In A Data Analytics Context What Is A Business Task
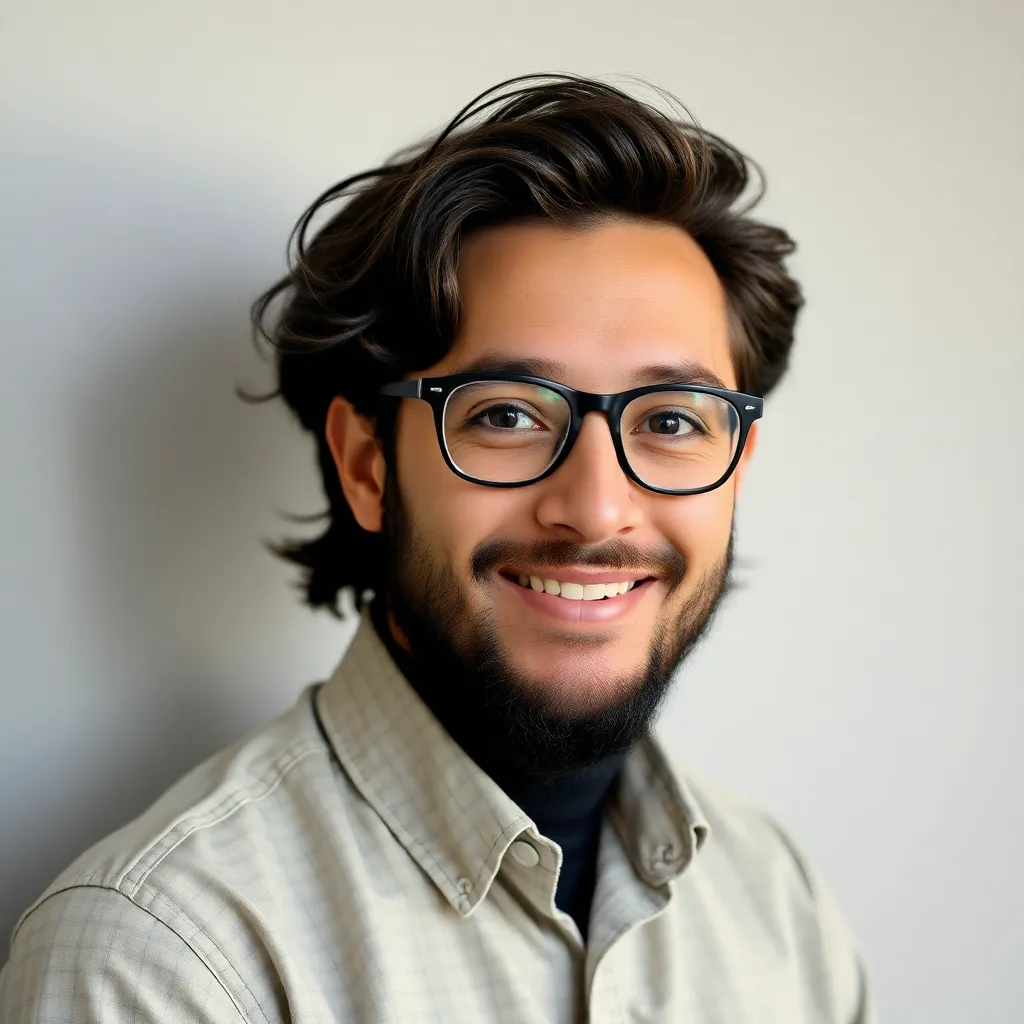
Breaking News Today
Apr 24, 2025 · 6 min read

Table of Contents
In a Data Analytics Context: What is a Business Task?
Data analytics is transforming how businesses operate, offering unprecedented insights into customer behavior, market trends, and operational efficiency. But the power of data is only unlocked when it's applied to solve specific business tasks. Understanding what constitutes a business task within a data analytics context is crucial for both data scientists and business stakeholders. This article will delve deep into this concept, exploring various types of business tasks, the data required to solve them, and the analytical methods employed.
Defining a Business Task in Data Analytics
A business task, in the context of data analytics, is a specific, measurable, achievable, relevant, and time-bound (SMART) objective that a business seeks to achieve using data-driven insights. It's not simply a general goal, but a clearly defined problem or opportunity that can be addressed through the analysis of data. These tasks directly impact business outcomes, driving strategic decisions and improvements across various departments.
Instead of vague statements like "improve customer satisfaction," a well-defined business task would be: "Increase customer retention rate by 15% within the next quarter by identifying and proactively addressing churn risk factors among high-value customers." This refined definition clearly outlines the desired outcome, the timeframe, and the target audience.
Key Characteristics of a Well-Defined Business Task:
- Specificity: The task is clearly stated, leaving no room for ambiguity.
- Measurability: Progress towards achieving the task can be quantitatively tracked.
- Achievability: The task is realistic and attainable within the given constraints.
- Relevance: The task aligns with the overall business objectives and strategic goals.
- Time-Bound: The task has a defined timeframe for completion.
Types of Business Tasks Addressed Through Data Analytics
Business tasks vary widely depending on the industry, business size, and specific goals. However, they generally fall into several key categories:
1. Customer Understanding & Segmentation:
This encompasses tasks related to understanding customer behavior, preferences, and needs. Examples include:
- Customer Segmentation: Identifying distinct customer groups based on shared characteristics (demographics, purchasing behavior, etc.) to tailor marketing campaigns and product offerings. This often involves clustering algorithms and other unsupervised learning techniques.
