The Process Of Simultaneously Analyzing The Behavior Of Individuals
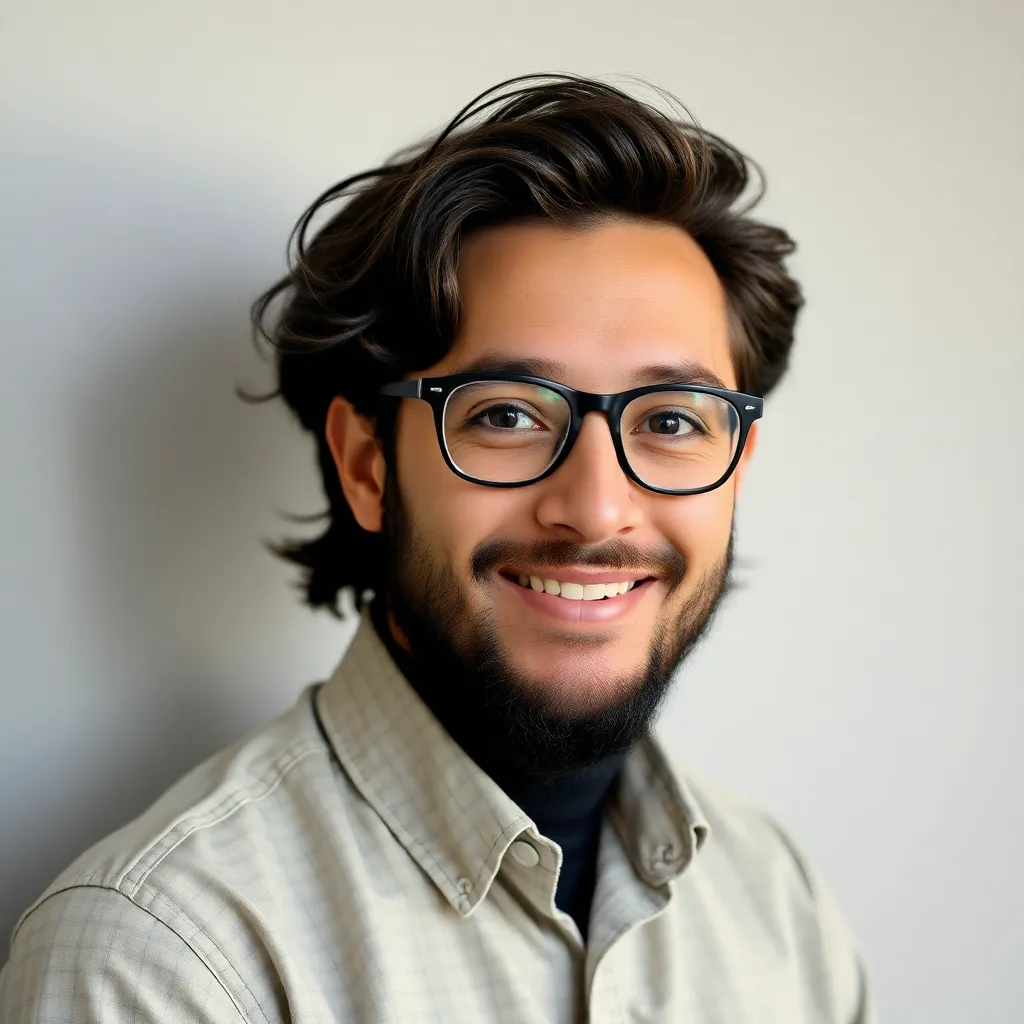
Breaking News Today
Apr 17, 2025 · 6 min read

Table of Contents
The Complexities of Simultaneously Analyzing Individual Behavior: A Deep Dive into Methods and Applications
Simultaneously analyzing the behavior of individuals presents a significant challenge across various fields, from social sciences and marketing to healthcare and security. Understanding the intricate dynamics of group behavior requires sophisticated methodologies capable of capturing individual actions and interactions within a shared context. This article delves into the intricacies of this process, exploring the diverse techniques employed, their inherent limitations, and their impactful applications across numerous domains.
The Challenges of Simultaneous Behavioral Analysis
The core difficulty lies in the inherent complexity of human behavior. Individuals aren't isolated entities; their actions are profoundly influenced by the social context, group dynamics, and the presence of others. Analyzing individuals simultaneously demands a framework that accounts for:
- Individual Differences: People possess unique personalities, motivations, and cognitive styles. A successful analysis must account for this heterogeneity, recognizing that individuals respond differently to the same stimuli.
- Interdependence: Actions often trigger reciprocal responses. One person's behavior can influence another, creating a cascade effect that complicates simple cause-and-effect relationships.
- Contextual Factors: The environment plays a vital role. Analyzing behavior in isolation neglects crucial contextual cues like time, location, and the nature of the interaction.
- Data Volume and Complexity: Simultaneously tracking multiple individuals generates massive datasets requiring efficient processing and analytical techniques. Managing this volume of information poses significant computational and analytical hurdles.
- Ethical Considerations: Collecting and analyzing behavioral data raises critical ethical concerns related to privacy, informed consent, and the potential for bias or misinterpretation.
Methodologies for Simultaneous Behavioral Analysis
Several approaches attempt to tackle the challenges of simultaneous behavioral analysis. These methodologies often involve a combination of quantitative and qualitative techniques:
1. Ethnographic Studies and Qualitative Analysis: This approach emphasizes deep immersion in the natural setting, observing individuals and their interactions within their natural environment. Researchers employ techniques like participant observation, interviews, and focus groups to gather rich, qualitative data. The analysis focuses on identifying patterns, themes, and shared meanings within the observed behaviors. While rich in detail, qualitative approaches often struggle with generalizability and rigorous quantitative analysis.
