The Standard Deviation Is Used In Conjunction With The
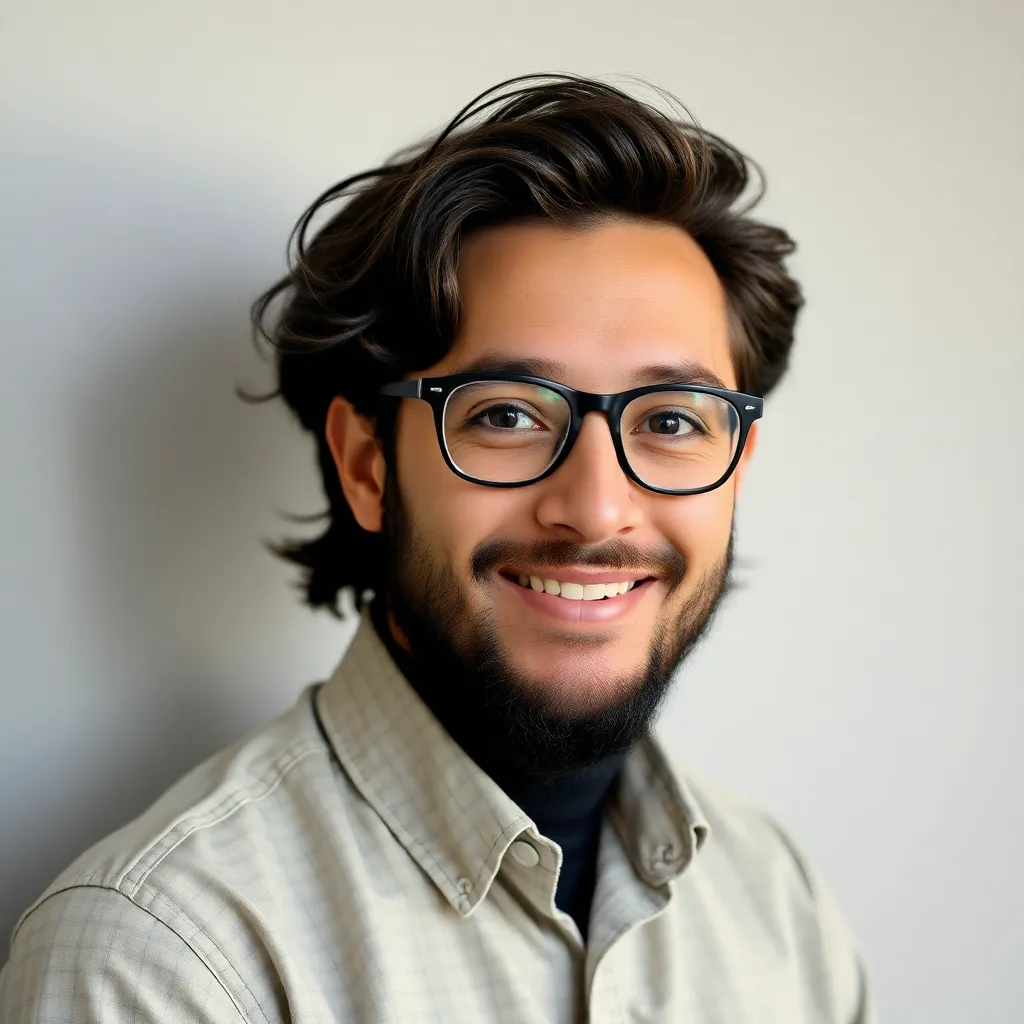
Breaking News Today
Apr 10, 2025 · 6 min read

Table of Contents
The Standard Deviation: Used in Conjunction With the Mean for Powerful Data Analysis
The standard deviation, a cornerstone of descriptive statistics, isn't a standalone metric. Its true power emerges when used in conjunction with the mean, providing a comprehensive understanding of data distribution and variability. This article delves deep into the synergistic relationship between these two statistical measures, exploring their applications across various fields and highlighting their importance in data interpretation and decision-making.
Understanding the Mean and Standard Deviation
Before exploring their interplay, let's briefly revisit each measure individually:
The Mean: A Measure of Central Tendency
The mean, or average, represents the central point of a dataset. It's calculated by summing all data points and dividing by the total number of data points. While simple to compute, the mean alone can be misleading, especially when dealing with datasets containing outliers or skewed distributions. It provides a single point of summary but lacks information about the data's spread or dispersion.
The Standard Deviation: A Measure of Data Dispersion
The standard deviation quantifies the amount of variation or dispersion within a dataset. It measures how much individual data points deviate from the mean. A small standard deviation indicates that data points cluster closely around the mean, signifying low variability. Conversely, a large standard deviation implies that data points are widely scattered, indicating high variability.
Calculating Standard Deviation: The standard deviation is calculated using the following steps:
- Calculate the mean (average) of the dataset.
- Find the difference between each data point and the mean.
- Square each of these differences.
- Sum the squared differences.
- Divide the sum of squared differences by (n-1), where 'n' is the number of data points (this is for sample standard deviation; for population standard deviation, divide by 'n').
- Take the square root of the result.
This process ensures that negative and positive deviations from the mean don't cancel each other out, providing a true representation of the overall dispersion.
The Synergistic Power of Mean and Standard Deviation
The true utility of the standard deviation becomes apparent when considered alongside the mean. Together, they paint a much more complete picture of the data than either measure can achieve independently.
