Unit 11 Probability And Statistics Answer Key
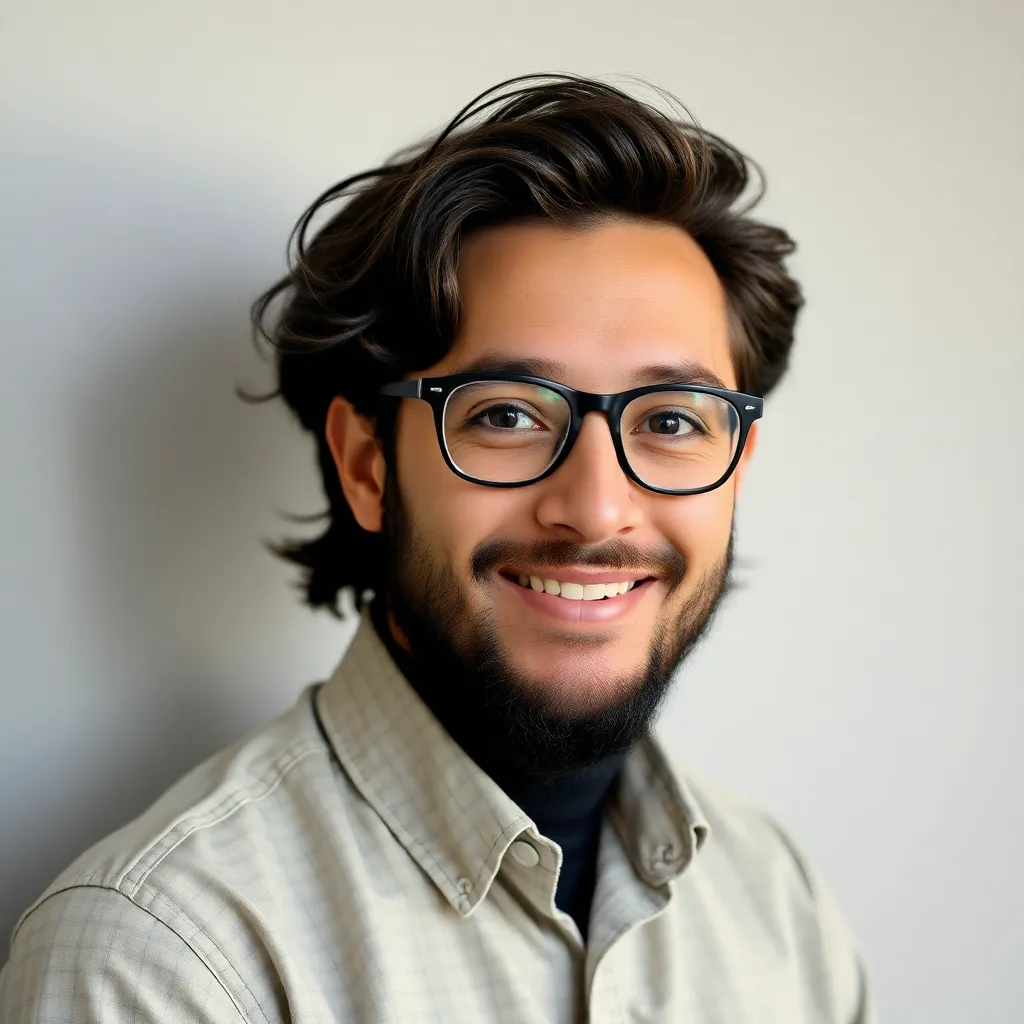
Breaking News Today
Apr 13, 2025 · 7 min read

Table of Contents
Unit 11: Probability and Statistics - A Comprehensive Guide and Answer Key
This comprehensive guide delves into the core concepts of Unit 11, Probability and Statistics, providing a detailed explanation of key topics and offering solutions to common problems. We'll cover probability distributions, statistical inference, hypothesis testing, and more, ensuring you develop a strong understanding of this crucial area of mathematics. This guide serves as a valuable resource whether you're a student aiming for exam success or an individual seeking to enhance your statistical literacy.
