The Two Main Branches Of Statistics Are
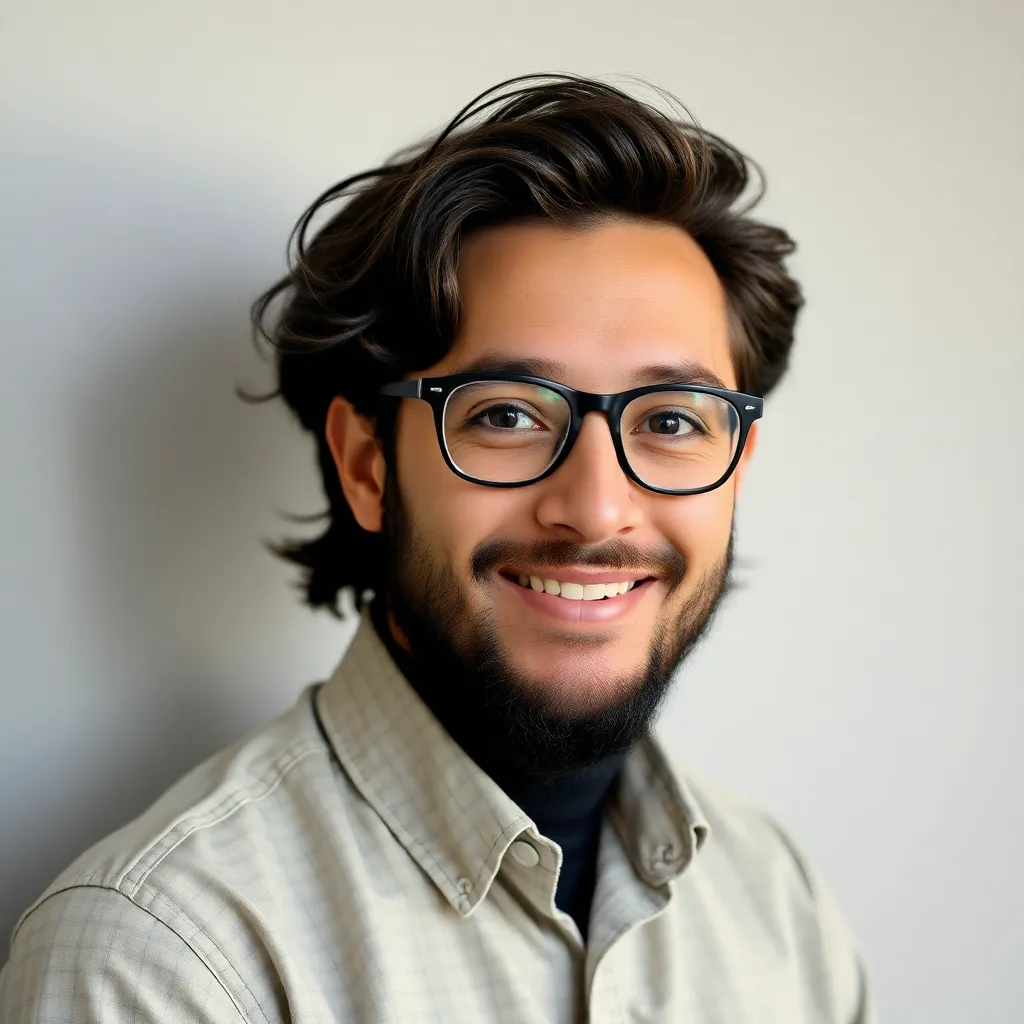
Breaking News Today
Apr 26, 2025 · 6 min read

Table of Contents
The Two Main Branches of Statistics: Descriptive and Inferential
Statistics, the science of collecting, analyzing, interpreting, presenting, and organizing data, is a cornerstone of modern research and decision-making across numerous fields. From understanding market trends to predicting election outcomes, from designing effective medical treatments to optimizing manufacturing processes, statistics provides the crucial tools for making sense of the world around us. While the field is vast and multifaceted, it's fundamentally divided into two major branches: descriptive statistics and inferential statistics. Understanding the differences and applications of each is essential for anyone working with data.
Descriptive Statistics: Summarizing and Presenting Data
Descriptive statistics, as its name suggests, focuses on describing and summarizing data. It doesn't attempt to make generalizations beyond the data at hand; its primary goal is to present a clear and concise picture of the collected information. This is achieved through a variety of methods, including:
Measures of Central Tendency
These statistics provide a single value that represents the "center" or "typical" value of a dataset. The most common measures are:
- Mean: The average of all values in a dataset. Calculated by summing all values and dividing by the total number of values. Highly sensitive to outliers (extreme values).
- Median: The middle value when a dataset is ordered from least to greatest. Less sensitive to outliers than the mean.
- Mode: The value that appears most frequently in a dataset. Can be used for both numerical and categorical data.
Measures of Dispersion (or Variability)
These statistics describe how spread out or scattered the data points are. Key measures include:
- Range: The difference between the highest and lowest values in a dataset. A simple measure but highly sensitive to outliers.
- Variance: The average of the squared differences from the mean. Provides a measure of the average spread of the data.
- Standard Deviation: The square root of the variance. Expressed in the same units as the original data, making it easier to interpret than variance. A larger standard deviation indicates greater variability.
