Probability And Statistics For Engineering And The Sciences
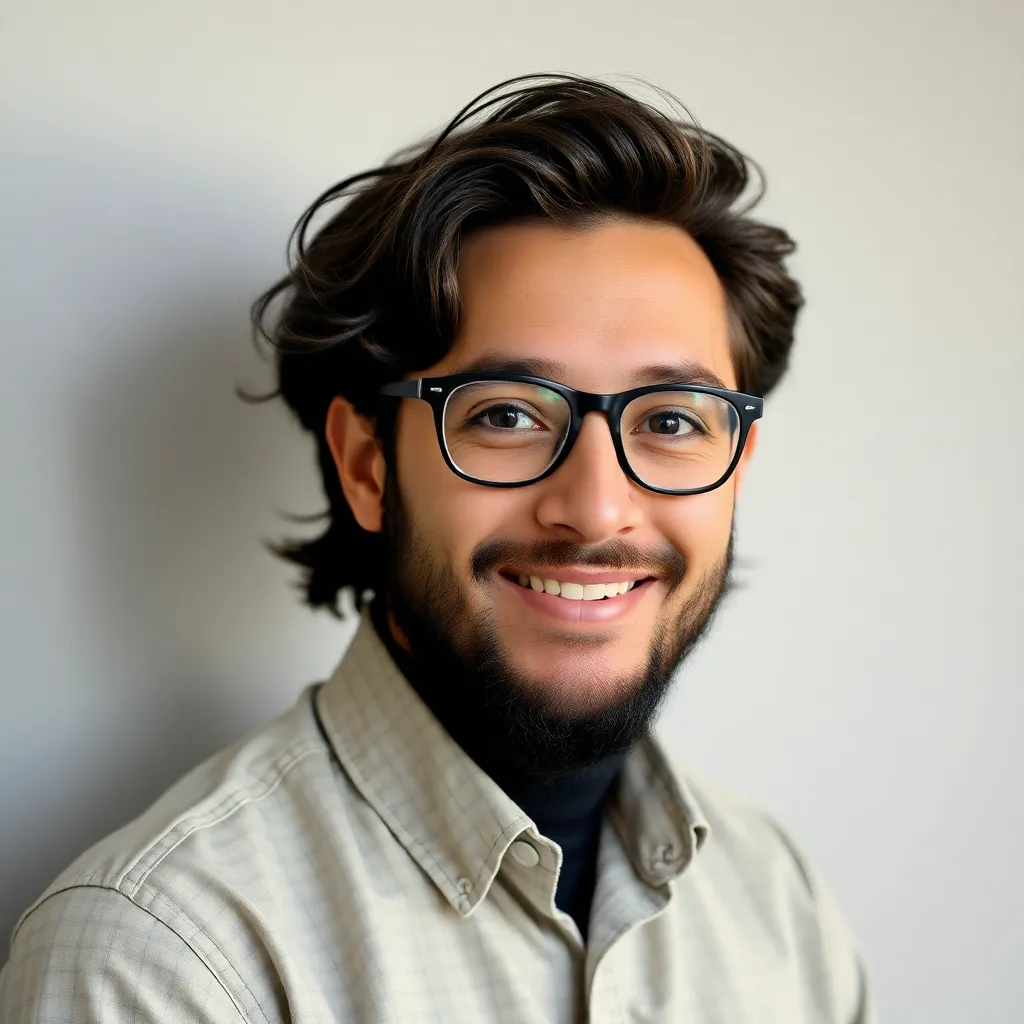
Breaking News Today
May 09, 2025 · 7 min read
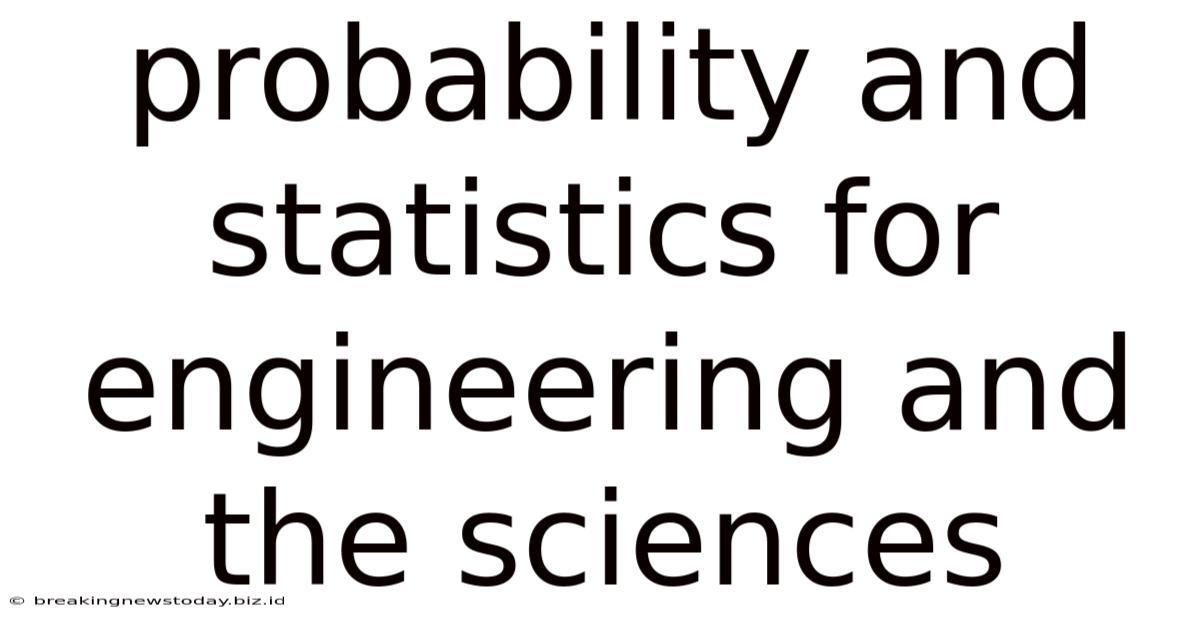
Table of Contents
Probability and Statistics for Engineering and the Sciences: A Comprehensive Guide
Probability and statistics are fundamental tools for engineers and scientists across numerous disciplines. From designing reliable systems to analyzing experimental data and making informed predictions, a solid understanding of these concepts is paramount. This comprehensive guide delves into the key principles of probability and statistics, highlighting their applications in engineering and the sciences.
Understanding Probability
Probability quantifies the likelihood of an event occurring. It's expressed as a number between 0 and 1, where 0 represents impossibility and 1 represents certainty. Several key concepts underpin probability theory:
1. Sample Space and Events
The sample space (S) is the set of all possible outcomes of a random experiment. An event (E) is a subset of the sample space; it's a specific outcome or a collection of outcomes. For example, if you roll a die, the sample space is S = {1, 2, 3, 4, 5, 6}. The event "rolling an even number" is E = {2, 4, 6}.
2. Types of Probability
Several methods exist for determining the probability of an event:
-
Classical Probability: Assumes all outcomes in the sample space are equally likely. The probability of an event E is calculated as: P(E) = (Number of favorable outcomes) / (Total number of outcomes). For instance, the probability of rolling a 3 on a fair die is 1/6.
-
Relative Frequency Probability: Based on observed frequencies of events in a large number of trials. The probability is estimated as: P(E) = (Number of times E occurred) / (Total number of trials). This approach is particularly useful when the theoretical probabilities are unknown.
-
