Optical Scanners Recognize Individual Letters Or Images
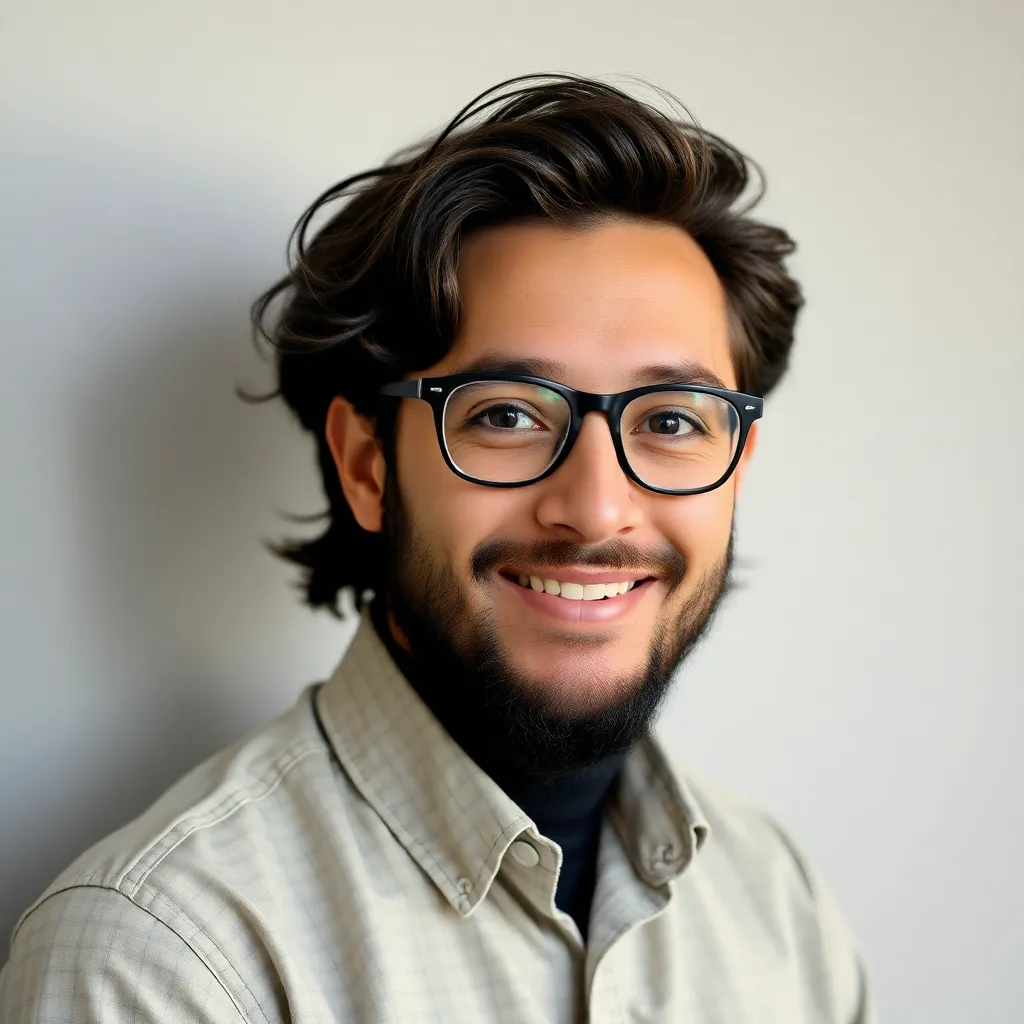
Breaking News Today
May 11, 2025 · 7 min read
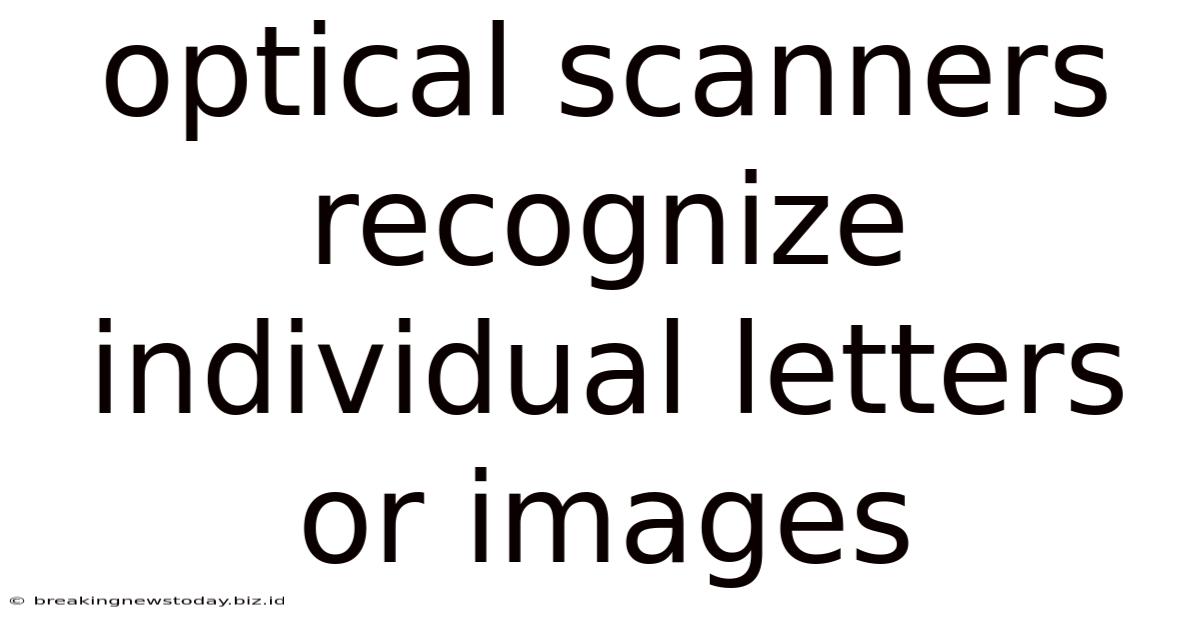
Table of Contents
Optical Scanners: Decoding the World, One Letter and Image at a Time
Optical scanners, ubiquitous devices in our digital age, play a crucial role in bridging the gap between the physical and digital worlds. Their ability to convert printed text, images, and other visual data into digital formats is transformative, impacting diverse fields from document management and healthcare to retail and manufacturing. But how exactly do these seemingly simple devices achieve this complex feat? This article delves into the fascinating world of optical scanners, exploring their inner workings, the various technologies employed, and their ongoing evolution. We'll unravel the mysteries of how optical scanners recognize individual letters, images, and other visual data, revealing the sophisticated processes behind their seemingly effortless performance.
The Mechanics of Image Acquisition
Before we can understand how optical scanners recognize individual letters or images, we need to grasp the fundamentals of image acquisition. The process begins with a light source, typically a LED or fluorescent lamp, illuminating the document or object being scanned. This light reflects off the surface, carrying information about the text, images, or other visual elements present. This reflected light then passes through a series of components designed to capture and process the visual information.
The Role of the Light Source and its Impact on Image Quality
The quality of the light source significantly impacts the final scanned image. A consistent and even light source minimizes shadows and ensures uniform illumination, resulting in clearer and more accurate image capture. Variations in light intensity can lead to uneven brightness levels, impacting the accuracy of character recognition (OCR) and overall image quality. Advanced scanners often incorporate sophisticated light source management systems to mitigate these issues, leading to superior image quality.
