Statistics For Business And Economics Chapter 7 Solutions
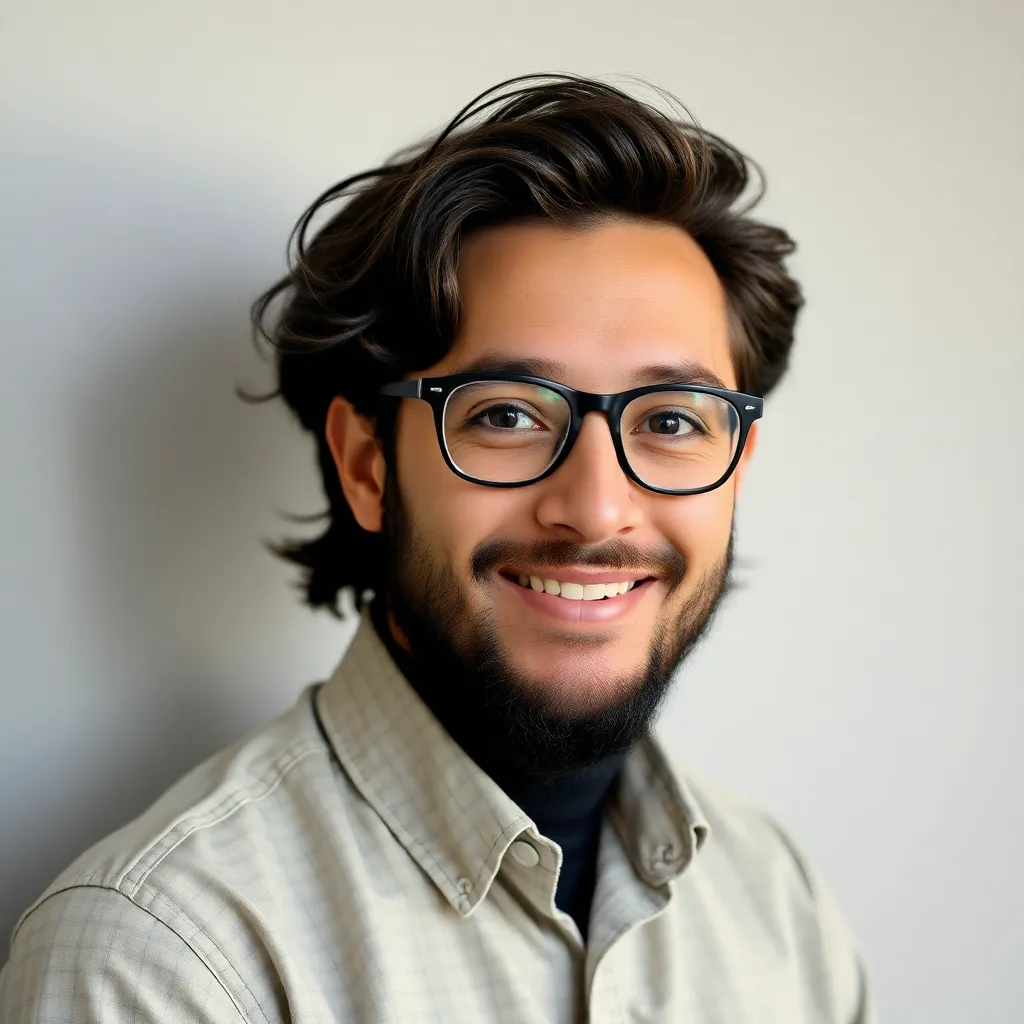
Breaking News Today
Apr 13, 2025 · 6 min read

Table of Contents
Statistics for Business and Economics: Chapter 7 Solutions – A Deep Dive
This comprehensive guide delves into the solutions for a typical Chapter 7 in a Statistics for Business and Economics textbook. While I cannot provide specific solutions tied to a particular textbook (due to copyright restrictions), I will cover the common statistical concepts and problem-solving techniques found in such chapters. This will empower you to tackle your own chapter 7 problems effectively. We will focus on building a strong understanding of the underlying principles rather than providing rote answers.
Chapter 7 Typically Covers: Hypothesis Testing
Chapter 7 in many Statistics for Business and Economics textbooks focuses on hypothesis testing. This crucial statistical technique allows us to make inferences about a population based on a sample of data. We'll explore the key elements and different types of hypothesis tests.
Understanding the Core Components of Hypothesis Testing
Before diving into specific problem-solving, let's solidify our understanding of the fundamental building blocks of hypothesis testing:
-
Null Hypothesis (H₀): This is the statement we are trying to disprove. It typically represents the status quo or a default assumption. It often states there is no significant difference or relationship. For example, "There is no difference in average income between men and women."
-
Alternative Hypothesis (H₁ or Hₐ): This is the statement we are trying to prove. It is the opposite of the null hypothesis. It suggests there is a significant difference or relationship. For example, "There is a difference in average income between men and women."
-
Significance Level (α): This is the probability of rejecting the null hypothesis when it is actually true (Type I error). It is often set at 0.05 (5%), meaning there's a 5% chance of incorrectly rejecting the null hypothesis.
-
Test Statistic: This is a calculated value based on the sample data that helps us determine whether to reject the null hypothesis. Different tests use different test statistics (e.g., t-statistic, z-statistic, F-statistic).
-
P-value: This is the probability of obtaining results as extreme as, or more extreme than, the observed results, assuming the null hypothesis is true. A low p-value (typically less than α) provides evidence against the null hypothesis.