Subjective Probability: Reflects an individual's belief about the likelihood of an event, based on experience, judgment, or intuition. This is often used in situations with limited data or uncertainty.
3. Probability Rules
Several fundamental rules govern probability calculations:
-
Addition Rule: Used to find the probability of either event A or event B occurring: P(A ∪ B) = P(A) + P(B) - P(A ∩ B), where P(A ∩ B) is the probability of both A and B occurring. If A and B are mutually exclusive (cannot occur simultaneously), then P(A ∩ B) = 0.
-
Multiplication Rule: Used to find the probability of both event A and event B occurring: P(A ∩ B) = P(A) * P(B|A), where P(B|A) is the conditional probability of B occurring given that A has already occurred. If A and B are independent (the occurrence of one doesn't affect the other), then P(B|A) = P(B).
-
Conditional Probability: The probability of event A occurring given that event B has already occurred: P(A|B) = P(A ∩ B) / P(B).
-
Bayes' Theorem: A powerful tool for updating probabilities based on new evidence: P(A|B) = [P(B|A) * P(A)] / P(B).
Introduction to Statistics
Statistics involves collecting, organizing, analyzing, interpreting, and presenting data. It complements probability by providing methods to analyze real-world data and draw inferences about populations based on samples.
1. Descriptive Statistics
Descriptive statistics summarize and describe the main features of a dataset. Key measures include:
-
Measures of Central Tendency: These describe the "center" of the data. Examples include the mean (average), median (middle value), and mode (most frequent value).
-
Measures of Dispersion: These describe the spread or variability of the data. Examples include the range (difference between maximum and minimum values), variance (average squared deviation from the mean), and standard deviation (square root of the variance).
-
Data Visualization: Graphs and charts (histograms, box plots, scatter plots) provide visual summaries of data, revealing patterns and trends.
