The Following Data Were Reported By A Corporation
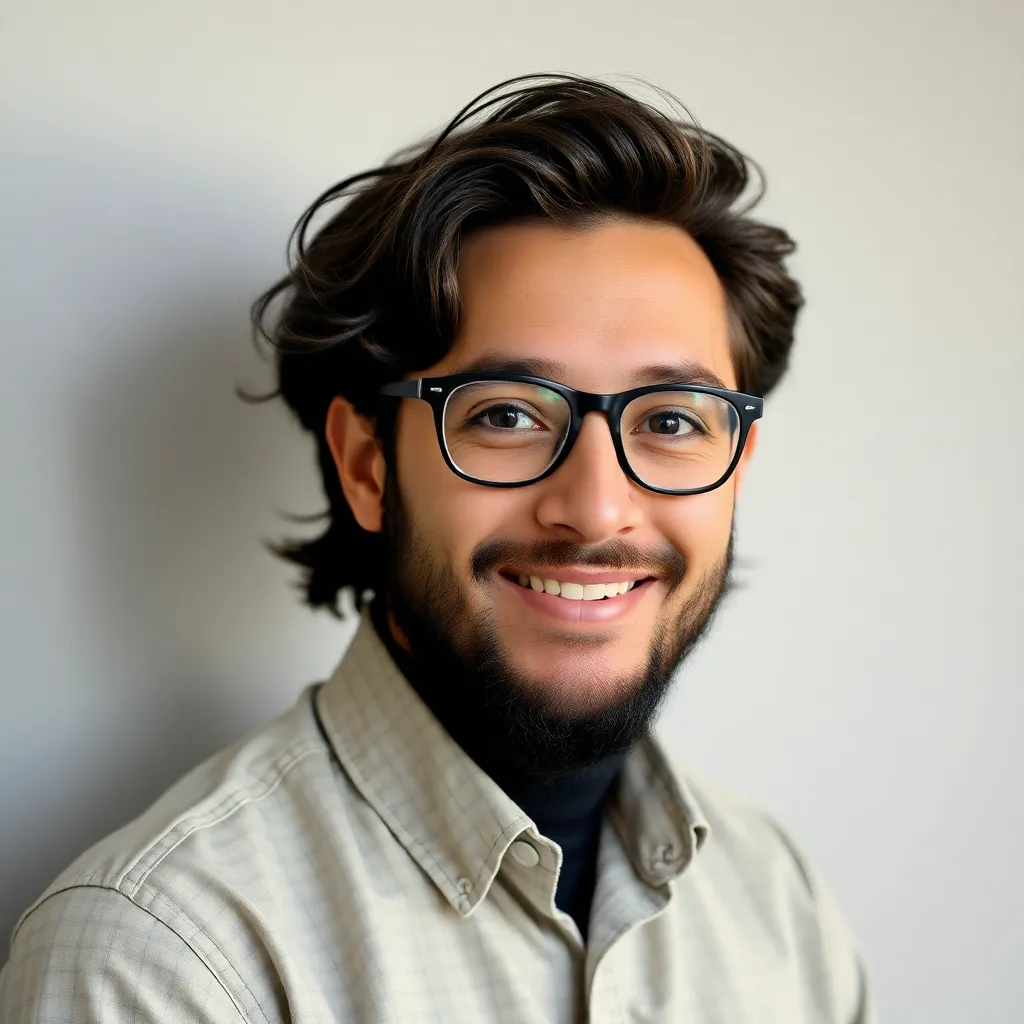
Breaking News Today
Apr 21, 2025 · 6 min read

Table of Contents
Unlocking the Power of Corporate Data: A Comprehensive Guide to Analysis and Interpretation
The sheer volume of data generated by corporations today is staggering. From sales figures and customer interactions to manufacturing processes and supply chain logistics, data points are generated constantly, offering a wealth of insights into business performance and future opportunities. However, raw data is just that – raw. Its potential remains untapped until it's analyzed, interpreted, and translated into actionable strategies. This article explores the crucial steps involved in understanding and utilizing corporate data effectively.
Understanding the Data Landscape: Types and Sources
Before diving into analysis, it's essential to grasp the different types of data corporations collect and the sources from which they originate. This knowledge forms the foundation for effective data management and interpretation.
1. Structured Data: This is organized and easily searchable data residing in relational databases. Examples include:
- Sales data: Transaction details, customer IDs, product information, and sales dates.
- Financial data: Revenue, expenses, profit margins, and cash flow.
- Inventory data: Stock levels, warehouse locations, and product movement.
- Customer relationship management (CRM) data: Customer profiles, purchase history, and communication records.
2. Unstructured Data: This data lacks a predefined format and is challenging to analyze using traditional methods. Examples include:
- Social media posts: Customer feedback, brand mentions, and sentiment analysis.
- Email communications: Customer inquiries, internal communications, and marketing campaigns.
- Customer reviews: Feedback on products, services, and overall customer experience.
- Sensor data: Real-time information from manufacturing equipment and IoT devices.
3. Semi-structured Data: This data falls between structured and unstructured data, possessing some organizational elements. Examples include:
- JSON and XML files: Data stored in a hierarchical format.
- Log files: System events and error messages.
Data Sources: Corporate data originates from various internal and external sources:
- Internal Systems: CRM systems, Enterprise Resource Planning (ERP) systems, financial systems, and operational databases.
- External Sources: Social media platforms, market research reports, industry publications, and customer surveys.
Data Cleaning and Preprocessing: Laying the Foundation for Accurate Analysis
Raw data is often incomplete, inconsistent, and contains errors. Before any meaningful analysis can be conducted, the data must undergo a thorough cleaning and preprocessing stage. This crucial step ensures the accuracy and reliability of subsequent analyses. Key aspects include:
-
Handling Missing Values: Identifying and addressing missing data points through imputation (filling in missing values based on existing data) or removal (excluding records with missing values, if appropriate). The choice depends on the extent of missing data and the potential impact on analysis.
