The Sampling Distribution Of The Mean Helps Us ______
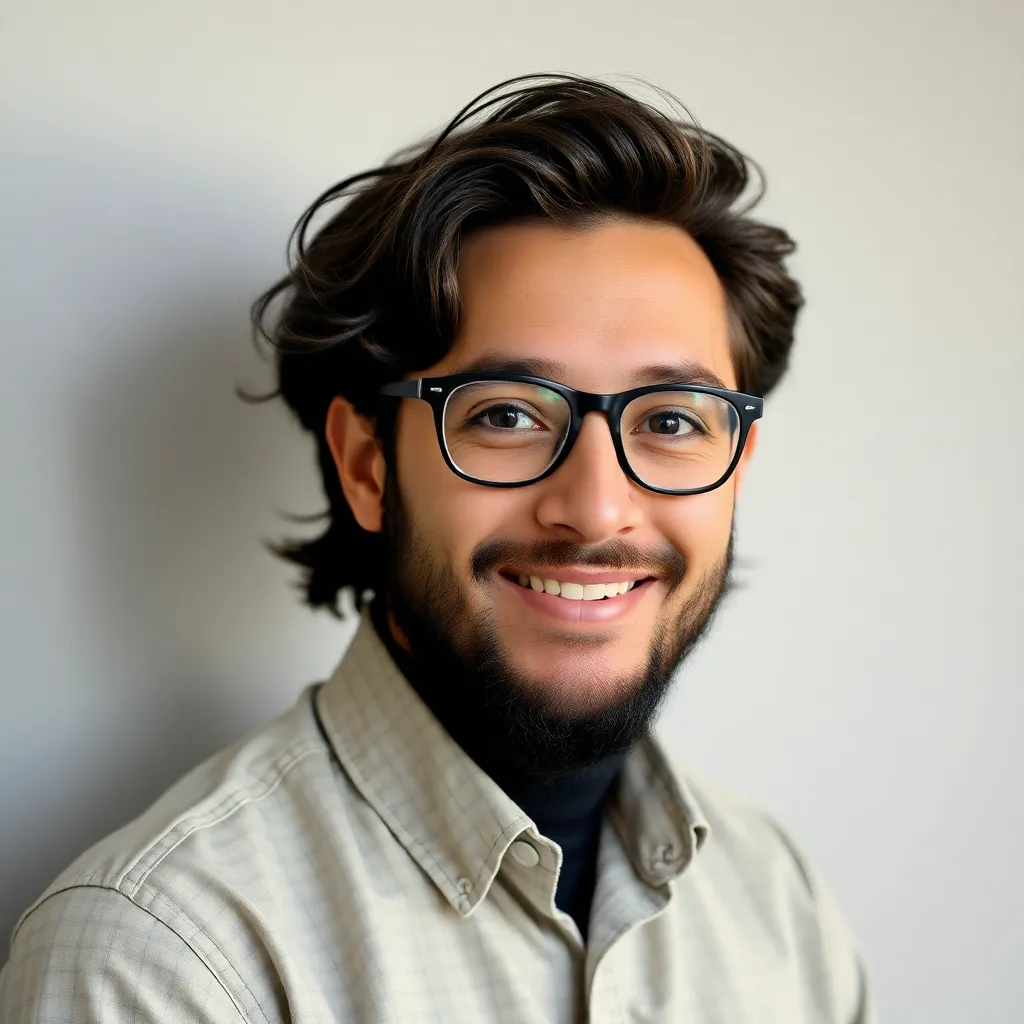
Breaking News Today
Apr 26, 2025 · 7 min read

Table of Contents
The Sampling Distribution of the Mean Helps Us Understand Population Parameters
The sampling distribution of the mean is a fundamental concept in statistics that bridges the gap between sample data and population parameters. It helps us understand population parameters by providing a framework for making inferences about a population based on information gathered from a sample. This understanding allows us to draw conclusions, make predictions, and test hypotheses with a quantifiable level of confidence. In short, the sampling distribution of the mean helps us estimate, infer, and test.
What is the Sampling Distribution of the Mean?
Before delving into its uses, let's solidify our understanding of what exactly the sampling distribution of the mean is. Imagine you have a large population – say, the heights of all adults in a country. Measuring the height of every single adult is practically impossible. Instead, we take multiple random samples from this population, each sample consisting of a certain number of individuals. For each sample, we calculate the mean height. Now, if we plotted all these sample means on a graph, the resulting distribution is the sampling distribution of the mean.
This distribution itself has its own characteristics: a mean (denoted as μ<sub>x̄</sub>) and a standard deviation (denoted as σ<sub>x̄</sub>, also known as the standard error of the mean). Crucially, the central limit theorem dictates that as the sample size increases, this sampling distribution of the mean will approach a normal distribution, regardless of the shape of the original population distribution. This is a powerful result that forms the basis for many statistical inferences.
Key Characteristics of the Sampling Distribution of the Mean
-
Center: The mean of the sampling distribution of the mean (μ<sub>x̄</sub>) is equal to the population mean (μ). This means that the average of all the sample means is equal to the true population mean.
