In A Statistical Problem A Population Consists Of
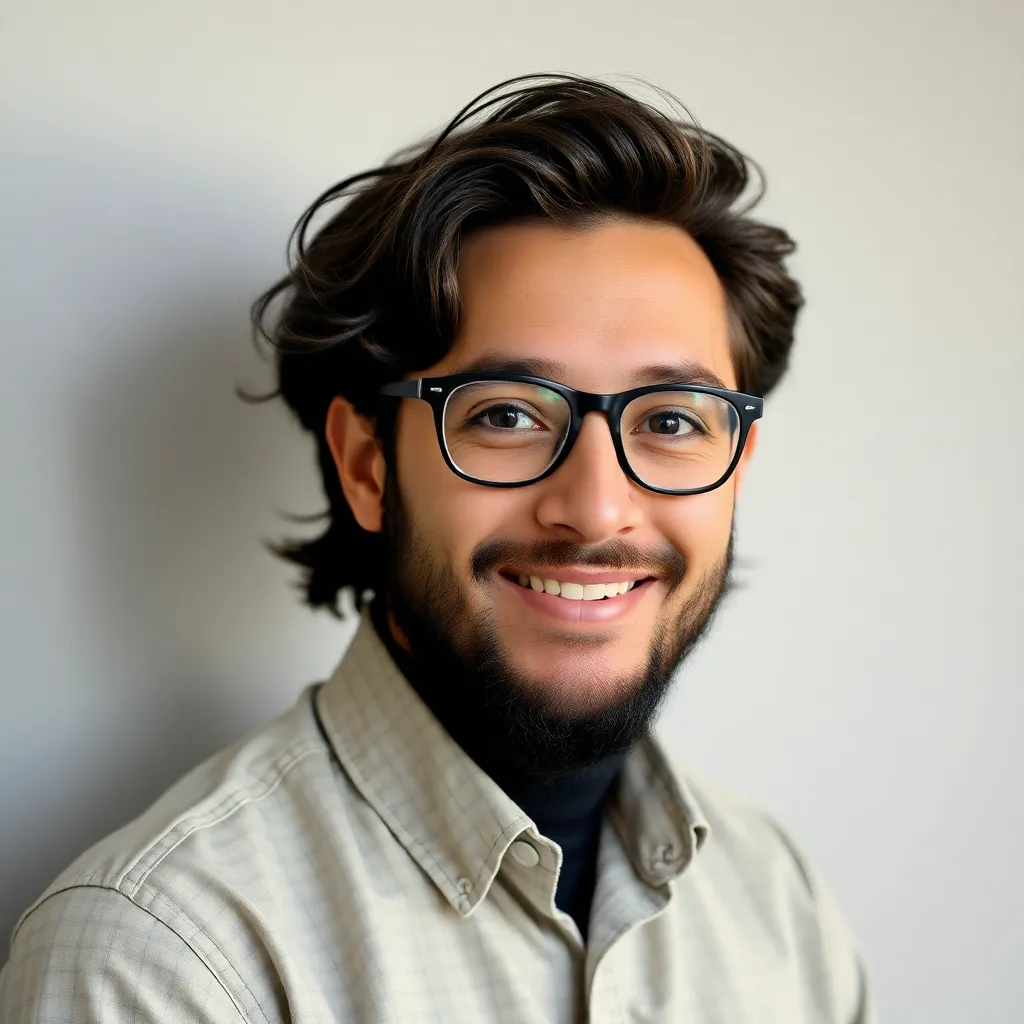
Breaking News Today
Apr 06, 2025 · 7 min read
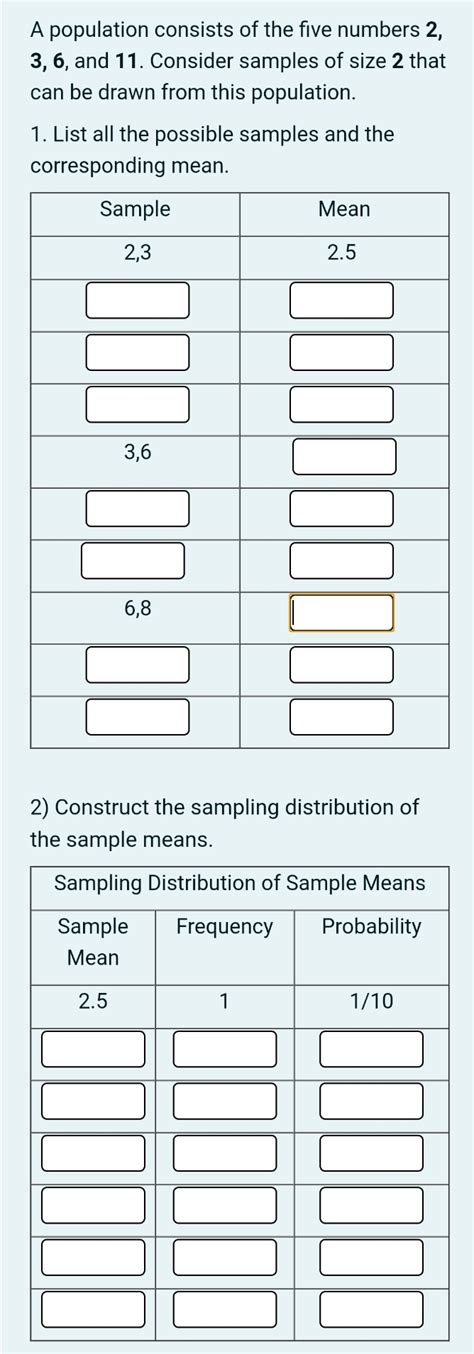
Table of Contents
In a Statistical Problem: A Deep Dive into Populations, Samples, and Inference
Understanding populations is fundamental to statistics. This article will explore the concept of a population in a statistical problem, delving into its characteristics, the crucial role of samples, and how we use sample data to make inferences about the entire population. We'll cover key statistical terms and concepts to provide a comprehensive understanding of this vital area of statistical analysis.
What is a Population in Statistics?
In statistical terms, a population refers to the entire group of individuals, objects, events, or measurements that share a common characteristic of interest. This characteristic is the focus of the statistical investigation. It's crucial to define the population precisely to ensure the accuracy and relevance of the statistical analysis. The population can be anything from:
- The entire human population of a country: Studying average income, age distribution, or health status.
- All the cars produced by a specific manufacturer in a year: Analyzing fuel efficiency, defect rates, or customer satisfaction.
- All the trees in a forest: Measuring height, diameter, or species distribution.
- All the possible outcomes of rolling a six-sided die: Investigating probabilities of different outcomes.
The key is that the population represents the complete set of entities you are interested in studying. It is the target of your investigation, and your goal is often to learn something about this population. However, studying an entire population is often impractical, expensive, or even impossible. This is where the concept of sampling comes into play.
The Importance of Samples: Why We Don't Study Entire Populations
Examining every single member of a population is often infeasible. Consider trying to measure the height of every tree in the Amazon rainforest or survey every person in the world about their political views. The cost, time, and logistical challenges would be insurmountable.