-
Critical Region (Rejection Region): This is the range of values for the test statistic that leads to the rejection of the null hypothesis.
-
Decision Rule: Based on the p-value or the test statistic's location relative to the critical region, we decide whether to reject or fail to reject the null hypothesis.
Types of Hypothesis Tests Covered in Chapter 7
A typical Chapter 7 will cover several types of hypothesis tests. These include:
-
One-sample t-test: Used to test whether the mean of a single population differs significantly from a hypothesized value. This is useful when comparing a sample mean to a known or theoretical population mean. For example, testing if the average customer satisfaction score is above a certain target.
-
Two-sample t-test (independent samples): Used to test whether the means of two independent populations differ significantly. This is frequently used when comparing the means of two different groups (e.g., comparing the average sales of two different marketing campaigns). The test considers whether the variances are equal or unequal.
-
Paired t-test: Used to test whether the means of two related populations differ significantly. This is used when the same subjects are measured twice (e.g., before and after a treatment).
-
One-way ANOVA (Analysis of Variance): Used to test whether the means of three or more independent populations differ significantly. This is useful for comparing the means of multiple groups (e.g., comparing the average yields of different types of fertilizers).
-
Chi-square test: Used to test for the association between two categorical variables. This is useful when dealing with non-numeric data. For example, determining if there is a relationship between gender and preference for a product.
Solving Hypothesis Testing Problems: A Step-by-Step Approach
Let's outline a general approach to solving hypothesis testing problems, applicable across the various tests mentioned above:
-
State the Hypotheses: Clearly define the null and alternative hypotheses. Make sure they are mutually exclusive and exhaustive.
-
Specify the Significance Level (α): Choose a significance level (often 0.05).
-
Select the Appropriate Test: Choose the appropriate statistical test based on the type of data and the research question. Consider the number of groups being compared, the type of variables (continuous or categorical), and whether the samples are independent or paired.
-
Check Assumptions: Most statistical tests have underlying assumptions. For instance, the t-test assumes normality of data. Check these assumptions before proceeding. If assumptions are violated, consider alternative non-parametric tests.
-
Calculate the Test Statistic: Use the appropriate formula to calculate the test statistic. Software packages (like SPSS, R, or Excel) can significantly simplify this step.
-
Determine the P-value: Use statistical tables or software to determine the p-value associated with the calculated test statistic.
-
Make a Decision: Compare the p-value to the significance level (α).
-
If the p-value is less than α, reject the null hypothesis. There is sufficient evidence to support the alternative hypothesis.
-
If the p-value is greater than or equal to α, fail to reject the null hypothesis. There is not enough evidence to support the alternative hypothesis.
-
-
Interpret the Results: State your conclusion in the context of the problem. Avoid statistical jargon and clearly explain the implications of your findings.
Advanced Concepts and Extensions (Potentially Covered in Chapter 7)
Some more advanced concepts that might be included in a more extensive Chapter 7 include:
-
Type I and Type II Errors: Understanding the risks of making incorrect decisions. Type I error (false positive) is rejecting the null hypothesis when it's true. Type II error (false negative) is failing to reject the null hypothesis when it's false.
-
Power of a Test: The probability of correctly rejecting the null hypothesis when it is false. A higher power is desired. Factors influencing power include sample size, significance level, and effect size.
-
Confidence Intervals: Constructing confidence intervals around the estimated parameters can provide additional insight into the precision of the estimates.
-
Effect Size: Measuring the magnitude of the difference or relationship. A statistically significant result might have a small effect size, indicating practical insignificance.
Real-World Applications of Chapter 7 Concepts
The principles covered in Chapter 7 are widely applicable in various business and economic contexts:
-
Marketing: Analyzing the effectiveness of advertising campaigns, comparing sales figures across different regions, or assessing customer satisfaction.
-
Finance: Evaluating investment strategies, forecasting market trends, or determining the impact of economic policies.
-
Operations Management: Improving production efficiency, controlling quality, or optimizing supply chains.
-
Human Resources: Analyzing employee performance, evaluating training programs, or assessing employee satisfaction.
Conclusion
Mastering hypothesis testing, as typically covered in Chapter 7 of a Statistics for Business and Economics textbook, is fundamental for making data-driven decisions in business and economics. By understanding the core concepts, following a systematic approach to problem-solving, and considering the various types of tests, you can confidently analyze data and draw meaningful conclusions. Remember that while software can simplify calculations, a strong understanding of the underlying principles is crucial for accurate interpretation and informed decision-making. This guide provides a strong foundation to tackle the challenges within Chapter 7 and confidently apply statistical methods to real-world scenarios. Remember to consult your textbook and instructor for specific details related to your course material.
Latest Posts
Latest Posts
-
In The 1960s The Policy Referenced In The Image Was
May 09, 2025
-
The Source Of Every Sound Is Something That Is
May 09, 2025
-
Mrs Lopez Is Enrolled In A Cost Plan
May 09, 2025
-
Which Storage Facility Provides The Best Protection For Ammunition
May 09, 2025
-
Employers Are Not Required To Make Accommodations For Employees
May 09, 2025
Related Post
Thank you for visiting our website which covers about Statistics For Business And Economics Chapter 7 Solutions . We hope the information provided has been useful to you. Feel free to contact us if you have any questions or need further assistance. See you next time and don't miss to bookmark.