Probability And Statistics For Engineers And Scientists
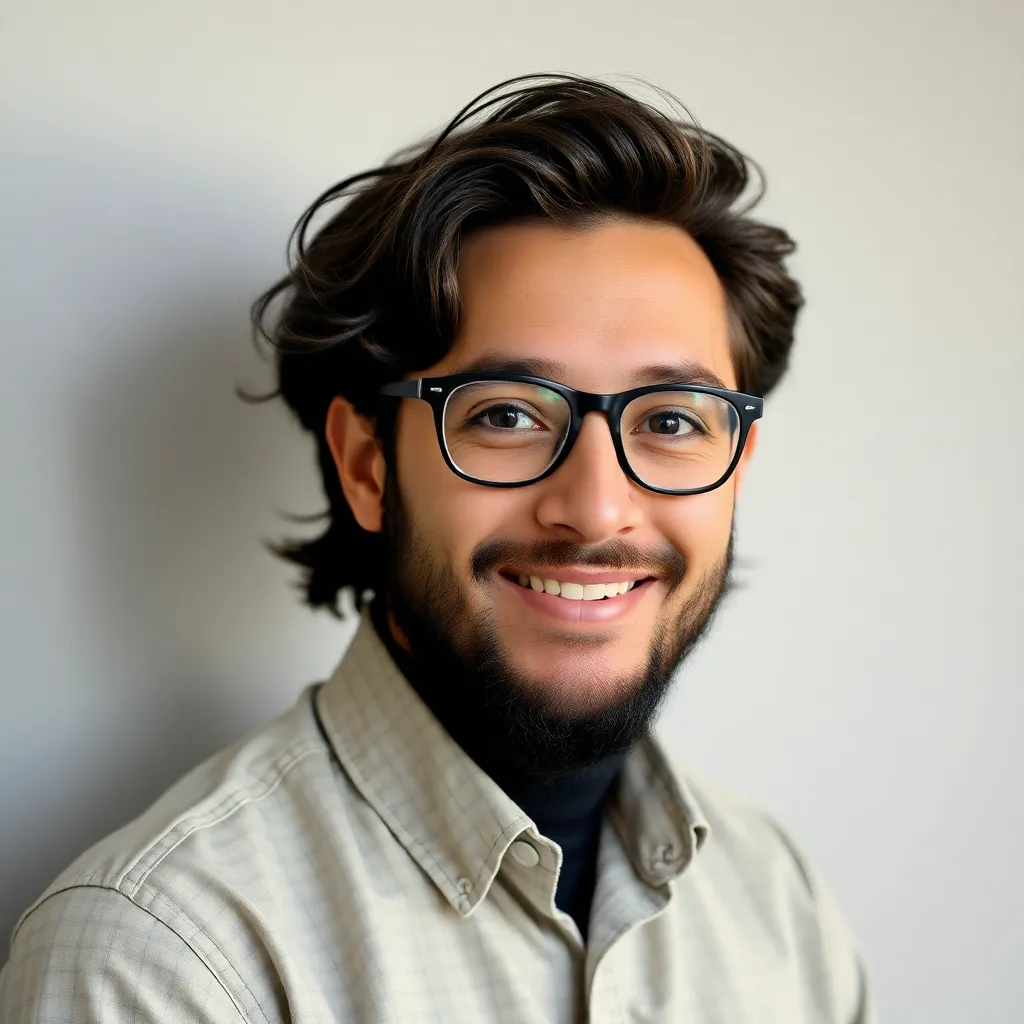
Breaking News Today
May 10, 2025 · 6 min read
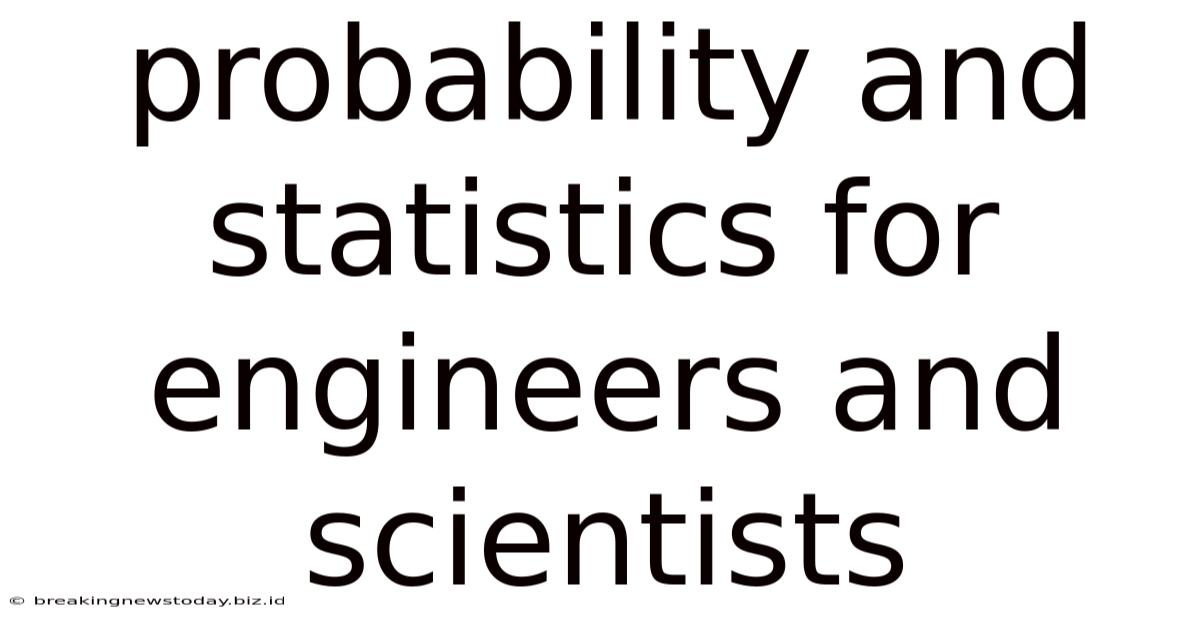
Table of Contents
Probability and Statistics for Engineers and Scientists: A Comprehensive Guide
Probability and statistics are fundamental tools for engineers and scientists across numerous disciplines. From designing reliable systems to analyzing experimental data, a solid grasp of these concepts is crucial for informed decision-making and innovative problem-solving. This comprehensive guide explores key aspects of probability and statistics, equipping you with the knowledge to effectively apply these techniques in your field.
Understanding Probability
Probability quantifies the likelihood of an event occurring. It's expressed as a number between 0 and 1, where 0 indicates impossibility and 1 indicates certainty. Several key concepts underpin the study of probability:
1. Sample Space and Events:
The sample space (S) encompasses all possible outcomes of an experiment. An event (E) is a subset of the sample space, representing a specific outcome or a collection of outcomes. For example, if we toss a coin, the sample space is {Heads, Tails}, and the event "getting heads" is {Heads}.
2. Types of Probability:
- Classical Probability: Based on equally likely outcomes. The probability of an event is the ratio of favorable outcomes to the total number of possible outcomes. For example, the probability of rolling a 6 on a fair die is 1/6.