This is why statisticians rely heavily on samples. A sample is a subset of the population, carefully selected to represent the characteristics of the entire population. The sample is used to make inferences about the larger population. The quality of the inferences depends heavily on how well the sample represents the population. A poorly chosen sample can lead to biased or inaccurate conclusions.
The process of selecting a sample is called sampling. There are many different sampling techniques, each with its own strengths and weaknesses. Some common methods include:
- Simple random sampling: Every member of the population has an equal chance of being selected.
- Stratified sampling: The population is divided into subgroups (strata), and a random sample is taken from each stratum.
- Cluster sampling: The population is divided into clusters, and a random sample of clusters is selected. All members of the selected clusters are then included in the sample.
- Systematic sampling: Members of the population are selected at regular intervals.
The choice of sampling method significantly impacts the accuracy and reliability of the statistical inferences. A well-designed sampling strategy is crucial for drawing meaningful conclusions.
Parameters and Statistics: Describing Populations and Samples
When dealing with populations and samples, we use different terms to describe their characteristics:
-
Parameters: Numerical values that describe a characteristic of the population. These are usually unknown and are what we aim to estimate. Examples include the population mean (μ), population standard deviation (σ), and population proportion (P).
-
Statistics: Numerical values that describe a characteristic of the sample. These are calculated from the sample data and are used to estimate the corresponding population parameters. Examples include the sample mean (x̄), sample standard deviation (s), and sample proportion (p).
Understanding the distinction between parameters and statistics is fundamental. We use sample statistics to make inferences about population parameters. For example, we might use the sample mean (x̄) to estimate the population mean (μ).