1. Understanding Data Distribution: The mean tells us the central location, while the standard deviation reveals the shape of the distribution. A dataset with a small standard deviation will likely exhibit a narrow, bell-shaped (normal) distribution centered around the mean. In contrast, a large standard deviation suggests a broader, potentially skewed distribution, indicating the presence of outliers or non-normal distribution characteristics.
2. Identifying Outliers: Outliers are data points significantly deviating from the rest of the dataset. While the mean can be heavily influenced by outliers, the standard deviation helps to identify them. Using the standard deviation, we can establish a range within which most data points should fall. Points falling far outside this range can be flagged as potential outliers and subjected to further investigation. For example, using Z-scores (calculated by dividing the difference between a data point and the mean by the standard deviation), we can identify outliers that deviate significantly from the norm.
3. Comparing Datasets: When comparing two or more datasets, merely comparing the means can be deceptive. The standard deviations provide crucial context. Two datasets might have similar means but drastically different standard deviations, indicating vastly different levels of variability. This information is critical in various comparisons, from comparing the performance of different investment strategies to analyzing the effectiveness of different medical treatments.
4. Confidence Intervals and Hypothesis Testing: In statistical inference, the mean and standard deviation are fundamental for constructing confidence intervals and performing hypothesis tests. Confidence intervals provide a range of values within which the true population mean is likely to fall, with a certain level of confidence (e.g., 95%). The standard deviation is crucial in determining the width of these intervals; a smaller standard deviation leads to narrower intervals, indicating greater precision in estimating the population mean. Hypothesis tests, similarly, rely on the standard deviation to assess the significance of observed differences between groups or samples. Standard error, which is the standard deviation of the sampling distribution of the mean, depends directly on the standard deviation of the original data.
5. Data Normalization and Standardization: Many statistical models and machine learning algorithms require data to be normally distributed. The standard deviation plays a crucial role in transforming non-normally distributed data into a more normal distribution. Standardization (or Z-score normalization) involves subtracting the mean from each data point and then dividing by the standard deviation. This process centers the data around zero and scales it by the standard deviation, making it suitable for various statistical techniques.
Applications Across Disciplines
The combined power of the mean and standard deviation is evident across diverse fields:
- Finance: Analyzing investment returns, assessing risk levels, and constructing portfolios. Standard deviation is often used as a measure of investment risk—higher standard deviation implies higher risk.
- Healthcare: Monitoring patient vital signs, analyzing clinical trial data, and evaluating treatment effectiveness. Analyzing the distribution of blood pressure or heart rate, for example, requires both the mean and standard deviation for a complete picture.
- Manufacturing: Monitoring product quality, controlling process variability, and ensuring consistency. Standard deviation is crucial in quality control, helping to identify variations in product dimensions or other characteristics.
- Engineering: Analyzing experimental results, evaluating the reliability of systems, and ensuring safety standards are met. In evaluating the strength of materials or the performance of machines, the standard deviation helps to quantify the variability and ensure that the average performance is representative of the overall behavior.
- Environmental Science: Analyzing pollutant levels, monitoring climate change impacts, and modeling ecological systems. The mean and standard deviation are integral to understanding environmental data and variability.
Beyond the Basics: Advanced Concepts
The interplay between the mean and standard deviation extends beyond the fundamental concepts discussed above. Several advanced statistical concepts rely heavily on these measures:
- Coefficient of Variation: This dimensionless measure compares the standard deviation relative to the mean, providing a standardized measure of variability that's useful when comparing datasets with vastly different means.
- Skewness and Kurtosis: These measures describe the asymmetry and peakedness of a distribution, respectively. They are often used in conjunction with the mean and standard deviation to provide a more comprehensive description of the data's distribution.
- Chebyshev's Inequality: This inequality provides a lower bound on the proportion of data within a certain number of standard deviations from the mean, regardless of the distribution's shape. This is particularly useful when dealing with datasets that are not normally distributed.
Conclusion: A Powerful Duo
In conclusion, the standard deviation is not just a number; it's a crucial measure that, when used in conjunction with the mean, provides a powerful tool for understanding and interpreting data. Their synergistic relationship unlocks insights into data distribution, variability, and outliers, enabling effective data analysis across various fields. From simple descriptive statistics to complex statistical modeling, the mean and standard deviation remain indispensable tools for data scientists, researchers, and anyone involved in data-driven decision-making. Mastering their interplay is essential for unlocking the full potential of your data and drawing meaningful conclusions. Understanding their limitations, and considering other descriptive statistics and data visualization techniques, complements the information provided by these two key measures, leading to more robust and insightful analyses.
Latest Posts
Latest Posts
-
When Answering A Multiple Choice Question Milady
Apr 18, 2025
-
What Reasons For Healthcare Encounters Are Included In Chapter 21
Apr 18, 2025
-
Common Type A Soils Are Each Of The Following Except
Apr 18, 2025
-
Jodi Is Traveling To San Francisco For A Conference
Apr 18, 2025
-
A High Premium On Material Possessions And Money
Apr 18, 2025
Related Post
Thank you for visiting our website which covers about The Standard Deviation Is Used In Conjunction With The . We hope the information provided has been useful to you. Feel free to contact us if you have any questions or need further assistance. See you next time and don't miss to bookmark.