Mathematical Statistics With Applications 7th Edition Solutions
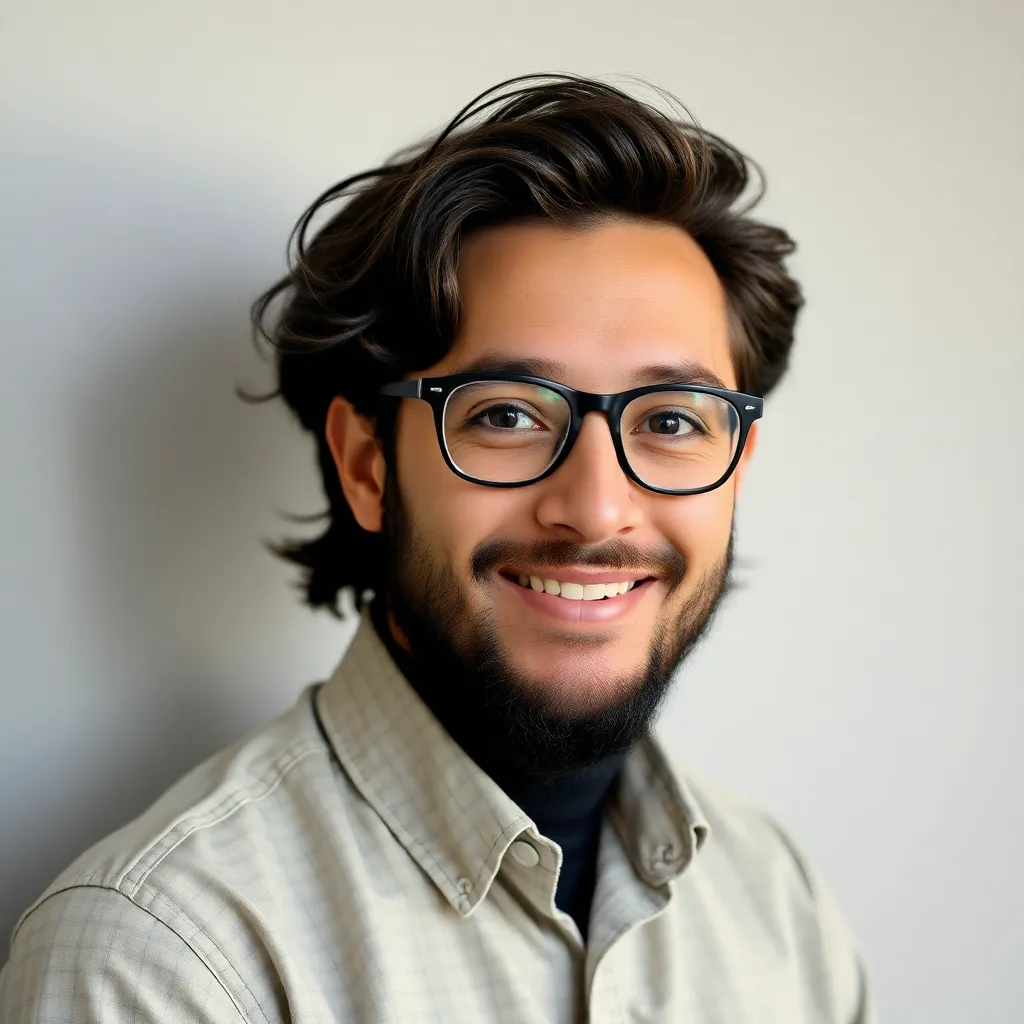
Breaking News Today
May 10, 2025 · 6 min read
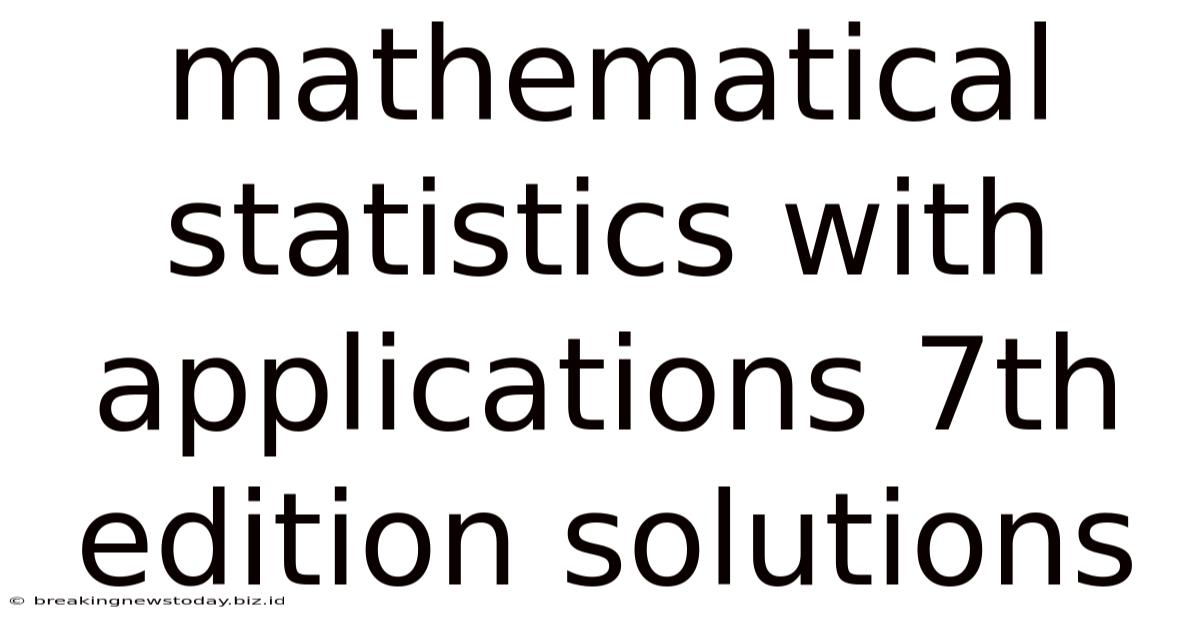
Table of Contents
Mathematical Statistics with Applications, 7th Edition: A Comprehensive Guide to Solving Problems
Mathematical Statistics with Applications, 7th edition, by Wackerly, Mendenhall, and Scheaffer, is a cornerstone text for many undergraduate and graduate statistics courses. Its comprehensive coverage of statistical theory and its numerous applications make it a valuable resource, but the sheer volume of material can be daunting for students. This article aims to provide a comprehensive guide to navigating the textbook and tackling its problem sets, offering insights into key concepts and problem-solving strategies. We won't provide direct solutions, as that defeats the purpose of learning, but instead offer a framework for approaching different problem types.
Understanding the Core Concepts: A Foundation for Success
Before diving into specific problems, it's crucial to have a strong grasp of the fundamental concepts presented in each chapter. The 7th edition covers a wide range of topics, including:
1. Descriptive Statistics: Summarizing Data
This section lays the groundwork for statistical inference. Mastering descriptive statistics is crucial for understanding data distributions and identifying patterns. Key concepts include:
- Measures of Central Tendency: Mean, median, mode. Understanding the properties and appropriate use of each measure is essential. Problems often involve calculating these measures for different data sets and interpreting their implications.
- Measures of Dispersion: Variance, standard deviation, range, interquartile range. These measures quantify the spread or variability within a data set. Be prepared to calculate these measures and interpret their meaning in the context of the problem.
