How Many Variables Should There Be In A Well-designed Experiment
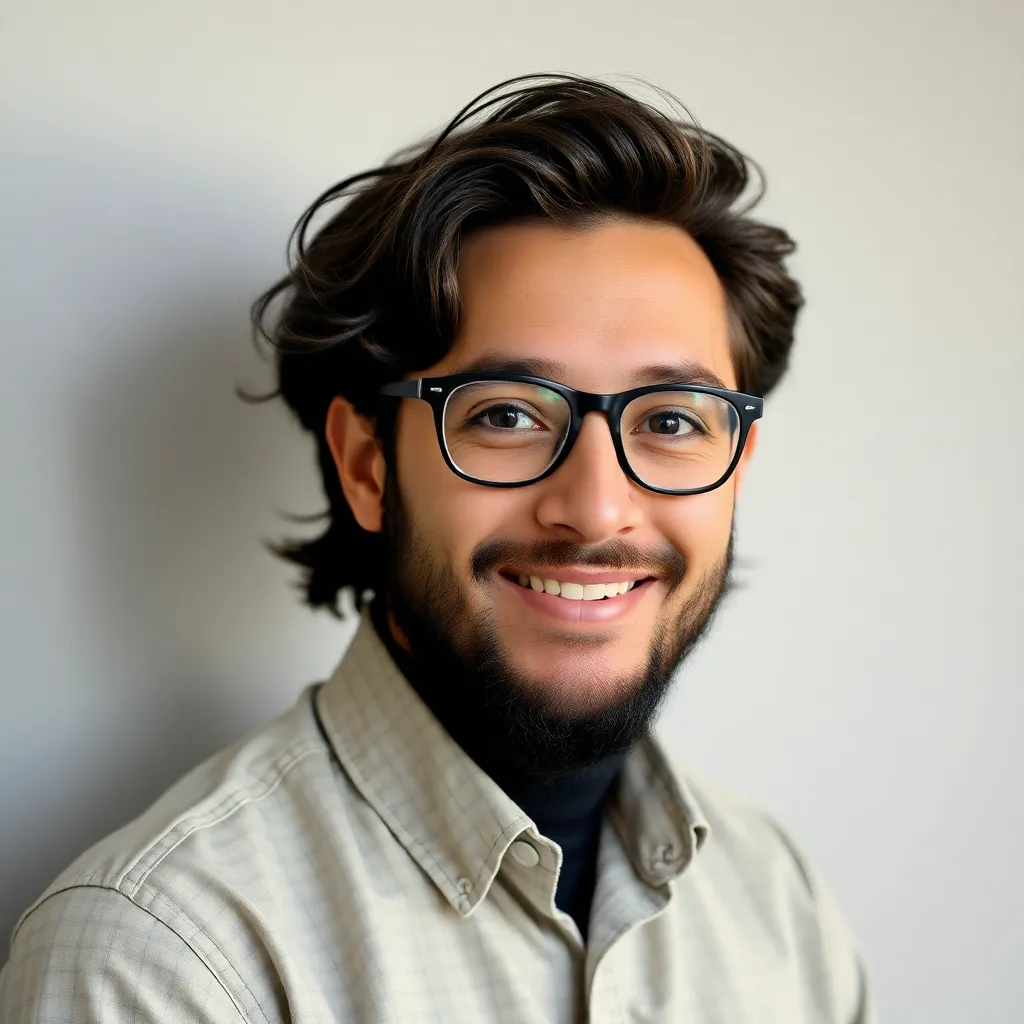
Breaking News Today
May 09, 2025 · 6 min read
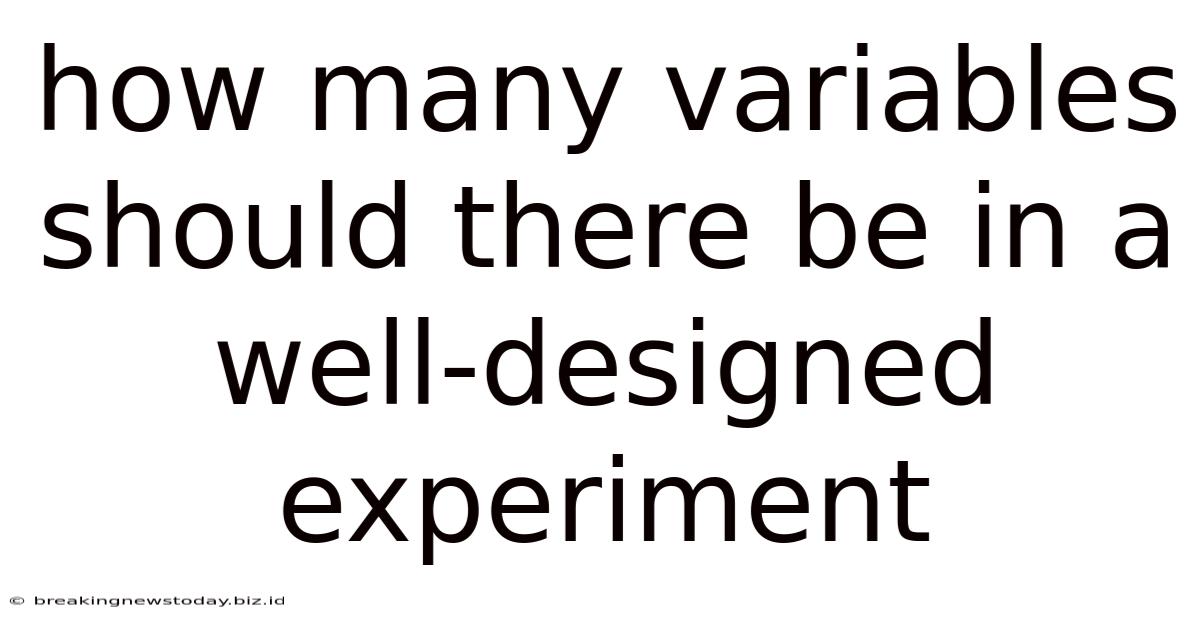
Table of Contents
How Many Variables Should There Be in a Well-Designed Experiment?
Determining the optimal number of variables in an experiment is a crucial aspect of research design. Too few variables can limit the scope and depth of your findings, while too many can lead to overwhelming complexity, confounding effects, and an inability to draw clear conclusions. The "sweet spot" depends heavily on the research question, available resources, and the chosen experimental design. This article delves into the complexities of variable selection, providing a comprehensive guide for researchers aiming to design robust and informative experiments.
Understanding the Types of Variables
Before tackling the number of variables, it's crucial to understand the different types:
1. Independent Variables (IVs):
These are the variables manipulated by the researcher. They are the potential causes you are investigating. A well-designed experiment typically focuses on a few key independent variables to isolate their individual and combined effects. Too many IVs can make it difficult to attribute observed effects to specific variables.
2. Dependent Variables (DVs):
These are the variables measured by the researcher. They are the effects or outcomes you're observing. You usually have one or a few primary DVs that directly address your research question. Secondary DVs might provide additional insights, but should be carefully considered to avoid overwhelming the analysis.
3. Control Variables:
