Jason Reads A Report That Says 80
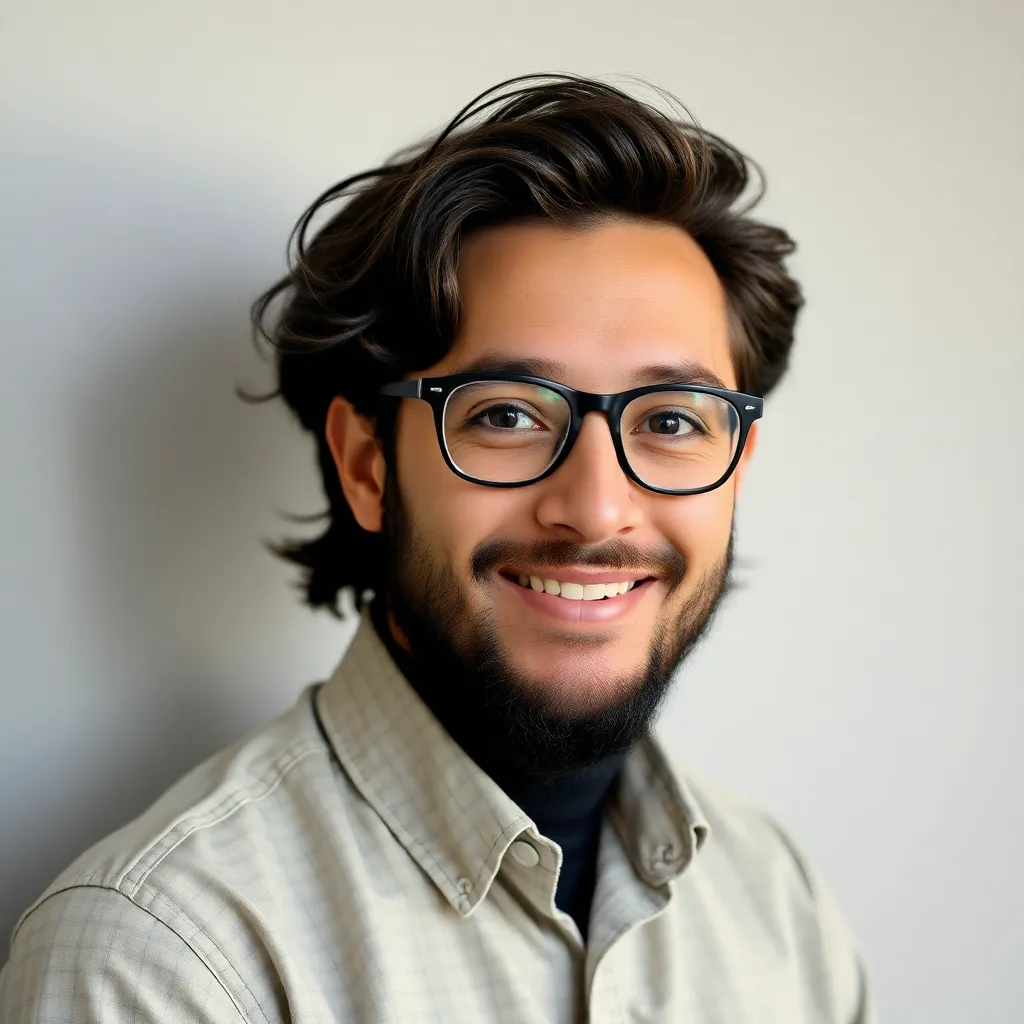
Breaking News Today
May 12, 2025 · 6 min read
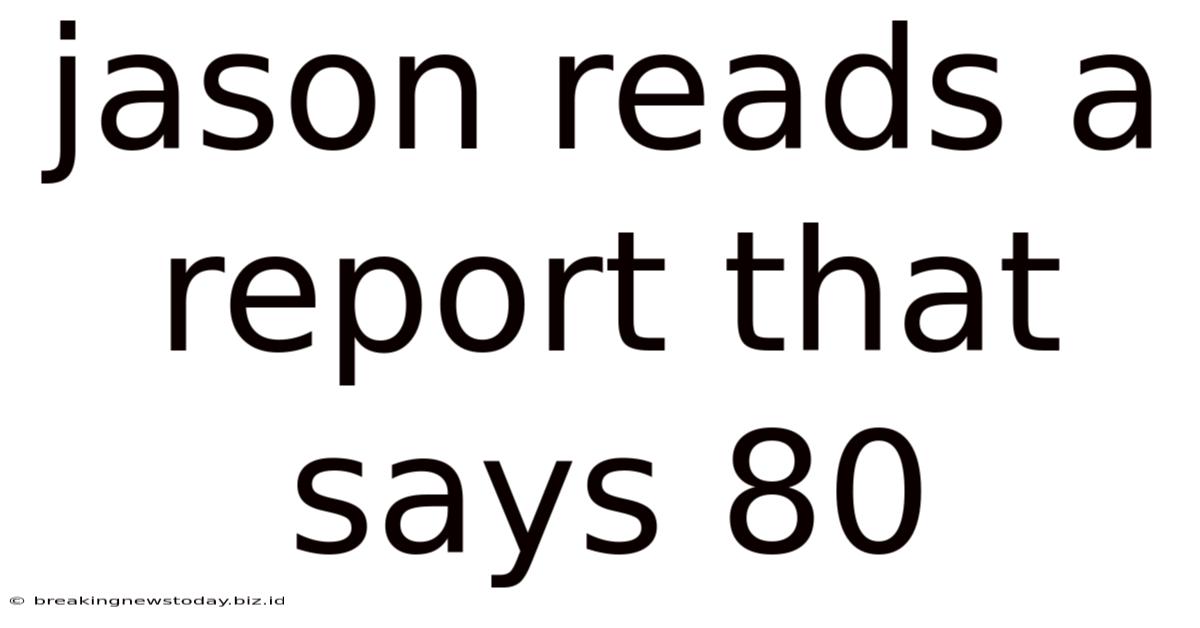
Table of Contents
Jason Reads a Report That Says 80%: A Deep Dive into the Power of Data and its Interpretation
Jason, a seasoned data analyst at a bustling tech startup, leaned back in his chair, the glow of his computer screen illuminating his thoughtful face. Before him, a meticulously crafted report flashed a stark statistic: 80%. This wasn't just any number; it represented the projected user engagement for their latest app feature, a revolutionary AI-powered personal assistant. But the number, while seemingly positive, triggered a cascade of questions in Jason's mind. Was this 80% truly indicative of success? What factors contributed to this figure, and what lurked beneath the surface? This seemingly simple statistic demanded a deep dive, a thorough analysis that went beyond the headline number.
Unpacking the 80%: Beyond the Surface Level
The report, compiled by a team of junior analysts, presented the 80% engagement rate as a resounding victory. However, Jason knew that raw data, without context, can be deceptive. He needed to understand the methodology, the sample size, and the underlying assumptions before he could draw any meaningful conclusions. The 80% might represent a monumental achievement, or it could be a misleading mirage built on shaky foundations.
Here's where Jason's analytical skills came into play:
-
Methodology Scrutiny: The first thing Jason did was to carefully examine the methodology used to arrive at the 80% figure. Was it based on a randomized controlled trial (RCT), a more robust method, or a less rigorous observational study? The way data is collected significantly impacts its reliability. A biased sampling method, for instance, could inflate or deflate the actual engagement rate. He also looked for any potential biases in data collection. Were certain user demographics over-represented? This could skew the results.