Understanding Probability: The Foundation of Statistics
Before we delve into the intricacies of statistical analysis, it’s crucial to grasp the fundamentals of probability. Probability quantifies the likelihood of an event occurring. It's expressed as a number between 0 and 1, where 0 represents an impossible event and 1 represents a certain event.
Types of Probability
Several approaches exist for determining probability:
-
Theoretical Probability: This is calculated based on logical reasoning and the possible outcomes of an event. For instance, the theoretical probability of flipping a fair coin and getting heads is 1/2.
-
Experimental Probability: This is determined by conducting experiments and observing the outcomes. The more trials conducted, the closer the experimental probability approaches the theoretical probability. For example, flipping a coin 100 times and observing the frequency of heads provides an experimental probability.
-
Subjective Probability: This relies on personal judgment and beliefs, rather than calculations or experiments. It's often used in situations with limited data or when dealing with uncertain events.
Key Probability Concepts
-
Independent Events: The occurrence of one event doesn't affect the probability of another. For example, rolling a die twice are independent events.
-
Dependent Events: The occurrence of one event impacts the probability of another. For example, drawing two cards from a deck without replacement.
-
Mutually Exclusive Events: Two events cannot occur simultaneously. For example, flipping a coin – you cannot get both heads and tails at the same time.
-
Conditional Probability: The probability of an event occurring given that another event has already occurred. This is denoted as P(A|B), the probability of A given B.
Probability Distributions: Modeling Random Variables
A random variable is a variable whose value is a numerical outcome of a random phenomenon. Probability distributions describe the likelihood of different outcomes for a random variable.
Common Probability Distributions
-
Binomial Distribution: Models the probability of a specific number of successes in a fixed number of independent Bernoulli trials (trials with only two possible outcomes, success or failure). Key parameters are 'n' (number of trials) and 'p' (probability of success).
-
Poisson Distribution: Models the probability of a given number of events occurring in a fixed interval of time or space, given the average rate of occurrence. The key parameter is λ (lambda), representing the average rate.
-
Normal Distribution: A continuous probability distribution characterized by its bell shape. It's defined by its mean (μ) and standard deviation (σ). Many natural phenomena approximately follow a normal distribution.
-
Uniform Distribution: A probability distribution where all outcomes are equally likely. For example, rolling a fair six-sided die follows a uniform distribution.
Statistical Inference: Drawing Conclusions from Data
Statistical inference involves using sample data to make inferences about a population. This typically involves estimating population parameters or testing hypotheses about them.
Estimation
-
Point Estimation: Provides a single value as an estimate for a population parameter (e.g., the sample mean as an estimate of the population mean).
-
Interval Estimation: Provides a range of values within which the population parameter is likely to fall (e.g., confidence intervals). Confidence intervals are constructed based on the chosen confidence level (e.g., 95%).
Hypothesis Testing
Hypothesis testing involves formulating a null hypothesis (a statement about a population parameter) and an alternative hypothesis (the opposite of the null hypothesis). We then use sample data to determine whether to reject the null hypothesis in favor of the alternative hypothesis. This involves calculating a test statistic and comparing it to a critical value or calculating a p-value.
Steps in Hypothesis Testing:
-
State the Hypotheses: Clearly define the null and alternative hypotheses.
-
Determine the Significance Level: Set the alpha level (α), typically 0.05, representing the probability of rejecting the null hypothesis when it is true (Type I error).
-
Calculate the Test Statistic: Use the sample data to calculate the appropriate test statistic (e.g., t-statistic, z-statistic, chi-square statistic).
-
Determine the P-value: The probability of obtaining a test statistic as extreme as or more extreme than the one calculated, assuming the null hypothesis is true.