These variables are held constant throughout the experiment to minimize their influence on the relationship between the IVs and DVs. Effective control is essential for establishing causality. While not directly part of the experimental manipulation, controlling for relevant factors is crucial for accurate interpretations.
4. Confounding Variables:
These are uncontrolled variables that might influence the DVs, leading to misleading results. Careful planning and rigorous experimental design aim to minimize confounding variables. Identifying and controlling for potential confounders is a critical step in ensuring the validity of your findings.
The Importance of Parsimony in Experimental Design
The principle of parsimony, also known as Occam's Razor, suggests that the simplest explanation is usually the best. This principle applies strongly to experimental design. While exploring multiple variables can be tempting, starting with a smaller, manageable number of IVs allows for a more focused and interpretable analysis. This reduces the risk of:
- Type I error: Falsely concluding that a relationship exists between variables when it doesn't.
- Type II error: Failing to detect a real relationship between variables.
- Overfitting: Developing a model that is too complex and performs poorly on new data.
- Increased experimental complexity: Managing many variables can make the experiment more difficult and time-consuming to conduct and analyze.
Factors Influencing the Number of Variables
The optimal number of variables isn't a fixed number. It's highly context-dependent and hinges on several factors:
1. Research Question:
A narrowly focused research question often requires fewer variables. A broader question might justify more, but even then, prioritizing the most critical variables is essential. Begin by clearly defining your research question and identifying the variables most directly related to it.
