The Practice Of Statistics 6th Edition Answers
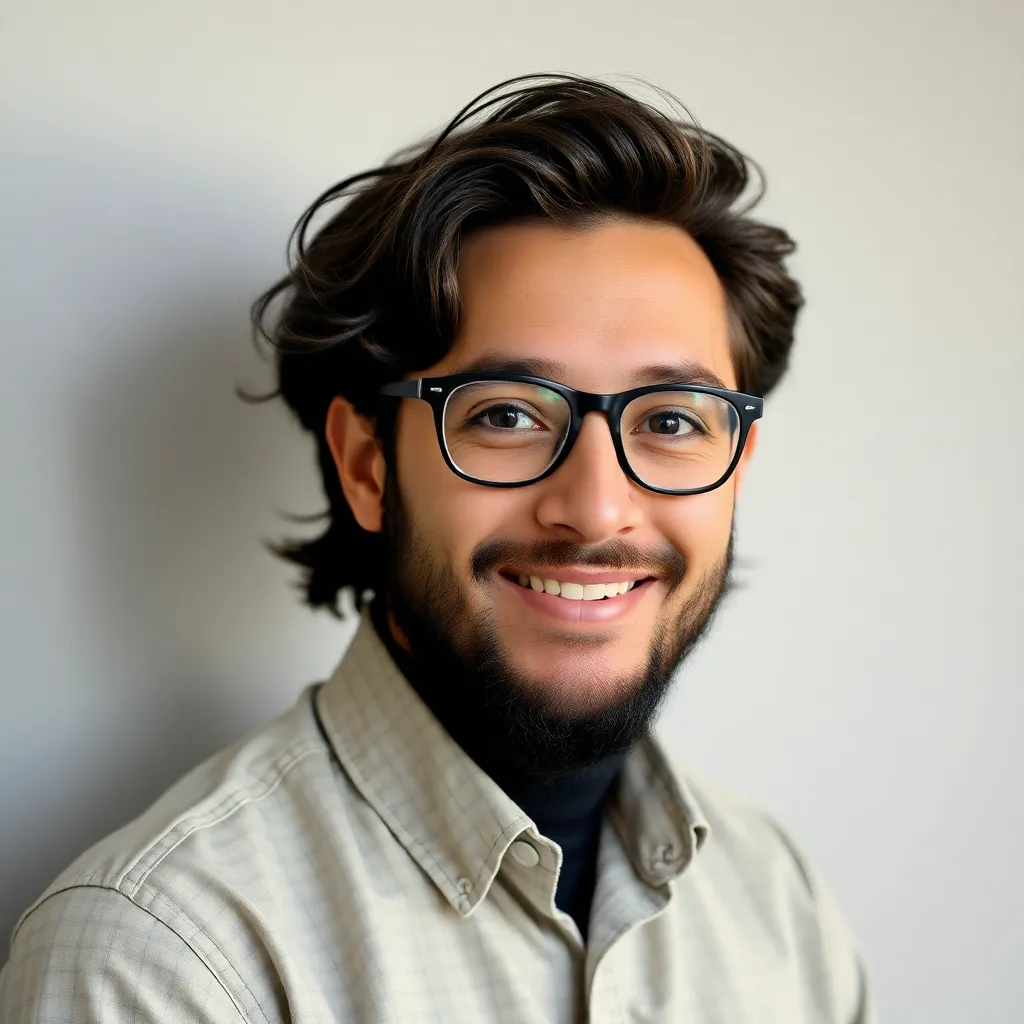
Breaking News Today
Mar 29, 2025 · 7 min read
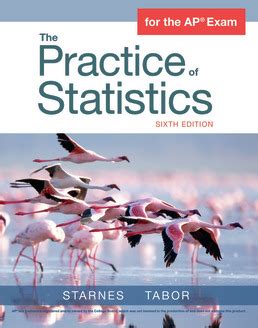
Table of Contents
The Practice of Statistics, 6th Edition: A Comprehensive Guide to Mastering Statistical Concepts and Problem Solving
The sixth edition of "The Practice of Statistics" remains a cornerstone text for introductory statistics courses. Its focus on conceptual understanding and problem-solving makes it both challenging and rewarding for students. This guide will delve into key concepts within the text, offering a deeper understanding of statistical principles and solutions to common problem areas. We will explore various statistical methods, offering detailed explanations and practical applications. Remember, while this guide provides valuable insights, active engagement with the textbook and practice problems is crucial for true mastery.
