The Study Of Statistics Rests On What Two Major Concepts
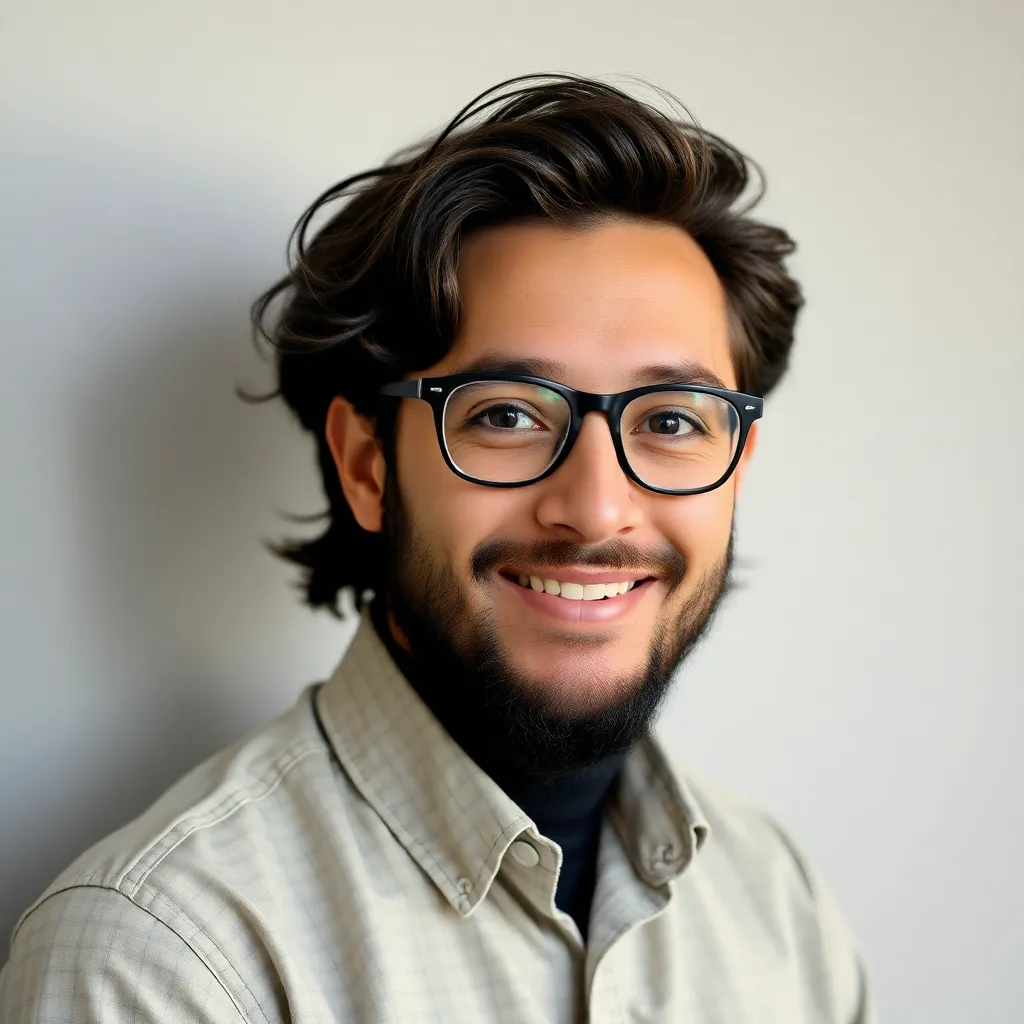
Breaking News Today
Apr 03, 2025 · 6 min read

Table of Contents
- The Study Of Statistics Rests On What Two Major Concepts
- Table of Contents
- The Study of Statistics Rests on What Two Major Concepts?
- Probability: The Foundation of Chance
- Key Concepts within Probability:
- Probability in Action: Real-World Examples
- Statistical Inference: Drawing Conclusions from Data
- Core Components of Statistical Inference:
- Statistical Inference in Practice: Applications Across Disciplines
- The Interplay Between Probability and Inference
- Avoiding Common Misconceptions
- Conclusion: Mastering the Tools of Data Analysis
- Latest Posts
- Latest Posts
- Related Post
The Study of Statistics Rests on What Two Major Concepts?
The field of statistics, crucial for making sense of the world around us, fundamentally rests on two major concepts: probability and inference. These concepts aren't merely theoretical underpinnings; they're the practical tools that allow us to analyze data, draw conclusions, and make informed decisions in countless areas, from medicine and finance to social sciences and environmental studies. Understanding these two concepts is key to understanding statistics itself. Let's delve deeper into each.
Probability: The Foundation of Chance
Probability is the cornerstone of statistics. It deals with the likelihood of events occurring. While deterministic systems predict outcomes with certainty (like calculating the area of a rectangle), probabilistic systems deal with uncertainty. This uncertainty is inherent in many real-world phenomena. We can't predict with absolute certainty whether a specific coin flip will result in heads or tails, or if a particular patient will respond positively to a new drug. Probability provides a framework for quantifying this uncertainty.
Key Concepts within Probability:
-
Random Variables: These are variables whose values are numerical outcomes of random phenomena. For instance, the number of heads observed in ten coin flips is a random variable. Random variables can be discrete (taking on specific values, like the number of heads) or continuous (taking on any value within a range, like the height of a person).
-
Probability Distributions: These describe the probability of different outcomes for a random variable. They can be visualized through graphs or tables. Common probability distributions include the normal distribution (bell curve), binomial distribution (for binary outcomes), and Poisson distribution (for rare events). Understanding probability distributions is essential for statistical inference.