-
Spread: The standard deviation of the sampling distribution of the mean (σ<sub>x̄</sub>) is equal to the population standard deviation (σ) divided by the square root of the sample size (n): σ<sub>x̄</sub> = σ/√n. This is the standard error of the mean, and it indicates how much the sample means vary from the population mean. A larger sample size leads to a smaller standard error, meaning the sample means are clustered more tightly around the population mean.
-
Shape: As the sample size (n) increases, the sampling distribution of the mean approaches a normal distribution, even if the original population distribution isn't normal. This is the essence of the central limit theorem, a cornerstone of statistical inference. For smaller sample sizes drawn from non-normal populations, the shape might deviate from normality, but the approximation to normality improves as the sample size grows.
How the Sampling Distribution of the Mean Helps Us
The sampling distribution of the mean is not just a theoretical concept; it's a powerful tool with several practical applications:
1. Estimating Population Parameters
One of the most significant ways the sampling distribution helps us is by providing a framework for estimating population parameters like the mean. Since it's often impractical or impossible to measure the entire population, we rely on samples. The sample mean (x̄) serves as a point estimate of the population mean (μ). However, we know this point estimate is likely to be slightly off from the true population mean. The sampling distribution tells us how much it's likely to be off. The standard error (σ<sub>x̄</sub>) quantifies this uncertainty. We can use this information to construct confidence intervals, which provide a range of values within which the true population mean likely lies with a specified level of confidence (e.g., 95% confidence interval).
2. Hypothesis Testing
Hypothesis testing involves making a statement (a hypothesis) about a population parameter and then using sample data to determine whether there is enough evidence to reject that statement. The sampling distribution of the mean is crucial here. We use it to calculate a test statistic (like a z-score or t-score), which measures how many standard errors the sample mean is from the hypothesized population mean. This test statistic is then used to determine the p-value, representing the probability of observing the obtained sample mean (or a more extreme value) if the null hypothesis (the statement being tested) were true. A small p-value (typically less than 0.05) indicates strong evidence against the null hypothesis, leading us to reject it in favor of an alternative hypothesis.
3. Comparing Population Means
The sampling distribution also allows us to compare the means of two or more populations. For example, we might want to compare the average income of men and women in a particular profession. By taking samples from both populations and constructing their respective sampling distributions of the mean, we can perform a hypothesis test (like an independent samples t-test) to see if there is a statistically significant difference between the population means. The sampling distribution provides the framework for determining the probability of observing the difference in sample means if there were no actual difference in the population means.
4. Understanding Sampling Variability
The sampling distribution highlights the inherent variability in sample statistics. Even if we take multiple random samples from the same population, we'll get different sample means each time. This is natural sampling variability. The sampling distribution shows us the pattern of this variability, allowing us to quantify how much our sample mean might differ from the true population mean due to random chance. This understanding is essential for making informed interpretations of our findings and avoiding overconfidence in results based on a single sample.
5. Power Analysis
Before conducting a study, power analysis is crucial to determine the necessary sample size to detect a meaningful effect if it exists. The sampling distribution plays a central role in power analysis. By specifying the desired level of significance (alpha), the effect size (the magnitude of the difference we want to detect), and the desired power (the probability of correctly rejecting the null hypothesis when it is false), we can calculate the minimum sample size needed to achieve the desired power. A larger sample size leads to a smaller standard error, increasing the power of the test, and allowing us to detect smaller effects with higher confidence.
Implications and Applications Across Disciplines
The power of the sampling distribution of the mean extends far beyond theoretical statistics. Its application is widespread across various fields:
-
Medical Research: Determining the effectiveness of a new drug by comparing the average improvement in a treatment group versus a control group relies heavily on the sampling distribution.
-
Social Sciences: Analyzing survey data to understand public opinion on social issues uses the sampling distribution to make inferences about the entire population based on a representative sample.
-
Business and Economics: Forecasting sales, analyzing market trends, and evaluating the effectiveness of marketing campaigns all involve utilizing the sampling distribution to draw conclusions from sample data.
-
Environmental Science: Monitoring changes in pollution levels, analyzing wildlife populations, and studying climate change all involve data analysis methods rooted in the sampling distribution.
-
Engineering: Quality control processes often rely on sampling data to assess whether products meet specified standards. The sampling distribution helps determine the acceptable range of variation.
Conclusion: A Cornerstone of Statistical Inference
The sampling distribution of the mean is a pivotal concept in statistics, acting as a crucial bridge between sample data and population parameters. Its properties allow us to estimate population parameters with quantified uncertainty, conduct hypothesis tests to make inferences about populations, and compare population means. Understanding the sampling distribution empowers us to draw meaningful conclusions from limited data, making it an indispensable tool for researchers and analysts across a wide range of disciplines. The ability to understand and apply the principles of sampling distribution is key to effectively interpreting data and making sound, data-driven decisions. The central limit theorem, underpinning the sampling distribution, provides a foundation for many statistical procedures, making it a cornerstone of modern statistical inference. As we've seen, its applications are vast and essential for navigating the complexities of data analysis in diverse fields. Mastering this concept enhances the ability to accurately interpret results, make robust predictions, and contribute meaningfully to evidence-based decision-making.
Latest Posts
Related Post
Thank you for visiting our website which covers about The Sampling Distribution Of The Mean Helps Us ______ . We hope the information provided has been useful to you. Feel free to contact us if you have any questions or need further assistance. See you next time and don't miss to bookmark.