-
Sample Size Evaluation: A large, representative sample size is critical for reliable results. If the 80% engagement was based on a small sample, the result could be statistically insignificant, meaning the high engagement rate might be just random chance rather than a true reflection of the app's performance. Jason needed to understand how the sample size was determined and whether it was sufficiently large to make generalizations about the entire user base.
-
Definition of "Engagement": What exactly constituted "engagement" in this report? Did it simply refer to app usage, or did it also encompass more meaningful interactions, such as completing tasks, using advanced features, or providing positive feedback? A vague definition of engagement could inflate the reported rate without reflecting genuine user satisfaction. A specific and comprehensive definition of engagement is crucial.
-
Contextual Factors: Jason knew that the 80% engagement couldn't be viewed in isolation. He needed to consider various contextual factors such as:
-
Market Trends: Is the 80% rate higher or lower than industry benchmarks for similar apps? Comparison with competitors provides valuable insights and perspective.
-
Marketing Efforts: Were there any significant marketing campaigns launched concurrently with the new feature? If so, the reported engagement might be partly attributed to the marketing push and not solely to the feature's inherent appeal.
-
User Demographics: Did the engagement rate vary significantly across different user demographics (age, location, device type)? Identifying such variations provides a richer understanding of the feature's appeal and potential areas for improvement.