-
Make a Decision: If the p-value is less than the significance level (α), reject the null hypothesis; otherwise, fail to reject the null hypothesis.
Common Statistical Tests
-
t-test: Used to compare the means of two groups. There are different versions of the t-test depending on the data (e.g., independent samples t-test, paired samples t-test).
-
z-test: Used to compare the means of two groups when the population standard deviation is known.
-
ANOVA (Analysis of Variance): Used to compare the means of three or more groups.
-
Chi-Square Test: Used to analyze categorical data and test for independence or goodness of fit.
Answer Key Examples (Illustrative)
Since a complete answer key for an entire Unit 11 on Probability and Statistics would be excessively long, I'll provide illustrative examples to demonstrate how to approach various problem types. These examples assume a basic understanding of the concepts previously explained. Remember to consult your textbook and class notes for specific problems and their solutions.
Example 1: Binomial Distribution
A coin is flipped 5 times. What is the probability of getting exactly 3 heads?
- Solution: This is a binomial distribution problem. n=5, p=0.5 (assuming a fair coin), and we want to find P(X=3). Using the binomial probability formula: P(X=k) = (nCk) * p^k * (1-p)^(n-k), we get: P(X=3) = (5C3) * (0.5)^3 * (0.5)^2 = 10 * 0.125 * 0.25 = 0.3125.
Example 2: Hypothesis Testing
A researcher wants to test if the average height of students is greater than 170 cm. A sample of 50 students has a mean height of 172 cm and a standard deviation of 5 cm. Conduct a one-sample t-test at a 0.05 significance level.
- Solution:
- Hypotheses: H0: μ ≤ 170 cm; H1: μ > 170 cm (one-tailed test).
- Significance level: α = 0.05.
- Test statistic: We would calculate the t-statistic using the formula: t = (sample mean - population mean) / (sample standard deviation / √sample size).
- P-value: We would then find the p-value associated with the calculated t-statistic using a t-distribution table or statistical software.
- Decision: If the p-value is less than 0.05, we reject the null hypothesis and conclude that the average height is significantly greater than 170 cm.
Example 3: Confidence Interval
Calculate a 95% confidence interval for the population mean based on the data from Example 2.
- Solution: We would use the formula for a confidence interval: Sample mean ± (t-critical value) * (sample standard deviation / √sample size). The t-critical value would be obtained from the t-distribution table based on the degrees of freedom (n-1) and the confidence level.
These examples illustrate the application of core concepts. A complete answer key would require addressing numerous problem types and variations within each topic, which is beyond the scope of this guide.
Improving Your Understanding of Unit 11
To strengthen your grasp of probability and statistics, consider the following strategies:
-
Practice Regularly: Work through numerous problems to build your problem-solving skills. Focus on understanding the underlying concepts, not just memorizing formulas.
-
Utilize Online Resources: Explore reputable websites and online courses that offer interactive exercises and explanations.
-
Seek Clarification: Don't hesitate to ask your teacher or instructor for help when you encounter difficulties.
By mastering the concepts covered in Unit 11, you'll develop a valuable skillset applicable to various fields, from data science and finance to healthcare and research. Remember that consistent effort and a focus on understanding the underlying principles are key to success in probability and statistics.
Latest Posts
Latest Posts
-
Fundamentals Of Logic Design 7th Edition Solutions Pdf
Apr 15, 2025
-
Unit 8 Progress Check Mcq Part B Apes
Apr 15, 2025
-
At Least Initially Remarriage Brings Which Conditions
Apr 15, 2025
-
What Do Fats Steroids And Waxes Have In Common
Apr 15, 2025
-
Drag Each Description To The Appropriate Bin
Apr 15, 2025
Related Post
Thank you for visiting our website which covers about Unit 11 Probability And Statistics Answer Key . We hope the information provided has been useful to you. Feel free to contact us if you have any questions or need further assistance. See you next time and don't miss to bookmark.