Chapter Breakdown and Key Concepts (Note: Specific chapter titles and content may vary slightly based on the specific version of the 6th edition).
This section will not provide direct answers to specific problems from the textbook, as that would defeat the purpose of learning. Instead, it will highlight crucial concepts within each chapter, equip you with the tools to solve problems independently, and guide your approach to statistical problem-solving. Remember to always refer to your textbook for detailed explanations and examples.
Chapter 1: Exploring Data
This foundational chapter introduces descriptive statistics, focusing on methods to summarize and present data effectively. Key concepts include:
- Variables: Understanding the distinction between categorical and quantitative variables is fundamental. Categorical variables describe qualities (e.g., eye color), while quantitative variables measure numerical quantities (e.g., height).
- Distributions: Learning to interpret histograms, stemplots, and boxplots is crucial for visualizing data patterns. Recognizing symmetry, skewness, and the presence of outliers is vital for a complete analysis.
- Measures of Center: Mean, median, and mode represent different aspects of central tendency. Understanding when each measure is appropriate is essential. Consider the impact of outliers on each measure.
- Measures of Spread: Range, interquartile range (IQR), and standard deviation quantify the variability within a dataset. These measures provide a crucial context to the measures of center.
Chapter 2: Summarizing Data
Building upon the previous chapter, this section often dives deeper into summarizing and interpreting data distributions. Crucial elements include:
- Five-Number Summary: The minimum, first quartile (Q1), median (Q2), third quartile (Q3), and maximum provide a concise summary of a dataset's distribution. This is particularly useful when constructing boxplots.
- Standard Deviation: Understanding the calculation and interpretation of standard deviation is paramount. It measures the typical distance of data points from the mean.
- Z-Scores: Z-scores standardize data, allowing comparison across different distributions. They measure how many standard deviations a data point is from the mean. Mastering Z-scores is key for hypothesis testing later in the book.
- Transforming Data: This section often covers methods for transforming data to achieve normality or symmetry, a common prerequisite for many statistical analyses.
Chapter 3: Probability
Probability forms the theoretical foundation of inferential statistics. Key concepts include:
- Basic Probability Rules: Understanding the addition rule, multiplication rule (both independent and dependent events), and complementary events is fundamental.
- Conditional Probability: This is a critical concept involving the probability of an event given that another event has occurred. Bayes' theorem is often introduced here.
- Random Variables: Understanding discrete and continuous random variables lays the groundwork for probability distributions.
- Probability Distributions: This section typically covers the binomial and normal distributions, which are frequently used in hypothesis testing and confidence intervals.
Chapter 4: Random Variables
This chapter deepens the understanding of probability distributions:
- Discrete vs. Continuous: Solidify your understanding of the differences between discrete and continuous random variables and their corresponding probability distributions.
- Expected Value and Variance: Learning to calculate and interpret the expected value (mean) and variance of a random variable is crucial.
- Binomial Distribution: Understanding the conditions for a binomial experiment and calculating binomial probabilities is essential. The binomial distribution models the probability of success in a fixed number of independent trials.
- Normal Distribution: The normal distribution is a cornerstone of statistics. Mastering its properties (symmetry, mean = median = mode) and using Z-scores for calculations is vital. Understanding the empirical rule (68-95-99.7 rule) is helpful for quick estimations.
Chapter 5: Sampling Distributions
This chapter bridges the gap between descriptive and inferential statistics.
- Sampling Variability: Understanding that different samples from the same population will produce different statistics is crucial. This variability is captured by the sampling distribution.
- Sampling Distribution of the Sample Mean: This is the distribution of sample means from all possible samples of a given size. It is typically approximately normal, even if the population distribution is not (Central Limit Theorem).
- Central Limit Theorem (CLT): This is arguably the most important theorem in introductory statistics. The CLT states that the sampling distribution of the sample mean becomes approximately normal as the sample size increases, regardless of the population distribution.