-
The Power of Data Visualization: Painting a Clearer Picture
Jason understood that raw numbers, however meticulously collected, can be difficult to interpret. He used data visualization techniques to create charts and graphs that presented the data in a more accessible and insightful manner. By visualizing the data, he could identify patterns and trends that were not immediately apparent from the raw data.
Some visualizations Jason might employ include:
-
Bar Charts: To compare engagement rates across different demographics or geographical locations.
-
Line Charts: To illustrate engagement trends over time, showing whether engagement is increasing, decreasing, or remaining stable.
-
Pie Charts: To showcase the proportion of users who engaged with the feature compared to those who did not.
-
Heatmaps: To visualize engagement patterns across different parts of the app interface.
Through these visualizations, Jason could quickly identify outliers and potential issues, focusing his further analysis on specific areas of concern.
Beyond the 80%: A Deeper Look at User Behavior
Jason also dove deep into qualitative data to understand why users were or weren't engaging with the new feature. The 80% only told part of the story. To gain a holistic understanding, he needed to investigate the underlying reasons for user behavior.
This might involve:
-
User Feedback Analysis: Scrutinizing user reviews, app store ratings, and feedback from focus groups or surveys to unearth valuable insights into user experiences and satisfaction levels. Positive feedback supports the high engagement rate, while negative feedback could highlight areas for improvement.
-
A/B Testing Results: If A/B testing was employed, Jason would scrutinize the results to understand which variations of the feature resonated better with users. This provides data-driven insights for future design iterations.
-
In-App Analytics: Analyzing user flows within the app, including time spent on different screens, navigation patterns, and task completion rates, could reveal pain points and areas for optimization. This could explain why the engagement rate is 80% and not higher.
Iterative Improvement: The Continuous Cycle of Analysis and Refinement
Jason understood that the 80% wasn't a final destination but rather a stepping stone. Data analysis is an iterative process—a cycle of analysis, refinement, and continuous improvement. Based on his thorough investigation, Jason might identify areas for enhancement:
-
Feature Refinement: If user feedback revealed usability issues or areas for improvement, Jason could recommend design tweaks to enhance user experience and boost engagement even further.
-
Targeted Marketing: By understanding which user segments engage most with the feature, Jason could recommend targeted marketing campaigns to reach a wider audience and improve overall engagement.
-
Improved Onboarding: If the analysis reveals that users struggle to understand the feature, Jason might recommend improvements to the onboarding process to ensure users quickly grasp its functionality and value proposition.
The Importance of Transparency and Communication
Once Jason completed his analysis, he knew it was crucial to communicate his findings clearly and transparently to stakeholders. A simple statement like "80% engagement" is insufficient. His report needed to include:
-
Detailed Methodology: A clear explanation of how the 80% figure was derived, including the sample size, data collection methods, and definition of engagement.
-
Data Visualization: Charts and graphs that presented the data in a clear and concise manner.
-
Key Findings: A summary of the most important findings, along with their implications for the app's future development.
-
Recommendations: Specific, actionable recommendations for improving the app based on the analysis.
By communicating his findings transparently, Jason could build trust and foster collaboration among stakeholders, ensuring everyone is on the same page and working towards the same goals.
Conclusion: The Journey Beyond 80%
Jason's journey with the 80% engagement report highlighted the crucial role of data analysis in decision-making. The number itself, while seemingly straightforward, concealed a wealth of information that required careful examination and interpretation. By applying rigorous analytical techniques, utilizing data visualization effectively, and exploring qualitative data, Jason transformed a single statistic into a powerful tool for understanding user behavior and driving app improvement. The 80% wasn't just a number; it was a springboard for continuous improvement, a testament to the power of data-driven decision-making, and a reminder that true understanding goes far beyond surface-level interpretations. The pursuit of deeper insights, beyond the initial figure, ensures that data is not merely reported, but used effectively to guide strategic decisions and ultimately, to achieve success. The journey to exceed the 80% is an ongoing process of refinement, adaptation, and a deep commitment to understanding the users' needs and experiences.
Latest Posts
Latest Posts
-
A Partial Bath Includes Washing A Residents
May 12, 2025
-
Which Of The Following Describes A Net Lease
May 12, 2025
-
Nurse Logic 2 0 Knowledge And Clinical Judgment
May 12, 2025
-
Panic Disorder Is Characterized By All Of The Following Except
May 12, 2025
-
Positive Individual Traits Can Be Taught A True B False
May 12, 2025
Related Post
Thank you for visiting our website which covers about Jason Reads A Report That Says 80 . We hope the information provided has been useful to you. Feel free to contact us if you have any questions or need further assistance. See you next time and don't miss to bookmark.