Trend Analysis And Time-series Analysis Refer To Analysis
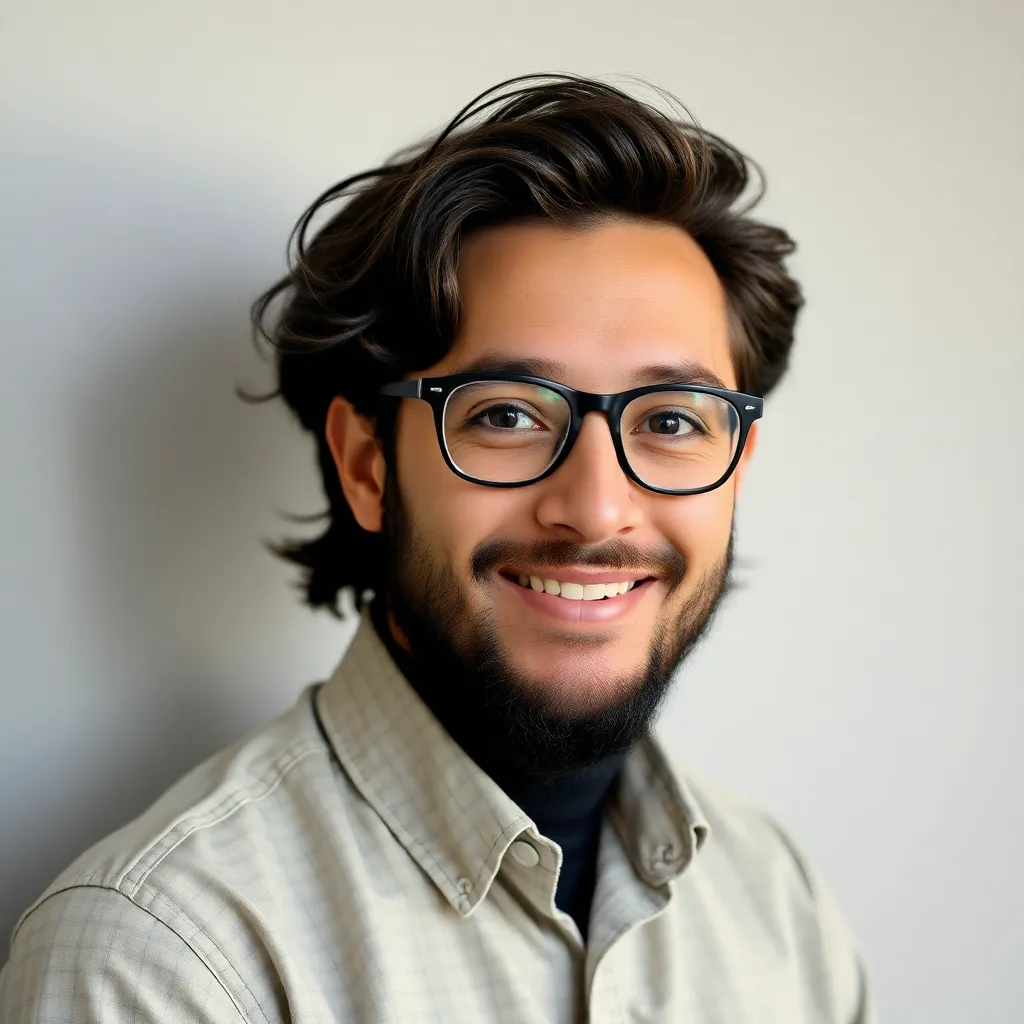
Breaking News Today
Apr 17, 2025 · 6 min read

Table of Contents
Trend Analysis and Time Series Analysis: A Comprehensive Guide
Trend analysis and time series analysis are powerful statistical tools used to understand patterns and make predictions based on data collected over time. While often used interchangeably, they have distinct focuses and methodologies. This comprehensive guide will delve into both, exploring their definitions, applications, methodologies, and limitations.
What is Trend Analysis?
Trend analysis focuses on identifying long-term patterns and directions in data. It's less concerned with the short-term fluctuations and more interested in the overall trajectory of a variable over an extended period. Think of it as looking at the "big picture" of your data. This involves identifying whether a trend is upward, downward, or stagnant. This information is then used to make informed decisions about future strategies and planning.
Key Characteristics of Trend Analysis:
- Focus on Long-Term Patterns: Trend analysis prioritizes the overall direction of the data, smoothing out short-term noise.
- Qualitative and Quantitative Methods: It utilizes both qualitative observations (e.g., market insights) and quantitative data analysis (e.g., regression analysis) to identify trends.
- Visual Representation: Graphical representations like line charts are crucial for visualizing and interpreting trends.
- Predictive Power: While not as precise as time series forecasting, it provides valuable insights into the likely future direction of a variable.
Methods Used in Trend Analysis:
- Moving Averages: This technique smooths out short-term fluctuations by calculating the average of data points over a specified period. A longer moving average period will result in a smoother line, better highlighting the underlying trend.
- Regression Analysis: This statistical method fits a line or curve to the data, allowing for the quantification of the trend's slope (rate of change) and prediction of future values. Linear regression is suitable for linear trends, while polynomial regression can model more complex curves.
- Exponential Smoothing: This method assigns exponentially decreasing weights to older data points, giving more importance to recent observations. This is particularly useful when dealing with data exhibiting seasonality or cyclical patterns.
- Qualitative Techniques: Expert opinions, market research, and Delphi studies can supplement quantitative methods, providing valuable context and insights into potential future trends.