- Standard Error: The standard deviation of the sampling distribution is called the standard error. It measures the variability of sample means.
Chapter 6: Estimating with Confidence
This chapter introduces confidence intervals, a crucial tool for estimating population parameters.
- Confidence Intervals: Understanding the concept of a confidence interval and interpreting its meaning is essential. It provides a range of plausible values for a population parameter.
- Margin of Error: The margin of error quantifies the uncertainty associated with the estimate.
- Choosing Sample Size: This section often covers methods for determining the necessary sample size to achieve a desired margin of error and confidence level.
- Conditions for Inference: Always check the conditions (random sampling, large sample size, etc.) before constructing a confidence interval.
Chapter 7: Testing a Hypothesis
Hypothesis testing is a core concept in inferential statistics.
- Null and Alternative Hypotheses: Clearly defining the null and alternative hypotheses is the first step in hypothesis testing.
- P-values: The p-value is the probability of observing the data (or more extreme data) if the null hypothesis is true. A small p-value provides evidence against the null hypothesis.
- Significance Level (alpha): The significance level sets the threshold for rejecting the null hypothesis. Commonly, alpha = 0.05.
- Types of Errors: Understanding Type I and Type II errors is crucial. A Type I error is rejecting a true null hypothesis, and a Type II error is failing to reject a false null hypothesis.
- One-Sample t-test: Used to compare a sample mean to a known population mean when the population standard deviation is unknown.
- Two-Sample t-test: Used to compare the means of two independent samples.
Chapter 8: Comparing Two Groups
This chapter extends hypothesis testing to compare two groups.
- Two-Sample t-test (Independent Samples): Used to compare the means of two independent groups.
- Paired t-test: Used to compare the means of two dependent groups (e.g., before-and-after measurements).
- Conditions for Inference: Always check the necessary conditions before performing these tests (randomization, independence, normality).
Chapter 9: Relationships Between Variables
This chapter explores relationships between variables using regression analysis.
- Scatterplots: Used to visualize the relationship between two quantitative variables.
- Correlation: Measures the strength and direction of a linear relationship.
- Regression: Used to model the relationship between a response variable and one or more explanatory variables.
- Least Squares Regression Line: The line that minimizes the sum of squared residuals.
- Interpreting Regression Coefficients: Understanding the meaning of the slope and intercept in a regression equation.
- Coefficient of Determination (R-squared): Represents the proportion of variance in the response variable explained by the explanatory variable(s).
Chapter 10: Analyzing Categorical Data
This chapter introduces methods for analyzing categorical data.
- Contingency Tables: Used to summarize the relationship between two categorical variables.
- Chi-Square Test: Used to test for independence between two categorical variables.
Chapter 11: More about Regression (Optional in some editions)
This chapter might delve deeper into regression analysis, covering topics such as:
- Multiple Regression: Extends simple linear regression to include multiple explanatory variables.
- Model Assumptions: Understanding and checking the assumptions of regression models (linearity, independence, constant variance, normality).
- Influential Points: Identifying and handling data points that have a strong influence on the regression results.
Chapter 12: Analysis of Variance (ANOVA) (Optional in some editions)
This chapter introduces ANOVA, a powerful technique for comparing the means of three or more groups.
This expanded guide provides a detailed overview of the key concepts covered in "The Practice of Statistics, 6th Edition." Remember to consult your textbook for detailed explanations, examples, and further practice problems. Active engagement with the material and consistent practice are key to mastering the concepts presented. Good luck with your studies!
Latest Posts
Latest Posts
-
Which Of The Following Is Not A Function Of Skin
Mar 31, 2025
-
Anything That Takes Up Space And Has Mass
Mar 31, 2025
-
An Example Of A Latent Viral Infection Is Quizlet
Mar 31, 2025
-
Why Are Olfaction And Gustation Called Chemical Senses
Mar 31, 2025
-
How Does International Trade Lead To Specialization Quizlet
Mar 31, 2025
Related Post
Thank you for visiting our website which covers about The Practice Of Statistics 6th Edition Answers . We hope the information provided has been useful to you. Feel free to contact us if you have any questions or need further assistance. See you next time and don't miss to bookmark.