Some Quantitative Data Sets Do Not Have Medians
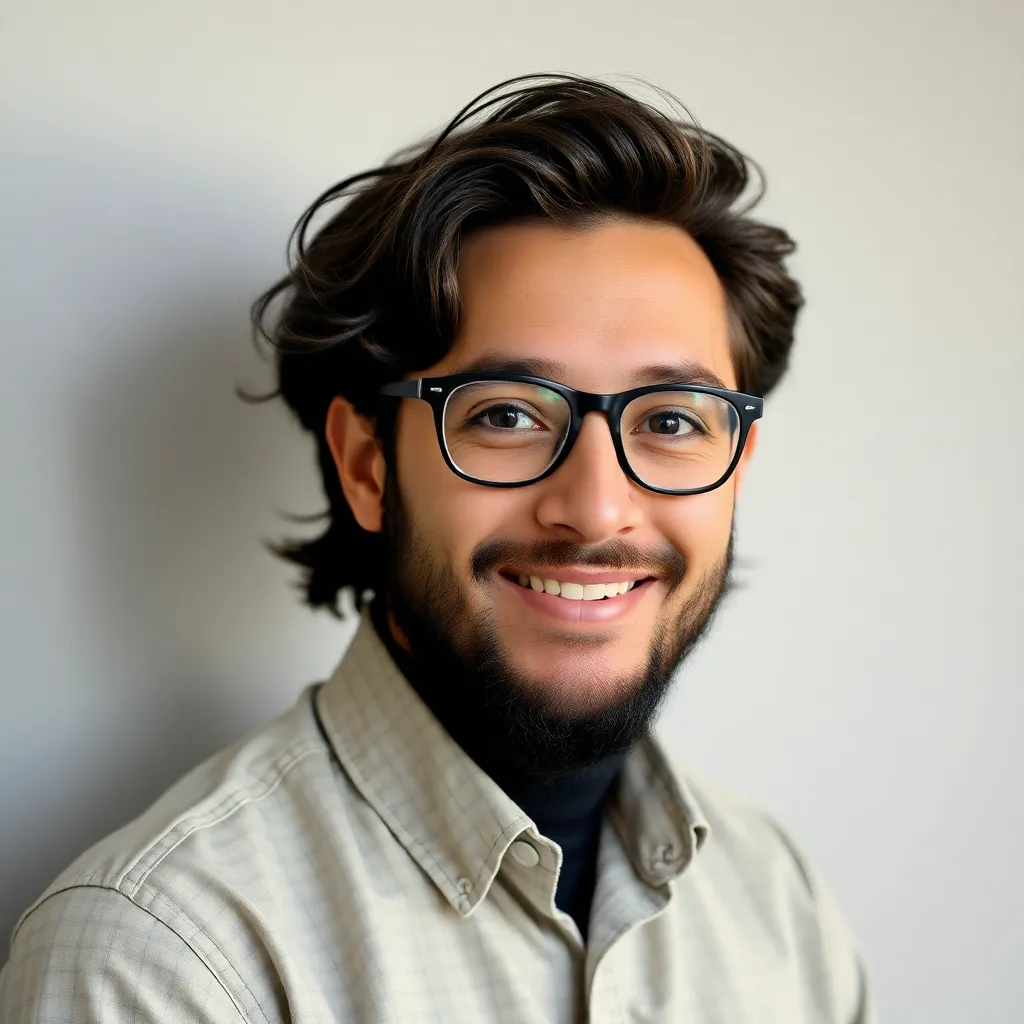
Breaking News Today
Apr 23, 2025 · 6 min read

Table of Contents
Some Quantitative Data Sets Do Not Have Medians
The median, a fundamental measure of central tendency, represents the middle value in an ordered dataset. While often straightforward to calculate, there are specific scenarios involving quantitative data where a median simply doesn't exist. Understanding these scenarios is crucial for data analysis and interpretation, preventing misleading conclusions and ensuring the application of appropriate statistical methods. This article delves into the intricacies of median calculation and explores various quantitative datasets that lack a defined median.
Understanding the Median: A Quick Recap
Before exploring the exceptions, let's briefly revisit the concept of the median. For a finite dataset, the median is the middle value when the data is arranged in ascending order. If the dataset has an odd number of observations, the median is the single middle value. If the dataset has an even number of observations, the median is the average of the two middle values.
Example 1 (Odd Number of Observations):
Dataset: 2, 5, 8, 11, 15
Median = 8
Example 2 (Even Number of Observations):
Dataset: 3, 7, 9, 12
Median = (7 + 9) / 2 = 8
This simple calculation works flawlessly for finite datasets with well-defined numerical values. However, complications arise when dealing with specific types of data and infinite datasets.