2. Resources:
Time, budget, participant availability, and equipment all constrain the feasibility of including numerous variables. Realistically assess your resources before designing an experiment that's too ambitious. It's better to conduct a smaller, well-designed experiment than a larger, poorly executed one.
3. Experimental Design:
Different designs accommodate different numbers of variables. Factorial designs, for example, systematically investigate the combined effects of multiple IVs. However, the number of conditions increases exponentially with each added IV, rapidly increasing complexity. A well-chosen experimental design can effectively handle several variables, while a poorly chosen one can make even a small number unwieldy.
4. Statistical Power:
The ability to detect a statistically significant effect is crucial. Including too many variables can reduce statistical power, making it harder to find real effects. Power analysis should be conducted before the experiment to determine the necessary sample size for detecting effects of a given magnitude with a desired level of confidence. This analysis can inform decisions about the number of variables to include.
Strategies for Managing Multiple Variables
When several variables are relevant, consider these approaches:
1. Prioritization:
Focus on the variables most directly related to your research question. Those that are less critical can be controlled, investigated in a separate experiment, or left for future research.
2. Factorial Designs (with caution):
Factorial designs allow you to examine the interactions between multiple IVs. However, the number of experimental conditions increases rapidly, necessitating careful consideration of resources and statistical power. Use fractional factorial designs or other efficient designs when the full factorial is impractical.
3. Blocking:
Grouping participants or experimental units based on relevant variables can reduce variability and improve the precision of your estimates. Blocking is a powerful technique to control for nuisance variables without explicitly including them in the experimental manipulation.
4. Moderation and Mediation Analysis:
These techniques explore how the relationship between IVs and DVs is influenced by other variables (moderators) or intermediate variables (mediators). These advanced statistical methods can allow for the inclusion of more variables while maintaining a manageable analysis.
5. Sequential Experiments:
Instead of trying to investigate numerous variables simultaneously, break the research into a series of smaller, focused experiments. Each experiment can focus on a subset of variables, building up a more comprehensive understanding over time. This approach reduces complexity and allows for more efficient resource allocation.
Practical Guidelines and Examples
While a definitive number is impossible, some general guidelines are:
- Start small: Begin with the core IVs directly addressing your research question.
- Consider interactions: If you suspect interactions between IVs, a factorial design might be appropriate, but start with a small number of factors.
- Conduct power analysis: Determine the required sample size to ensure sufficient statistical power.
- Control confounders: Carefully identify and control for potential confounding variables.
- Iterative process: Refinement often requires multiple rounds of experimental design and analysis.
Example 1 (Simple): A study investigating the effect of caffeine on reaction time might have one IV (caffeine dose) and one DV (reaction time). Control variables could be age, sleep deprivation, and time of day.
Example 2 (Moderate Complexity): A study examining the effectiveness of different teaching methods on student performance might have two IVs (teaching method, learning style) and one DV (test scores). Control variables might include prior knowledge and student motivation.
Example 3 (Complex): A clinical trial testing a new drug might have multiple IVs (dosage, treatment duration, patient subgroups) and several DVs (symptom severity, side effects, biomarkers). This complex scenario would require careful experimental design and advanced statistical analysis.
Conclusion: A Balanced Approach
The optimal number of variables in an experiment is a delicate balance between ambition and feasibility. Prioritize clarity and interpretability over sheer complexity. A well-designed experiment with a smaller, carefully chosen set of variables will produce more reliable and meaningful results than a sprawling experiment with too many uncontrolled factors. Thorough planning, robust design, and appropriate statistical analysis are crucial for successful research, regardless of the number of variables involved. Remember, the goal isn't to include every possible variable but to gain meaningful insights into the research question using a scientifically sound and manageable approach.
Latest Posts
Latest Posts
-
The First Stage In The Rational Decision Making Process Involves
May 09, 2025
-
Cells Divide Differentiate Or Die What Is Differentiation
May 09, 2025
-
Unpaid Referral Traffic Is Traffic That Comes From
May 09, 2025
-
If You Get Drowsy While Driving It Is Best To
May 09, 2025
-
The Objectives Of The Scene Designer Do Not Include
May 09, 2025
Related Post
Thank you for visiting our website which covers about How Many Variables Should There Be In A Well-designed Experiment . We hope the information provided has been useful to you. Feel free to contact us if you have any questions or need further assistance. See you next time and don't miss to bookmark.