The Imaging Sensor: Capturing the Reflected Light
The reflected light then interacts with the imaging sensor, the heart of the scanning process. This sensor, typically a Charged Coupled Device (CCD) or Complementary Metal-Oxide-Semiconductor (CMOS) sensor, converts the reflected light into an electrical signal. The sensor is composed of millions of tiny light-sensitive elements called pixels, each capturing a small portion of the reflected light. The greater the number of pixels, the higher the resolution of the scanned image, resulting in sharper details and improved accuracy in character and image recognition. High-resolution scanners are crucial for applications requiring fine detail, such as scanning high-resolution photographs or documents with small print.
From Light to Data: The Conversion Process
Once the imaging sensor converts the reflected light into an electrical signal, the data needs to be processed and converted into a digital format that computers can understand. This involves several crucial steps:
Analog-to-Digital Conversion (ADC): Transforming Signals into Digital Data
The electrical signals from the imaging sensor are analog in nature, meaning they vary continuously. To make them usable by a computer, they must be converted into digital data using an Analog-to-Digital Converter (ADC). The ADC samples the analog signal at regular intervals, assigning a discrete numerical value to each sample. The precision of this conversion, measured in bits, determines the color depth and the overall quality of the scanned image. Higher bit depths provide richer color representation and greater detail, leading to improved recognition accuracy.
Image Processing: Enhancing and Refining the Raw Data
The raw digital data from the ADC often requires further processing to improve its quality and prepare it for character and image recognition. This image processing stage may involve several steps, including:
- Noise Reduction: Removing unwanted artifacts and imperfections in the scanned image.