-
Outlier Detection and Treatment: Identifying and handling extreme values (outliers) that deviate significantly from the norm. Outliers can skew results and distort interpretations. Techniques include removing outliers, transforming data (e.g., using logarithmic transformations), or using robust statistical methods that are less sensitive to outliers.
-
Data Transformation: Converting data into a suitable format for analysis. This may involve data type conversions (e.g., converting text to numerical values), standardization (scaling data to a common range), or normalization (adjusting data to a specific distribution).
-
Data Reduction: Reducing the dimensionality of the data by eliminating redundant or irrelevant variables. This improves efficiency and can simplify analysis without significant loss of information. Techniques include principal component analysis (PCA) and feature selection.
-
Data Deduplication: Identifying and removing duplicate records to prevent bias and ensure data accuracy.
Data Analysis Techniques: Unveiling Hidden Insights
Once the data is cleaned and preprocessed, various analytical techniques can be applied to extract valuable insights. The choice of technique depends on the research question and the nature of the data.
1. Descriptive Statistics: This involves summarizing and describing the main features of the data using measures such as mean, median, mode, standard deviation, and percentiles. This provides a basic understanding of the data distribution and identifies potential patterns.
2. Inferential Statistics: This involves drawing conclusions about a population based on a sample of data. Techniques include hypothesis testing, regression analysis, and ANOVA (analysis of variance). This helps to identify relationships between variables and make predictions.
3. Data Mining: This involves discovering patterns and insights from large datasets using techniques such as association rule mining, clustering, and classification. This can reveal hidden relationships and correlations that might not be apparent through simpler methods.
4. Machine Learning: This involves using algorithms to learn from data and make predictions or decisions. Techniques include regression, classification, and clustering algorithms. Machine learning can be particularly useful for predictive modeling and identifying customer behavior patterns.
5. Predictive Analytics: This uses historical data to predict future outcomes. Techniques include time series analysis, forecasting models, and machine learning algorithms. This helps in anticipating trends and making informed business decisions.
Data Visualization: Communicating Insights Effectively
Data visualization is crucial for communicating the findings of data analysis to stakeholders. Visual representations of data make complex information easily digestible and understandable, even for individuals without a technical background. Effective data visualizations include:
-
Charts and Graphs: Bar charts, line graphs, pie charts, scatter plots, and histograms are used to illustrate trends, patterns, and relationships in the data.
-
Dashboards: Interactive dashboards provide a comprehensive overview of key performance indicators (KPIs) and allow users to explore data interactively.
-
Data Storytelling: Presenting data insights in a narrative form that engages the audience and facilitates understanding. A compelling story enhances the impact of the data.
Interpreting Results and Drawing Conclusions: From Data to Action
Interpreting the results of data analysis requires careful consideration and a deep understanding of the business context. The findings must be contextualized and translated into actionable insights that can drive business decisions. This involves:
-
Identifying Key Trends and Patterns: Determining the significant findings of the analysis and their implications for the business.
-
Drawing Meaningful Conclusions: Interpreting the results in the context of the business objectives and making inferences about the data.
-
Developing Actionable Recommendations: Formulating concrete strategies based on the insights gained from the data analysis. These recommendations should be specific, measurable, achievable, relevant, and time-bound (SMART).
-
Communicating Findings Effectively: Presenting the results and recommendations to stakeholders in a clear, concise, and persuasive manner. Data visualization plays a crucial role in this communication process.
Data Security and Privacy: Ethical Considerations
Handling corporate data responsibly is paramount. Protecting data security and respecting user privacy are critical ethical considerations. This involves:
-
Data Encryption: Protecting data from unauthorized access through encryption techniques.
-
Access Control: Limiting access to sensitive data to authorized personnel only.
-
Compliance with Regulations: Adhering to relevant data privacy regulations, such as GDPR and CCPA.
-
Data Anonymization: Protecting user privacy by removing or masking personally identifiable information.
The Future of Corporate Data Analysis: Emerging Trends
The field of corporate data analysis is constantly evolving. Several emerging trends are shaping the future of this critical business function:
-
Big Data Analytics: Handling and analyzing massive datasets using advanced techniques and technologies.
-
Artificial Intelligence (AI) and Machine Learning (ML): Leveraging AI and ML for automated insights generation, predictive modeling, and decision-making.
-
Cloud Computing: Storing and processing data in the cloud to improve scalability and accessibility.
-
Internet of Things (IoT): Integrating data from connected devices to gain real-time insights into operations and customer behavior.
By effectively leveraging the power of corporate data, businesses can gain a competitive edge, improve operational efficiency, enhance customer experiences, and drive sustainable growth. The journey from raw data to actionable insights requires a multi-faceted approach, encompassing data cleaning, sophisticated analysis techniques, compelling visualization, and a responsible approach to data security and privacy. The future of successful corporations will increasingly rely on their ability to harness the power of their data.
Latest Posts
Latest Posts
-
Which Combining Form Means Plaque Or Fatty Substance
Apr 21, 2025
-
Suturing Together The Ends Of A Severed Nerve
Apr 21, 2025
-
Which Of The Following Statistics Can Turn Negative
Apr 21, 2025
-
Which Of The Following Activate Cd8 Cells
Apr 21, 2025
-
Inattentional Blindness Can Best Be Described As
Apr 21, 2025
Related Post
Thank you for visiting our website which covers about The Following Data Were Reported By A Corporation . We hope the information provided has been useful to you. Feel free to contact us if you have any questions or need further assistance. See you next time and don't miss to bookmark.