- Empirical Probability (Relative Frequency): Determined from observed data. It's the ratio of the number of times an event occurs to the total number of trials. For instance, if a coin is tossed 100 times and heads appears 52 times, the empirical probability of getting heads is 52/100 = 0.52.
- Subjective Probability: Based on expert judgment or belief, often used when objective data is limited.
3. Probability Rules:
- Addition Rule: For mutually exclusive events (events that cannot occur simultaneously), P(A or B) = P(A) + P(B). For non-mutually exclusive events, P(A or B) = P(A) + P(B) - P(A and B).
- Multiplication Rule: For independent events (events whose occurrence doesn't affect each other), P(A and B) = P(A) * P(B). For dependent events, P(A and B) = P(A) * P(B|A), where P(B|A) is the conditional probability of B given A.
- Conditional Probability: The probability of an event occurring given that another event has already occurred. It's calculated as P(A|B) = P(A and B) / P(B).
- Bayes' Theorem: Used to revise probabilities based on new evidence. It states: P(A|B) = [P(B|A) * P(A)] / P(B).
Understanding Statistics
Statistics involves collecting, analyzing, interpreting, presenting, and organizing data to draw meaningful conclusions and make informed decisions. It's broadly categorized into descriptive and inferential statistics.
1. Descriptive Statistics:
Descriptive statistics summarize and describe the main features of a dataset. Key measures include:
-
Measures of Central Tendency:
- Mean: The average of the data.
- Median: The middle value when the data is sorted.
- Mode: The most frequent value.
-
Measures of Dispersion:
- Range: The difference between the maximum and minimum values.
- Variance: The average of the squared deviations from the mean.