- Interquartile Range (IQR): The difference between the third quartile (75th percentile) and the first quartile (25th percentile). A robust measure of dispersion, less sensitive to outliers than the range.
Data Visualization
Descriptive statistics are often accompanied by visual representations of the data to make it more easily understandable. Common techniques include:
- Histograms: Show the frequency distribution of a continuous variable.
- Bar Charts: Show the frequency distribution of a categorical variable.
- Pie Charts: Show the proportion of each category in a categorical variable.
- Box Plots: Display the median, quartiles, and outliers of a dataset. Useful for comparing the distribution of multiple datasets.
- Scatter Plots: Show the relationship between two continuous variables.
Example: Imagine a researcher collecting data on the heights of 100 students. Descriptive statistics would involve calculating the mean, median, and mode height; calculating the standard deviation to understand the variability in heights; and creating a histogram to visualize the distribution of heights. This summarizes the data without making any inferences about the heights of students beyond those in the sample.
Inferential Statistics: Making Generalizations and Predictions
Inferential statistics takes descriptive statistics a step further. Instead of simply summarizing the data at hand, it uses sample data to make inferences about a larger population. It involves making estimations, predictions, and testing hypotheses about the population based on the information gathered from a representative sample. This is crucial because it's often impractical or impossible to collect data from an entire population.
Key Concepts in Inferential Statistics
- Population: The entire group of individuals or objects that are of interest in a study.
- Sample: A subset of the population selected for study. The sample should be representative of the population to ensure the inferences made are accurate.
- Sampling Techniques: Methods used to select a sample from the population. Various techniques exist, each with its own strengths and weaknesses. Proper sampling is crucial for the validity of inferential statistics.
- Probability Distributions: Mathematical functions that describe the likelihood of different outcomes. Understanding probability distributions is fundamental to inferential statistics. Examples include the normal distribution, binomial distribution, and t-distribution.
- Hypothesis Testing: A formal procedure used to test claims about a population based on sample data. Involves stating a null hypothesis (no effect) and an alternative hypothesis (an effect exists) and then using statistical tests to determine whether to reject or fail to reject the null hypothesis. The p-value, a measure of the strength of evidence against the null hypothesis, plays a crucial role.
- Confidence Intervals: Ranges of values that are likely to contain the true population parameter with a certain degree of confidence. For example, a 95% confidence interval for the mean height of students means that there's a 95% probability that the true mean height falls within that interval.
- Regression Analysis: A statistical technique used to model the relationship between a dependent variable and one or more independent variables. Used for prediction and understanding the influence of independent variables on the dependent variable.
Common Inferential Statistical Tests
The choice of statistical test depends on the type of data (categorical or numerical) and the research question. Some common examples include:
- t-tests: Used to compare the means of two groups.
- ANOVA (Analysis of Variance): Used to compare the means of three or more groups.
- Chi-square test: Used to analyze categorical data and determine if there's an association between two categorical variables.
- Correlation analysis: Used to measure the strength and direction of the linear relationship between two continuous variables.
Example: A pharmaceutical company wants to test the effectiveness of a new drug. They conduct a clinical trial with a sample of patients, measuring their blood pressure before and after taking the drug. Inferential statistics would be used to analyze the data and determine whether the observed decrease in blood pressure in the sample is statistically significant enough to conclude that the drug is effective for the entire population of patients with the condition. This might involve using a paired t-test to compare the before-and-after blood pressure measurements and calculating a confidence interval for the mean difference in blood pressure.
The Interrelationship Between Descriptive and Inferential Statistics
While distinct, descriptive and inferential statistics are inherently linked. Inferential statistics relies heavily on descriptive statistics to summarize the sample data before making inferences about the population. The mean, standard deviation, and other descriptive statistics of the sample are used as inputs for inferential statistical tests and calculations. For instance, the mean of a sample is used to estimate the population mean, and the sample standard deviation is used to estimate the population standard deviation.
Conclusion: Choosing the Right Statistical Approach
The choice between descriptive and inferential statistics depends entirely on the research goals. If the goal is simply to summarize and present the characteristics of a dataset, descriptive statistics is sufficient. However, if the goal is to make generalizations about a population based on a sample, inferential statistics is necessary. In many research projects, both types of statistics are used in conjunction, with descriptive statistics providing the foundation for inferential analysis. Understanding the strengths and limitations of each branch is crucial for conducting sound statistical analysis and drawing meaningful conclusions from data. This fundamental understanding allows researchers to effectively communicate their findings and contribute to knowledge advancement in their respective fields. Mastering both descriptive and inferential statistics equips researchers and data analysts with a powerful toolkit for navigating the complexities of data analysis and contributing significantly to evidence-based decision-making in a wide range of disciplines.
Latest Posts
Latest Posts
-
A Blank Sign Must Have Red As The Dominant Color
Apr 26, 2025
-
Which Of The Following Is True Regarding Equestrian Portraits
Apr 26, 2025
-
All Sonnets Have Fourteen Lines True False
Apr 26, 2025
-
Which Statement Regarding Depression And Gender Is Correct
Apr 26, 2025
-
Why Is It Important To Ease Into An Exercise Program
Apr 26, 2025
Related Post
Thank you for visiting our website which covers about The Two Main Branches Of Statistics Are . We hope the information provided has been useful to you. Feel free to contact us if you have any questions or need further assistance. See you next time and don't miss to bookmark.