2. Inferential Statistics
Inferential statistics uses sample data to make inferences about a larger population. Key techniques include:
-
Hypothesis Testing: A formal procedure to determine whether there is enough evidence to reject a null hypothesis (a statement about the population). This involves setting a significance level (alpha), calculating a test statistic, and determining a p-value. If the p-value is less than alpha, the null hypothesis is rejected.
-
Confidence Intervals: Provide a range of values within which a population parameter (e.g., mean) is likely to lie with a certain level of confidence. For example, a 95% confidence interval means there's a 95% probability that the true population parameter falls within the calculated interval.
-
Regression Analysis: Investigates the relationship between a dependent variable and one or more independent variables. Linear regression models the relationship as a straight line, while more complex models can capture non-linear relationships.
Probability and Statistics in Engineering
Engineering applications of probability and statistics are vast:
1. Reliability Engineering
Reliability engineering uses probability models to assess the likelihood of system failure. Techniques like fault tree analysis and reliability block diagrams help identify potential failure points and estimate the overall system reliability. This is crucial in designing safe and dependable systems, such as aircraft, bridges, and power plants. Statistical methods are used to analyze failure data and estimate parameters of the probability distributions that govern component lifetimes.
2. Quality Control
Statistical process control (SPC) uses control charts to monitor manufacturing processes and detect deviations from desired quality levels. This prevents defects and ensures consistent product quality. Sampling techniques are used to efficiently assess product quality without testing every item.
3. Signal Processing
Probability and statistics are essential in signal processing, where noisy signals need to be filtered and analyzed. Techniques like Fourier analysis and wavelet transforms are used to decompose signals into their frequency components, and statistical methods help separate the signal from the noise. This is critical in applications such as image processing, communication systems, and biomedical signal analysis.
4. Structural Engineering
Structural engineers use statistical methods to model and analyze the loads and stresses on structures. Probability distributions are used to represent uncertain loads (e.g., wind, earthquakes), and statistical analysis helps assess the structural integrity and safety under these loads. This is particularly important in designing structures that must withstand extreme events.
5. Civil Engineering
In civil engineering, statistical methods are used to analyze traffic flow, predict water resource availability, and assess environmental impact. For example, probabilistic models are used to estimate the risk of flooding or predict the lifespan of infrastructure components.
Probability and Statistics in the Sciences
The application of probability and statistics in various scientific disciplines is equally extensive:
1. Experimental Design
Scientists use statistical methods to design experiments, ensuring that the results are reliable and statistically significant. This involves choosing appropriate sample sizes, controlling for confounding variables, and selecting suitable statistical tests to analyze the data.
2. Data Analysis
Statistical techniques are vital for analyzing scientific data. This may involve exploring data using descriptive statistics, identifying significant relationships using regression analysis, or testing hypotheses using appropriate statistical tests.
3. Modeling and Simulation
Probabilistic and statistical models are used to simulate complex phenomena in various scientific fields. For example, climate models use statistical methods to represent uncertain factors and predict future climate scenarios. Similarly, population models incorporate stochasticity to account for unpredictable events and estimate population dynamics.
4. Genomics and Bioinformatics
Probability and statistics play a crucial role in analyzing large genomic datasets. Statistical methods are used to identify genes associated with diseases, predict protein structures, and understand evolutionary relationships.
5. Astrophysics and Cosmology
In astronomy and cosmology, statistical methods are used to analyze astronomical data, estimate distances to celestial objects, and build cosmological models. For instance, probability distributions are used to represent the uncertainty in astronomical measurements.
Conclusion
Probability and statistics are indispensable tools for engineers and scientists. Understanding these concepts and applying appropriate techniques is crucial for designing reliable systems, analyzing experimental data, drawing valid inferences, and making informed decisions. The examples provided in this article merely scratch the surface of the vast applications of probability and statistics across these fields, emphasizing the importance of continued learning and development in these areas. Further exploration into specialized statistical methods within individual engineering and scientific disciplines is highly recommended for a deeper understanding and enhanced professional capabilities.
Latest Posts
Latest Posts
-
Label The Histology Of The Ovary Using The Hints Provided
May 09, 2025
-
What Performance Specification Does 2ghz Refer To
May 09, 2025
-
Historical Criticism Considers A Texts Relationship To The
May 09, 2025
-
Food Handlers Must Wash Their Hands Before Which Activity
May 09, 2025
-
Another Name For A Noncontingent Schedule Is A Continuous Schedule
May 09, 2025
Related Post
Thank you for visiting our website which covers about Probability And Statistics For Engineering And The Sciences . We hope the information provided has been useful to you. Feel free to contact us if you have any questions or need further assistance. See you next time and don't miss to bookmark.