- Customer Churn Prediction: Forecasting which customers are likely to churn and developing strategies to retain them. This usually utilizes predictive modeling, incorporating variables like purchase frequency, customer service interactions, and engagement levels.
- Customer Lifetime Value (CLTV) Prediction: Estimating the total revenue a customer will generate throughout their relationship with the business. This helps prioritize customer acquisition and retention efforts. This involves statistical modeling and forecasting methods.
- Personalized Recommendations: Suggesting products or services relevant to individual customers based on their past behavior and preferences. This commonly employs collaborative filtering and content-based filtering techniques.
2. Marketing Optimization & Campaign Management:
Data analytics plays a crucial role in optimizing marketing strategies and measuring campaign effectiveness. Examples include:
- Marketing Campaign Performance Analysis: Assessing the success of marketing campaigns across various channels (e.g., email, social media, search engine marketing) by analyzing key metrics like click-through rates, conversion rates, and return on investment (ROI). This often involves A/B testing and multivariate testing.
- Lead Scoring & Qualification: Prioritizing leads based on their likelihood of converting into paying customers. This commonly involves predictive modeling and machine learning techniques.
- Channel Optimization: Identifying the most effective marketing channels for reaching the target audience and allocating resources accordingly. This often includes statistical analysis and attribution modeling.
3. Operations & Supply Chain Optimization:
Data analytics can streamline operational processes and improve supply chain efficiency. Examples include:
- Inventory Management: Optimizing inventory levels to minimize costs while ensuring sufficient stock to meet demand. This often involves forecasting models and inventory optimization techniques.
- Supply Chain Risk Management: Identifying potential disruptions in the supply chain and developing mitigation strategies. This often uses network analysis and simulation techniques.
- Predictive Maintenance: Forecasting equipment failures to minimize downtime and maintenance costs. This commonly involves machine learning algorithms and time series analysis.
- Process Optimization: Identifying bottlenecks and inefficiencies in operational processes to improve productivity and reduce costs. This frequently uses process mining and statistical process control methods.
4. Financial Analysis & Forecasting:
Data analytics enables businesses to gain insights into their financial performance and make better financial decisions. Examples include:
- Financial Forecasting: Predicting future revenue, expenses, and profitability. This often involves time series analysis and econometric modeling.
- Fraud Detection: Identifying fraudulent transactions and preventing financial losses. This frequently uses anomaly detection and machine learning techniques.
- Risk Management: Assessing and mitigating financial risks. This often involves statistical modeling and scenario planning.
5. Product Development & Innovation:
Data analytics informs product development decisions, ensuring products meet customer needs and market demands. Examples include:
- Product Feature Prioritization: Determining which features to develop based on customer feedback and market trends. This often involves sentiment analysis and conjoint analysis.
- A/B testing for product features: Evaluating the effectiveness of different product features by comparing user behavior under various conditions.
- New Product Development: Identifying opportunities for new products and services based on market research and customer insights. This often uses market basket analysis and other market research techniques.
Data Required for Addressing Business Tasks
The type of data required to solve a business task depends heavily on the specific task itself. However, some common data sources include:
- Transaction Data: Data related to sales, purchases, and other financial transactions.
- Customer Data: Information about customers, such as demographics, purchase history, and customer service interactions.
- Website Data: Data collected from website analytics, such as page views, bounce rates, and conversion rates.
- Social Media Data: Data from social media platforms, such as posts, comments, and engagement metrics.
- Sensor Data: Data collected from sensors, such as temperature, pressure, and humidity.
- Operational Data: Data related to business processes, such as production, logistics, and inventory.
Analytical Methods Employed to Solve Business Tasks
The choice of analytical methods depends on the specific business task and the type of data available. Common methods include:
- Descriptive Analytics: Summarizing past data to understand what happened. Examples include creating dashboards, reports, and summaries of key performance indicators (KPIs).
- Diagnostic Analytics: Identifying the reasons behind past events. Examples include root cause analysis and drill-down techniques.
- Predictive Analytics: Forecasting future outcomes based on past data. Examples include regression analysis, time series analysis, and machine learning techniques.
- Prescriptive Analytics: Recommending actions to optimize future outcomes. Examples include optimization algorithms, simulation, and decision support systems.
The Role of Data Scientists and Business Stakeholders
Successful data analytics projects require close collaboration between data scientists and business stakeholders. Data scientists possess the technical expertise to analyze data and build models, while business stakeholders provide the context and understanding of the business problem. Effective communication and a shared understanding of the business task are crucial for achieving positive outcomes.
Conclusion
Understanding what constitutes a business task in a data analytics context is fundamental to leveraging the power of data to drive business value. By clearly defining SMART business tasks, selecting the appropriate data sources, and employing suitable analytical methods, businesses can unlock valuable insights that inform strategic decision-making, improve operational efficiency, and enhance customer experiences. Remember, the journey starts with a well-defined question—the business task—that guides the entire data analytics process. The more precise and well-defined this task, the more impactful and relevant the resulting insights will be for the business.
Latest Posts
Latest Posts
-
The Overall Objective Of Financial Planning Is To
Apr 24, 2025
-
How Does An Emergency Action Plan Benefit Your Workplace
Apr 24, 2025
-
Scott Joplin Won The Pulitzer Prize Posthumously For Which
Apr 24, 2025
-
What Is The Best Definition Of The Term Cottage Industry
Apr 24, 2025
-
Under What Conditions Will Informational Social Influence Be More Likely
Apr 24, 2025
Related Post
Thank you for visiting our website which covers about In A Data Analytics Context What Is A Business Task . We hope the information provided has been useful to you. Feel free to contact us if you have any questions or need further assistance. See you next time and don't miss to bookmark.