Applications of Trend Analysis:
Trend analysis finds applications in numerous fields, including:
- Business: Identifying market trends, forecasting sales, analyzing customer behavior, and making strategic decisions.
- Finance: Predicting stock prices, assessing investment risk, and managing portfolios.
- Healthcare: Tracking disease outbreaks, analyzing patient demographics, and evaluating healthcare program effectiveness.
- Environmental Science: Monitoring climate change, assessing pollution levels, and studying ecosystem changes.
- Social Sciences: Analyzing demographic trends, tracking social movements, and studying societal changes.
What is Time Series Analysis?
Time series analysis is a more rigorous and sophisticated statistical technique focusing on understanding the temporal dependencies within a dataset. It delves deeper than trend analysis, analyzing the short-term and long-term patterns, seasonality, and cyclical variations within the data. It's used to model and forecast the future values of a variable based on its historical behavior.
Key Characteristics of Time Series Analysis:
- Temporal Dependence: The core principle is recognizing that data points are correlated over time. Past values influence future values.
- Decomposition: Time series data is often decomposed into its constituent components: trend, seasonality, cyclicity, and residuals (random noise).
- Forecasting: The primary goal is to predict future values based on the identified patterns and models.
- Statistical Modeling: Various statistical models are employed, depending on the characteristics of the data.
Methods Used in Time Series Analysis:
- ARIMA Models (Autoregressive Integrated Moving Average): These are powerful models that capture the autocorrelations within the time series. They are particularly effective for stationary data (data with constant mean and variance). Different variations (e.g., ARIMA(p,d,q)) exist depending on the parameters (p, d, q) that represent autoregressive, differencing, and moving average components respectively.
- SARIMA Models (Seasonal ARIMA): These extend ARIMA models to account for seasonality in the data. They are suitable for time series exhibiting regular seasonal patterns.
- Exponential Smoothing Methods (Holt-Winters): These methods, particularly the Holt-Winters method, are effective for data with trends and seasonality. They offer a simpler alternative to ARIMA models, especially for forecasting shorter-term future values.
- Prophet (from Meta): This is a relatively recent model specifically designed for business time series data with strong seasonality and trend. It is known for its robustness and ability to handle missing data.
- GARCH Models (Generalized Autoregressive Conditional Heteroskedasticity): These models are used to model the volatility (variance) of the time series, particularly useful in finance for managing risk.
Applications of Time Series Analysis:
The applications of time series analysis are widespread and include:
- Finance: Forecasting stock prices, analyzing market volatility, and managing investment risk.
- Economics: Predicting economic growth, inflation, and unemployment rates.
- Supply Chain Management: Forecasting demand, optimizing inventory levels, and improving logistics.
- Energy Forecasting: Predicting electricity demand, optimizing energy production, and improving grid management.
- Environmental Science: Forecasting weather patterns, analyzing pollution levels, and managing water resources.
Comparing Trend Analysis and Time Series Analysis:
While both deal with data over time, they differ significantly in their approach and goals:
Feature | Trend Analysis | Time Series Analysis |
---|---|---|
Focus | Long-term patterns and direction | Short-term and long-term patterns, seasonality |
Methodology | Simpler methods, often visual | Sophisticated statistical modeling |
Goal | Identify overall trend, qualitative insights | Precise forecasting, understanding temporal dependencies |
Data Handling | Often aggregates data, less detail needed | Requires detailed data with accurate timestamps |
Predictive Power | Less precise, broader predictions | More precise, detailed forecasts |
Limitations of Trend and Time Series Analysis:
Both methods have limitations:
- Data Dependency: Both rely heavily on the quality and quantity of historical data. Insufficient or inaccurate data can lead to misleading results.
- Assumptions: Statistical models often make assumptions (e.g., stationarity) that may not always hold true in real-world data.
- External Factors: Both struggle to incorporate unforeseen events or external factors that can significantly impact the variable being analyzed. A sudden economic downturn, a natural disaster, or a major policy shift can invalidate forecasts.
- Overfitting: Complex models, like some ARIMA variants, can overfit the data, performing well on historical data but poorly on future data. Model selection and validation are crucial to prevent overfitting.
- Interpretation: Correct interpretation of the results is crucial. Misinterpreting statistical significance or failing to consider context can lead to flawed conclusions.
Conclusion:
Trend analysis and time series analysis are valuable tools for understanding patterns and making predictions from time-dependent data. Trend analysis provides a broader overview of long-term directional changes, while time series analysis offers more precise forecasting through detailed modeling of temporal dependencies. Choosing the appropriate method depends on the specific research question, the characteristics of the data, and the desired level of precision in the analysis and forecasts. Understanding their strengths and limitations is essential for accurate interpretation and effective decision-making. Remember to always validate your models and consider the potential impact of unforeseen events when making predictions based on these analyses.
Latest Posts
Latest Posts
-
Glands That Respond To Rising Androgen Levels Are The
Apr 19, 2025
-
Which Of The Following Personally Owned Peripherals 2024
Apr 19, 2025
-
Unit 2 Progress Check Frq Ap Bio
Apr 19, 2025
-
Flour Milled To Leave Some Of The Bran Intact
Apr 19, 2025
-
La 1 Of 1 De Sara Es Grande
Apr 19, 2025
Related Post
Thank you for visiting our website which covers about Trend Analysis And Time-series Analysis Refer To Analysis . We hope the information provided has been useful to you. Feel free to contact us if you have any questions or need further assistance. See you next time and don't miss to bookmark.