- Contrast Enhancement: Improving the difference between light and dark areas to enhance the clarity of text and images.
- Sharpening: Improving the definition of edges and details for better recognition accuracy.
- Color Correction: Adjusting color balance and intensity to ensure accurate color representation.
These processes are critical for optimizing the quality of the scanned data, ensuring that the subsequent character and image recognition steps are as accurate as possible.
Optical Character Recognition (OCR): Decoding Text
Optical Character Recognition (OCR) is the process of converting scanned text into editable text files. This involves sophisticated algorithms that analyze the scanned image, identifying individual characters and converting them into their corresponding digital representations. The accuracy of OCR depends heavily on factors such as image quality, font type, and the complexity of the text.
The Stages of OCR: From Pixels to Text
OCR typically involves the following stages:
- Image Preprocessing: This stage prepares the scanned image for character recognition, often involving steps like noise reduction, skew correction (straightening tilted text), and binarization (converting the image to black and white).
- Character Segmentation: The image is divided into individual characters, each of which is analyzed separately.
- Feature Extraction: Key features of each character are extracted, such as the number of loops, lines, and curves.
- Character Classification: The extracted features are compared to a database of known characters, identifying the most likely match. This often involves machine learning algorithms trained on vast amounts of data.
- Post-Processing: This final step involves checking for errors and correcting them, often involving spell-checking and context analysis.
Advanced OCR systems employ sophisticated algorithms, often incorporating neural networks and deep learning techniques, resulting in significantly improved accuracy. They can handle various fonts, styles, and languages, making them indispensable tools for digitizing large volumes of text.
Image Recognition: Beyond Text
While OCR focuses on text recognition, image recognition encompasses a much broader range of capabilities. Image recognition involves identifying and classifying objects, scenes, and patterns within an image. This is a significantly more complex task than OCR, requiring more advanced algorithms and computational power.
Techniques in Image Recognition: Feature Extraction and Classification
Several techniques are employed in image recognition, including:
- Feature Extraction: Similar to OCR, image recognition relies on extracting key features from the image. These features might include edges, corners, textures, and other visual patterns. The choice of features depends on the specific recognition task.
- Machine Learning: Machine learning algorithms are crucial for classifying images based on their extracted features. These algorithms are trained on vast datasets of images, learning to identify patterns and distinguish different objects or scenes. Convolutional Neural Networks (CNNs) are particularly effective in image recognition, excelling at identifying complex patterns.
Image recognition is used in various applications, including:
- Medical Imaging: Identifying abnormalities in medical scans.
- Facial Recognition: Identifying individuals based on their facial features.
- Object Detection: Identifying and locating objects within an image.
- Scene Understanding: Interpreting the context and content of an image.
The ongoing advancements in machine learning and deep learning are continuously improving the accuracy and capabilities of image recognition systems.
The Future of Optical Scanners: Enhanced Capabilities and Integration
Optical scanners are continuously evolving, benefiting from advancements in imaging technology, computational power, and artificial intelligence. Several trends are shaping the future of these devices:
- Improved Resolution and Speed: Higher resolution sensors and faster processing capabilities are leading to faster scanning speeds and higher-quality images.
- Enhanced OCR and Image Recognition: Advancements in machine learning are dramatically improving the accuracy and capabilities of OCR and image recognition systems, enabling the handling of more complex documents and images.
- Increased Automation: Optical scanners are becoming increasingly automated, minimizing the need for manual intervention. This includes features such as automatic document feeding, automatic skew correction, and automatic image processing.
- Integration with Cloud Services: Many modern scanners integrate seamlessly with cloud services, enabling easy storage, sharing, and collaboration on scanned documents.
As technology progresses, we can expect optical scanners to play an even greater role in digitizing our world, making information more accessible and enhancing efficiency across a wide range of applications. The journey from reflected light to digital data, from pixels to text and images, is a testament to human ingenuity and the ongoing quest to bridge the gap between the physical and digital realms.
Latest Posts
Latest Posts
-
As The Resistance Decreases The Blood Flow
May 12, 2025
-
Loss Of Innocence Quotes Lord Of The Flies
May 12, 2025
-
A Is The Surgical Repair Of The Mouth
May 12, 2025
-
Cats And Dogs Reading Passage Teas Test
May 12, 2025
-
Which Of The Following Quantities Represent Mass
May 12, 2025
Related Post
Thank you for visiting our website which covers about Optical Scanners Recognize Individual Letters Or Images . We hope the information provided has been useful to you. Feel free to contact us if you have any questions or need further assistance. See you next time and don't miss to bookmark.