Cases Where the Median is Undefined
Several situations prevent the calculation of a median, often stemming from the nature of the data itself or its distribution. Let's examine these in detail:
1. Open-Ended Data Intervals:
Many datasets, particularly in surveys or questionnaires, use open-ended intervals. For instance, an income survey might have categories like "Under $25,000," "$25,000 - $50,000," "$50,000 - $75,000," and "Over $75,000." The "Over $75,000" category lacks an upper bound, making it impossible to definitively order all values and find a middle point. The lack of a defined upper limit for at least one interval prevents median calculation. The same applies if the lower limit is undefined (e.g., "Less than $25,000").
2. Infinite Datasets:
While the median is readily calculable for finite datasets, its application to infinite datasets is problematic. Consider a continuous probability distribution, such as the normal distribution. Theoretically, it contains an infinite number of values. There's no single "middle" value in an infinite set; therefore, the median is undefined in this context. Instead, we utilize the concept of the median of a probability distribution, which is defined as the value m such that P(X ≤ m) ≥ 0.5 and P(X ≥ m) ≥ 0.5. This median is a theoretical value representing the point that divides the distribution's area into two equal halves.
3. Datasets with Unordered Categorical Data:
The median requires numerical data that can be ranked in ascending order. Datasets with unordered categorical data, such as hair color (brown, black, blonde, red), types of cars (sedan, SUV, truck), or favorite colors, do not have a defined median. While we can calculate the mode (most frequent category), the concept of a middle value is meaningless in the absence of a numerical ordering scheme.
4. Datasets with Missing Values:
Missing data poses challenges to various statistical calculations, including the median. If a dataset has numerous missing values, especially if they are not missing completely at random (MCAR), attempting to calculate the median can be misleading. Imputation techniques (replacing missing values with estimated values) can be employed before calculating the median, but the imputed values introduce uncertainty and might affect the accuracy of the result. Therefore, the presence of extensive missing values could effectively render the median undefined or unreliable.
5. Datasets with Extreme Outliers:
While not strictly undefined, datasets with significant outliers can substantially skew the median's meaningfulness. A single extreme outlier can drastically alter the median, especially in smaller datasets. For example, if we have data points: 1, 2, 3, 4, 5, and 1000, the median is 3.5, a value heavily influenced by the outlier. In such cases, the median might not provide a robust representation of the central tendency and other robust measures of central tendency like the trimmed mean might be more appropriate.
6. Datasets with Bi-modal or Multi-modal Distributions:
Data with multiple peaks in its distribution (bi-modal or multi-modal) is not necessarily without a median, but the median's value might not effectively summarize the central tendency. In such distributions, the median might fall in a less densely populated region, unlike the mode, which represents the most frequent value(s). Using the median alongside other measures, like the mode and mean, along with visual representation using histograms, can provide a clearer understanding of the data's distribution.
Dealing with Data Sets Lacking a Median: Alternatives and Strategies
When confronted with datasets lacking a well-defined median, several strategies can be employed to analyze and interpret the data effectively:
-
Data Transformation: In some cases, transforming the data can make median calculation possible. For instance, open-ended intervals might be dealt with by assigning plausible upper or lower bounds based on domain knowledge or by grouping less common values into wider intervals. However, this approach involves assumptions which might influence the final results.
-
Robust Measures of Central Tendency: When outliers or skewed distributions affect the median, robust measures like the trimmed mean (mean after removing a certain percentage of extreme values from both ends) or the median absolute deviation (MAD) can provide more stable and representative measures of central tendency.
-
Mode or other Descriptive Statistics: When numerical ordering isn't feasible, the mode can describe the most frequent category. Additionally, other descriptive statistics, such as percentiles (25th, 75th percentiles) or quartiles, can offer insights into the data's distribution and spread even without a clear median.
-
Visualization: Histograms, box plots, and other visualizations provide crucial visual summaries of the data, revealing the distribution, presence of outliers, and the absence or unreliability of a median, without requiring the calculation of the median itself.
-
Consider the Research Question: Before choosing any measure of central tendency, carefully assess the research question. The appropriate measure depends on the research objectives and the nature of the data.
Conclusion
The median, while a valuable statistical tool, is not always applicable. Understanding the specific circumstances where a median cannot be calculated or is unreliable is crucial for accurate data analysis and meaningful interpretation. By recognizing the limitations of the median and employing alternative approaches like robust statistical measures, data transformations, or visualizations, we can obtain comprehensive insights even from complex datasets that lack a clearly defined middle value. Remember, the choice of statistical measures should always be guided by the nature of the data and the research question at hand, ensuring that the analysis remains robust and insightful.
Latest Posts
Latest Posts
-
The Aed Is Most Advantageous To The Emt Because
Apr 23, 2025
-
Multiple Sclerosis And Atherosclerosis Both Refer To
Apr 23, 2025
-
Vesicant Blister Agents Include All Of The Following Except
Apr 23, 2025
-
Conflicts Arise Between Parents And Adolescence Because
Apr 23, 2025
-
What Do Deal Of The Day Websites Offer Subscribers
Apr 23, 2025
Related Post
Thank you for visiting our website which covers about Some Quantitative Data Sets Do Not Have Medians . We hope the information provided has been useful to you. Feel free to contact us if you have any questions or need further assistance. See you next time and don't miss to bookmark.