- Standard Deviation: The square root of the variance, representing the spread of the data around the mean.
-
Data Visualization: Histograms, box plots, scatter plots, and other graphical representations are used to visually display data patterns and relationships.
2. Inferential Statistics:
Inferential statistics uses sample data to make inferences about a larger population. Key techniques include:
- Estimation: Estimating population parameters (like the mean or standard deviation) based on sample statistics. This involves constructing confidence intervals, which provide a range of values likely to contain the true population parameter.
- Hypothesis Testing: Testing specific claims or hypotheses about a population. This involves setting up null and alternative hypotheses, collecting data, calculating a test statistic, and determining whether to reject or fail to reject the null hypothesis based on the p-value (the probability of observing the data if the null hypothesis is true). Common tests include t-tests, ANOVA, and chi-square tests.
- Regression Analysis: Modeling the relationship between a dependent variable and one or more independent variables. Linear regression is a common technique used to fit a straight line to the data, allowing predictions to be made.
Probability Distributions
Probability distributions describe the probabilities of different outcomes for a random variable. Several key distributions are crucial for engineers and scientists:
1. Discrete Probability Distributions:
- Binomial Distribution: Models the probability of getting a certain number of successes in a fixed number of independent Bernoulli trials (trials with only two possible outcomes, like success or failure).
- Poisson Distribution: Models the probability of a certain number of events occurring in a fixed interval of time or space, when the events are rare and independent.
2. Continuous Probability Distributions:
- Normal Distribution (Gaussian Distribution): A bell-shaped distribution that's widely used to model many natural phenomena. It's characterized by its mean (μ) and standard deviation (σ).
- Exponential Distribution: Models the time between events in a Poisson process.
- Uniform Distribution: All outcomes within a given range are equally likely.
Applications in Engineering and Science
The applications of probability and statistics are vast and varied across engineering and scientific disciplines:
1. Civil Engineering:
- Structural Reliability: Assessing the probability of structural failure under various load conditions.
- Risk Assessment: Evaluating the risk associated with natural hazards (earthquakes, floods) and human-induced disasters.
- Traffic Flow Modeling: Analyzing traffic patterns and optimizing traffic management systems.
2. Mechanical Engineering:
- Quality Control: Monitoring product quality and identifying sources of defects.
- Reliability Engineering: Designing and analyzing the reliability of mechanical systems.
- Experimental Design: Planning and conducting experiments to evaluate the performance of mechanical components.
3. Electrical Engineering:
- Signal Processing: Analyzing and processing signals corrupted by noise.
- Communication Systems: Designing reliable communication systems that minimize errors.
- Control Systems: Designing control systems that maintain stability and desired performance.
4. Chemical Engineering:
- Process Control: Monitoring and controlling chemical processes to maintain desired product quality.
- Process Optimization: Optimizing chemical processes to improve efficiency and yield.
- Experimental Design: Designing experiments to study the effects of process variables on product quality.
5. Data Science and Machine Learning:
- Data Analysis: Cleaning, transforming, and analyzing large datasets.
- Model Building: Developing statistical models to predict future outcomes.
- Algorithm Evaluation: Assessing the performance of machine learning algorithms.
Conclusion
Probability and statistics are indispensable tools for engineers and scientists. A thorough understanding of these concepts allows for effective data analysis, robust system design, informed decision-making, and the ability to draw meaningful conclusions from experimental data. This guide provides a solid foundation for further exploration and application of these powerful techniques in diverse fields. Continuous learning and practical application are key to mastering probability and statistics and harnessing their full potential in your chosen domain. Remember to always critically evaluate your results and consider the limitations of your statistical methods. By combining rigorous statistical analysis with practical engineering judgment, you can significantly enhance your problem-solving capabilities and contributions to your field.
Latest Posts
Latest Posts
-
Explain The Concept Of Market And Market Identification
May 10, 2025
-
Jack Needs A Convenient Way To Browse The Internet
May 10, 2025
-
A Nurse Is Performing A Preadmission Assessment
May 10, 2025
-
When A Tax Is Levied On Buyers The
May 10, 2025
-
Which Of The Following Is An Example Of Translational Research
May 10, 2025
Related Post
Thank you for visiting our website which covers about Probability And Statistics For Engineers And Scientists . We hope the information provided has been useful to you. Feel free to contact us if you have any questions or need further assistance. See you next time and don't miss to bookmark.