- Data Visualization: Histograms, boxplots, scatterplots. Understanding how to create and interpret these visual representations of data is critical for identifying patterns and trends. Many problems will require you to construct and analyze these plots.
2. Probability: The Foundation of Inference
Probability is the backbone of statistical inference. A thorough understanding of probability distributions is crucial for solving many problems in the textbook. Key areas to focus on include:
- Discrete Probability Distributions: Binomial, Poisson, hypergeometric. Understanding the assumptions and applications of each distribution is vital. Many problems will involve calculating probabilities using these distributions.
- Continuous Probability Distributions: Normal, exponential, gamma. Similar to discrete distributions, mastering the properties and applications of continuous distributions is crucial. Problems often involve calculating probabilities, percentiles, and other characteristics of these distributions.
- Joint Probability Distributions: Understanding how to work with multiple random variables and their joint distributions is essential for more advanced topics like regression and correlation.
3. Statistical Inference: Drawing Conclusions from Data
This is arguably the most important section of the book, focusing on techniques for making inferences about populations based on sample data. Key topics include:
- Point Estimation: Understanding different methods of estimating population parameters (e.g., mean, variance) from sample data. Problems often involve calculating point estimates and evaluating their properties (bias, efficiency).
- Interval Estimation: Constructing confidence intervals for population parameters. This involves understanding the concept of confidence level and margin of error. Problems will require you to calculate confidence intervals and interpret their meaning.
- Hypothesis Testing: Formulating and testing hypotheses about population parameters. This involves understanding concepts like null and alternative hypotheses, p-values, and Type I and Type II errors. Many problems require conducting hypothesis tests and interpreting the results.