-
Expected Value and Variance: These are crucial summary statistics for probability distributions. The expected value represents the average outcome we expect to observe over many repetitions of the random phenomenon. The variance measures the spread or dispersion of the outcomes around the expected value. A higher variance indicates greater uncertainty.
-
Conditional Probability and Bayes' Theorem: Conditional probability addresses the probability of an event occurring given that another event has already occurred. Bayes' Theorem is a powerful tool for updating probabilities based on new evidence, which has applications in various fields, such as medical diagnosis and spam filtering.
Probability in Action: Real-World Examples
-
Medical Trials: Probability is used to assess the effectiveness of new drugs. Researchers calculate the probability of a positive outcome (e.g., recovery) in the treatment group compared to a control group.
-
Weather Forecasting: Weather forecasts rely heavily on probability. Meteorologists use statistical models to predict the likelihood of rain, snow, or other weather events.
-
Insurance: Insurance companies use probability to assess the risk of various events, such as car accidents or house fires, and set premiums accordingly.
Statistical Inference: Drawing Conclusions from Data
Statistical inference takes the concepts of probability and applies them to draw conclusions about a population based on a sample drawn from that population. It bridges the gap between the data we observe and the broader conclusions we want to make. This is crucial because studying an entire population is often impractical or impossible.
Core Components of Statistical Inference:
-
Sampling: The process of selecting a representative subset from a larger population. The method of sampling significantly impacts the reliability of inferences. Random sampling is crucial to minimize bias.
-
Estimators: These are statistical measures used to estimate population parameters (e.g., population mean, population standard deviation). Good estimators are unbiased (their expected value equals the population parameter) and efficient (they have a low variance).
-
Hypothesis Testing: This is a formal procedure used to test specific claims or hypotheses about a population. It involves setting up null and alternative hypotheses, collecting data, calculating a test statistic, and determining whether the evidence supports rejecting the null hypothesis. Common tests include t-tests, z-tests, and chi-squared tests.
-
Confidence Intervals: These provide a range of values within which a population parameter is likely to fall with a certain level of confidence. For instance, a 95% confidence interval suggests that there's a 95% probability that the true population parameter lies within that range.
-
Regression Analysis: This is a powerful technique used to model the relationship between variables. It allows us to predict the value of a dependent variable based on the values of independent variables. Linear regression is a common type of regression analysis.
Statistical Inference in Practice: Applications Across Disciplines
-
Market Research: Companies use statistical inference to analyze survey data and understand consumer preferences.
-
Public Health: Epidemiologists use statistical methods to study disease outbreaks and identify risk factors.
-
Economics: Economists use statistical models to forecast economic growth and analyze the impact of policy changes.
-
Environmental Science: Scientists use statistical techniques to analyze environmental data and assess the impact of pollution or climate change.
-
Social Sciences: Sociologists and psychologists use statistical methods to analyze survey data and experimental results to understand social phenomena and human behavior.
The Interplay Between Probability and Inference
Probability and inference are deeply intertwined. Probability provides the theoretical foundation for inference. The validity of statistical inferences depends on the underlying probability models. For example, the reliability of a confidence interval hinges on the assumptions made about the probability distribution of the data. When we conduct a hypothesis test, we use probability to determine the likelihood of observing the data if the null hypothesis were true. A low probability suggests that the null hypothesis is unlikely, leading to its rejection.
Avoiding Common Misconceptions
Several common misconceptions surround probability and statistical inference. It's crucial to avoid these pitfalls for accurate and reliable analyses:
-
Correlation does not equal causation: Just because two variables are correlated doesn't mean one causes the other. There could be a third, confounding variable or the relationship could be purely coincidental.
-
Sample bias: Inferences are only as good as the data they are based on. Biased samples lead to inaccurate conclusions. It's vital to ensure representative samples to avoid misleading results.
-
Overfitting: In complex models (like regression models), overfitting occurs when the model fits the sample data too closely but doesn't generalize well to new data. This leads to poor predictions.
-
Misinterpreting p-values: A low p-value doesn't automatically mean the null hypothesis is false. It indicates the likelihood of observing the data if the null hypothesis were true, not the probability that the null hypothesis is true.
-
Ignoring confidence intervals: While hypothesis tests provide a yes/no answer, confidence intervals provide a more nuanced understanding of the uncertainty surrounding estimates. They give a range of plausible values for the population parameter, providing a more complete picture.
Conclusion: Mastering the Tools of Data Analysis
The study of statistics hinges on the fundamental concepts of probability and inference. Understanding these concepts allows us to extract meaningful insights from data, make informed decisions, and address complex real-world problems across diverse fields. While the mathematical underpinnings can be challenging, the ability to analyze data and draw valid conclusions is increasingly vital in today's data-driven world. Mastering these core statistical concepts empowers individuals and organizations to navigate uncertainty, make better decisions, and contribute to advancements across many disciplines. The continuous development and refinement of statistical methods ensure the ongoing relevance and impact of these fundamental concepts in understanding and shaping our world.
Latest Posts
Latest Posts
-
Correctly Label The Following Tissues Of The Digestive Tract
Apr 08, 2025
-
Which Of The Following Is Not Correct Concerning Nerves
Apr 08, 2025
-
Phishing Is Responsible For Most Of The Recent Pii Breaches
Apr 08, 2025
-
Assigning Your Team Members To Individual Roles
Apr 08, 2025
-
When Cleaning Up Blood Use Cloth Towels
Apr 08, 2025
Related Post
Thank you for visiting our website which covers about The Study Of Statistics Rests On What Two Major Concepts . We hope the information provided has been useful to you. Feel free to contact us if you have any questions or need further assistance. See you next time and don't miss to bookmark.