2. Network Analysis: This method focuses on the relationships between individuals, mapping interactions and influence flows within a group. Social network analysis (SNA) uses graph theory to visualize and quantify relationships, revealing central figures, clusters, and the overall structure of the social network. SNA can identify key influencers, diffusion patterns, and the spread of information or behaviors within a group.
3. Agent-Based Modeling (ABM): ABM is a computational approach that simulates individual behavior and its impact on the overall system. Researchers define rules governing individual agents' actions, then run simulations to observe emergent group patterns. ABM allows for exploring "what-if" scenarios and testing hypotheses about the interplay between individual and collective behavior. However, the accuracy of ABM simulations hinges heavily on the realism of the agent rules and the underlying assumptions.
4. Time-Series Analysis: When dealing with longitudinal data – repeated observations over time – time-series analysis is essential. This methodology tracks changes in individual behavior over time, identifying trends, patterns, and correlations between different variables. Techniques such as autoregressive integrated moving average (ARIMA) models and other time series approaches help uncover temporal dependencies in behavior.
5. Machine Learning and Data Mining: The vast amounts of data generated by simultaneous behavioral observation often necessitate machine learning techniques. Algorithms like clustering, classification, and prediction can identify patterns and predict future behaviors based on past observations. This approach can handle high-dimensional data and identify complex relationships that might be missed by human observation. However, overreliance on machine learning can lead to "black box" effects, obscuring the underlying mechanisms driving the observed behaviors.
Applications Across Diverse Fields
Simultaneous behavioral analysis finds application in diverse fields:
1. Marketing and Consumer Behavior: Analyzing shopper behavior in retail stores, online browsing patterns, and social media interactions helps marketers understand consumer preferences, segment markets effectively, and personalize product recommendations. This allows for targeted advertising and product development tailored to specific consumer segments.
2. Healthcare: Studying patient interactions in hospital settings or analyzing telehealth data allows for a better understanding of patient needs, improving care coordination and treatment efficacy. Analyzing the spread of infectious diseases requires tracking individual contacts and movements to contain outbreaks efficiently.
3. Security and Surveillance: Analyzing crowd behavior in public spaces helps security personnel predict potential risks, manage crowd flow, and respond effectively to emergencies. In cybersecurity, monitoring user activity across multiple systems can identify anomalous behavior and potential security breaches.
4. Social Sciences: Researching group dynamics, social influence, and collective decision-making requires observing and analyzing simultaneous interactions within social groups. This allows for deeper insights into how social norms, attitudes, and beliefs influence individual and collective behavior.
5. Urban Planning: Analyzing pedestrian and vehicular traffic patterns in urban environments informs effective city planning, optimizing infrastructure design, and improving overall urban functionality.
Ethical Considerations and Responsible Data Handling
The power of simultaneously analyzing individual behavior comes with substantial ethical responsibilities:
- Data Privacy: Gathering behavioral data requires strict adherence to privacy regulations and ethical guidelines. Anonymization techniques and secure data storage are crucial to protect individual identities.
- Informed Consent: Individuals should be fully informed about the purpose of data collection and their rights regarding their data. Obtaining informed consent before data collection is essential.
- Bias and Fairness: Analyzing behavioral data can inadvertently amplify existing biases. Careful consideration must be given to potential biases in data collection, analysis, and interpretation.
- Transparency and Accountability: Methods and findings should be transparent and clearly documented, allowing for scrutiny and accountability.
- Data Security: Robust security measures are necessary to prevent data breaches and unauthorized access to sensitive information.
Future Directions and Advancements
The field of simultaneous behavioral analysis is rapidly evolving, driven by technological advancements and the increasing availability of data:
- Wearable Sensors and IoT Devices: Advances in wearable technology and the Internet of Things (IoT) provide opportunities for continuous and unobtrusive data collection, generating richer and more comprehensive behavioral datasets.
- Advanced Analytics and AI: The application of more sophisticated machine learning algorithms and artificial intelligence (AI) will enable more accurate and insightful analysis of complex behavioral patterns.
- Multimodal Data Integration: Combining data from various sources (e.g., video, audio, physiological signals) will lead to a more holistic understanding of individual behavior.
- Ethical Frameworks and Guidelines: Developing robust ethical frameworks and guidelines for data collection, analysis, and interpretation will be crucial for responsible innovation in this field.
In conclusion, the simultaneous analysis of individual behavior presents both significant challenges and opportunities. The methods described above offer powerful tools for understanding complex social dynamics across various disciplines. However, navigating the ethical considerations and employing rigorous methodologies are paramount to ensuring responsible innovation and the ethical use of this powerful analytical approach. The future of this field lies in combining advanced technologies with ethical frameworks, leading to more accurate, insightful, and responsible analysis of human behavior.
Latest Posts
Latest Posts
-
In The Third Party Payment System The Provider Is The
Apr 19, 2025
-
The Term Imaginary Audience Refers To Adolescents
Apr 19, 2025
-
Trying To Get Things Perfect Takes Time
Apr 19, 2025
-
For A Health Plan What Are The Possible Consequences
Apr 19, 2025
-
The Horizontal Rows Of The Periodic Table Are Called
Apr 19, 2025
Related Post
Thank you for visiting our website which covers about The Process Of Simultaneously Analyzing The Behavior Of Individuals . We hope the information provided has been useful to you. Feel free to contact us if you have any questions or need further assistance. See you next time and don't miss to bookmark.