Inferential Statistics: Drawing Conclusions about Populations from Samples
Inferential statistics is the branch of statistics that deals with drawing conclusions about a population based on information obtained from a sample. This involves using sample statistics to estimate population parameters and to test hypotheses about the population. Key inferential techniques include:
-
Confidence intervals: These provide a range of values within which we are confident that the true population parameter lies. For example, a 95% confidence interval for the population mean indicates that we are 95% confident that the true mean falls within that interval.
-
Hypothesis testing: This involves formulating a hypothesis about a population parameter and then using sample data to test the validity of that hypothesis. This typically involves calculating a test statistic and determining its p-value, which represents the probability of obtaining the observed results if the hypothesis is true.
The reliability of inferential statistics depends heavily on the size and representativeness of the sample. Larger, more representative samples generally lead to more accurate and reliable inferences.
Types of Data and Their Relevance to Population Studies
The type of data collected also influences the statistical methods used to analyze the population. Common data types include:
-
Nominal data: Categorical data with no inherent order (e.g., colors, gender). Analysis often involves frequencies and proportions.
-
Ordinal data: Categorical data with a meaningful order (e.g., education levels, customer satisfaction ratings). Analysis might involve rank-order statistics.
-
Interval data: Numerical data with meaningful intervals but no true zero point (e.g., temperature in Celsius or Fahrenheit). Arithmetic operations like addition and subtraction are meaningful.
-
Ratio data: Numerical data with a true zero point (e.g., height, weight, income). All arithmetic operations are meaningful.
Understanding the type of data allows researchers to choose appropriate statistical methods for analysis and interpretation.
Dealing with Sampling Error and Bias
Even with careful sampling, there will always be some difference between the sample statistics and the true population parameters. This difference is called sampling error. Sampling error is a natural consequence of not being able to study the entire population. However, we can minimize sampling error by using larger, more representative samples.
Sampling bias occurs when the sample is not representative of the population. This can lead to inaccurate and misleading conclusions. Bias can arise from various sources, including:
- Selection bias: Occurs when the method of selecting the sample systematically excludes certain parts of the population.
- Non-response bias: Occurs when a significant portion of the selected sample does not respond to the survey or study.
- Measurement bias: Occurs when the method of measuring the variable of interest is flawed or inaccurate.
Identifying and mitigating bias is crucial for ensuring the validity of the statistical inferences.
Advanced Statistical Concepts Related to Populations
Several advanced statistical concepts build upon the foundation of populations and sampling:
-
Regression analysis: Used to model the relationship between a dependent variable and one or more independent variables in a population. Often involves estimating population parameters of the regression model.
-
Analysis of variance (ANOVA): Used to compare the means of multiple groups or populations.
-
Bayesian statistics: Provides a framework for incorporating prior knowledge or beliefs about the population into the statistical analysis.
-
Time series analysis: Used to analyze data collected over time, often to predict future values in a population.
These techniques allow for more complex and nuanced analyses of population characteristics.
Conclusion: The Pivotal Role of Populations in Statistics
Understanding populations is paramount in statistical analysis. While studying an entire population is often impossible, carefully selected samples allow statisticians to make inferences about population characteristics with a certain degree of confidence. The choice of sampling method, the type of data collected, and awareness of potential biases are crucial for accurate and reliable conclusions. By mastering these concepts, researchers can effectively analyze data and draw meaningful insights about the world around us. The accuracy and reliability of these insights ultimately depend on a solid understanding of populations, samples, and the principles of statistical inference. Further exploration into the advanced techniques mentioned above will enhance one's ability to conduct rigorous and meaningful statistical analyses.
Latest Posts
Latest Posts
-
I Want To Pay The Check Duolingo
Apr 06, 2025
-
Drivers Ed Final Exam 50 Questions Maryland
Apr 06, 2025
-
Which Statement Best Describes The Skeletal System
Apr 06, 2025
-
The Try Square Is Made At A Fixed
Apr 06, 2025
-
Which Area Of Corporate Law Is Connected To Technology
Apr 06, 2025
Related Post
Thank you for visiting our website which covers about In A Statistical Problem A Population Consists Of . We hope the information provided has been useful to you. Feel free to contact us if you have any questions or need further assistance. See you next time and don't miss to bookmark.