4. Regression Analysis: Modeling Relationships Between Variables
This section delves into techniques for modeling the relationship between a dependent variable and one or more independent variables. Key topics include:
- Simple Linear Regression: Modeling the relationship between two variables. Problems often involve estimating the regression equation, testing the significance of the regression coefficients, and making predictions.
- Multiple Linear Regression: Extending simple linear regression to include multiple independent variables. This involves understanding concepts like multicollinearity and model selection.
- Model Assumptions and Diagnostics: Understanding the assumptions underlying regression models and how to check for violations of these assumptions is crucial for ensuring the validity of the results.
5. Analysis of Variance (ANOVA): Comparing Means
ANOVA techniques are used to compare the means of several groups. Key aspects include:
- One-Way ANOVA: Comparing the means of two or more groups with a single factor. Problems often involve conducting an ANOVA test and interpreting the results.
- Two-Way ANOVA: Extending one-way ANOVA to include two factors and their interaction.
- Post-Hoc Tests: Determining which groups differ significantly after a significant ANOVA result.
Effective Problem-Solving Strategies
Successfully tackling the problems in Mathematical Statistics with Applications, 7th edition, requires a structured approach:
-
Thorough Understanding of Concepts: Before attempting any problem, ensure you understand the underlying theoretical concepts. Review the relevant sections of the textbook and consult additional resources if needed.
-
Careful Problem Reading: Read each problem carefully, identifying the key information and what is being asked. Underline or highlight important details.
-
Identify the Appropriate Method: Determine the appropriate statistical method to use based on the problem statement and the type of data.
-
Clearly Define Variables and Parameters: Clearly define all variables and parameters involved in the problem. This helps in avoiding confusion and errors.
-
Show Your Work: Always show your work, including all calculations and intermediate steps. This helps in identifying errors and facilitates understanding.
-
Interpret Your Results: Don't just calculate numbers; interpret the results in the context of the problem. What do your findings mean? What conclusions can you draw?
-
Use Technology Wisely: Statistical software packages (like R, SPSS, or SAS) can significantly aid in solving complex problems. However, ensure you understand the underlying concepts before relying solely on software.
-
Practice Regularly: The key to mastering statistical methods is consistent practice. Work through numerous problems, gradually increasing the complexity.
Navigating Specific Problem Types
While providing direct solutions is impossible within this format, we can outline approaches to common problem types:
-
Probability Problems: Clearly identify the type of probability distribution involved. Use the appropriate formulas and tables (or software) to calculate probabilities. Pay close attention to whether the problem involves discrete or continuous variables.
-
Confidence Interval Problems: Identify the appropriate confidence level and critical value. Calculate the margin of error and construct the confidence interval. Interpret the results in the context of the problem.
-
Hypothesis Testing Problems: Clearly state the null and alternative hypotheses. Calculate the test statistic and p-value. Compare the p-value to the significance level (alpha) to make a decision about the null hypothesis. Interpret the results in the context of the problem.
-
Regression Problems: Estimate the regression equation using the appropriate method. Assess the goodness of fit of the model using metrics like R-squared. Test the significance of the regression coefficients. Make predictions based on the estimated model. Analyze residuals to check for model assumptions.
-
ANOVA Problems: Calculate the F-statistic and p-value. Compare the p-value to the significance level to determine whether there is a significant difference among the group means. If significant, use post-hoc tests to determine which groups differ significantly.
Conclusion: Mastering Mathematical Statistics
Mathematical Statistics with Applications, 7th edition, offers a challenging yet rewarding journey into the world of statistics. By understanding the core concepts, employing effective problem-solving strategies, and practicing consistently, you can successfully navigate the textbook's problems and build a strong foundation in mathematical statistics. Remember, the process of learning and problem-solving is as important as obtaining the correct answer. Embrace the challenges, persist in your efforts, and you will reap the benefits of a deep understanding of this essential field. This article serves as a roadmap, guiding you through the complexities, providing a framework for tackling the challenges, and ultimately empowering you to excel in your studies. Good luck!
Latest Posts
Latest Posts
-
Explain How Employee Benefits Affect Total Employment Compensation
May 10, 2025
-
What Fields Are Left Blank For Medicare Claims
May 10, 2025
-
How Long Have You Been In The Navy
May 10, 2025
-
Where Does Aj Dad Find Aj Phone
May 10, 2025
-
Bleeding From The Larynx Is Known As
May 10, 2025
Related Post
Thank you for visiting our website which covers about Mathematical Statistics With Applications 7th Edition Solutions . We hope the information provided has been useful to you. Feel free to contact us if you have any questions or need further assistance. See you next time and don't miss to bookmark.