several sampling techniques aim to create unbiased samples, each with its strengths and weaknesses:
1. Simple Random Sampling:
Every member of the population has an equal chance of being selected. This is achieved through techniques like lottery methods or random number generators. Simple random sampling is a foundation for many other sampling methods.
2. Stratified Sampling:
The population is divided into subgroups (strata) based on relevant characteristics (e.g., age, gender, income). A random sample is then drawn from each stratum, ensuring that the sample reflects the proportions of these characteristics in the population. This is particularly useful when dealing with populations that are heterogeneous.
3. Cluster Sampling:
The population is divided into clusters (groups), and a random sample of clusters is selected. All members within the selected clusters are then included in the sample. This is efficient for geographically dispersed populations.
4. Systematic Sampling:
Every kth member of the population is selected after a random starting point. This method is simple and straightforward but can be susceptible to bias if the population has a hidden pattern that aligns with the sampling interval.
5. Convenience Sampling:
This involves selecting individuals who are readily available and easily accessible. While convenient, convenience sampling is highly susceptible to bias and should be avoided whenever possible, especially in situations requiring reliable inferences.
Sampling Error and its Implications
Even with the most carefully planned sampling techniques, there will always be some degree of sampling error. This is the difference between the sample statistic (e.g., sample mean) and the true population parameter (e.g., population mean). Sampling error is inherent in the process of sampling and is a source of uncertainty in statistical inference. It's crucial to acknowledge and quantify this uncertainty.
The magnitude of sampling error can be estimated using statistical methods, allowing researchers to construct confidence intervals. Confidence intervals provide a range of values within which the true population parameter is likely to fall, with a specified level of confidence (e.g., 95% confidence interval).
Sample Size and its Importance
The size of the sample also plays a crucial role in the accuracy of statistical inferences. Larger samples generally lead to smaller sampling errors and more precise estimates of population parameters. However, increasing sample size also increases costs and time investment. Determining the optimal sample size involves balancing the desired precision with practical constraints. Statistical power analysis can help determine the appropriate sample size needed to detect a meaningful effect with a specified level of confidence.
Bias in Sampling: A Major Threat to Accuracy
Bias is a systematic error that can significantly distort the results of a statistical study. Bias can arise from various sources:
- Selection bias: Occurs when the sampling method systematically favors certain individuals or groups over others.
- Non-response bias: Occurs when a significant portion of the selected sample does not participate in the study. Non-respondents may differ systematically from respondents, leading to biased results.
- Measurement bias: Occurs when the method used to collect data systematically overestimates or underestimates the true value of the variable being measured.
Conclusion: The Essential Role of Sampling in Statistical Inference
The relationship between a sample and a population is fundamental to statistical inference. While it is often impractical or impossible to study an entire population, carefully selected samples can provide valuable insights into the characteristics of the population. Understanding sampling methods, sampling error, and potential biases is crucial for drawing accurate and reliable conclusions from sample data. By employing appropriate sampling techniques and acknowledging the inherent uncertainties, researchers can effectively use sample data to make informed decisions and gain valuable insights about populations of interest. Remember, the goal is to minimize bias, maximize representativeness, and quantify uncertainty to ensure the inferences drawn are both meaningful and reliable. The responsible and ethical use of sampling is essential for the integrity and credibility of statistical research. Careful planning, rigorous execution, and transparent reporting are critical for ensuring the value and validity of the results obtained from sample data.
Latest Posts
Latest Posts
-
Allows One Assembly To Attach To Another Without A Nut
May 11, 2025
-
How Do Strict Constructionists And Liberal Constructionists Differ
May 11, 2025
-
Which Of The Following Statements About Cellulitis Is True
May 11, 2025
-
Which Of The Following Best Describes A Gulf
May 11, 2025
-
Amca Fan Performance Classifications Are Based On
May 11, 2025
Related Post
Thank you for visiting our website which covers about How Is A Sample Related To A Population . We hope the information provided has been useful to you. Feel free to contact us if you have any questions or need further assistance. See you next time and don't miss to bookmark.