Unsupervised Learning In An Artificial Training System Improves
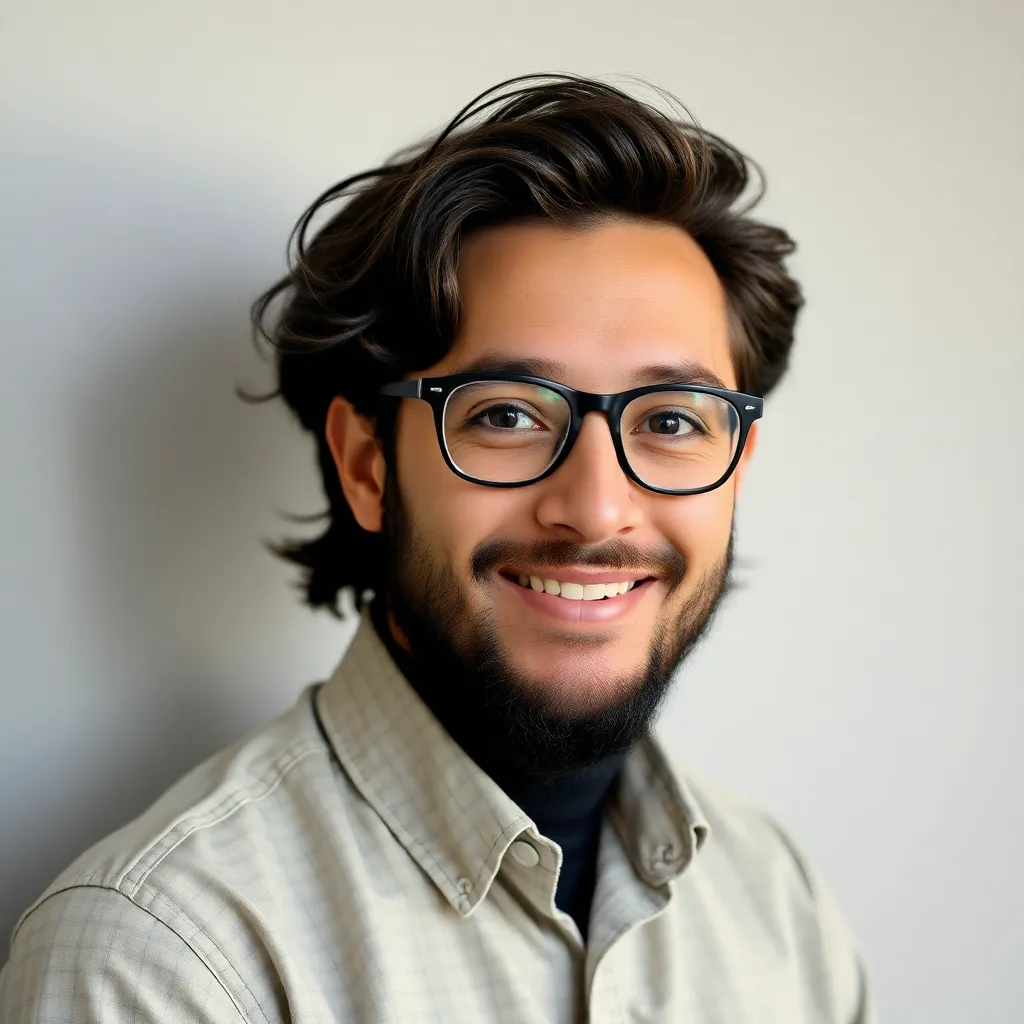
Breaking News Today
Apr 08, 2025 · 6 min read

Table of Contents
Unsupervised Learning in Artificial Training Systems: A Comprehensive Guide to Improved Performance
Unsupervised learning, a cornerstone of artificial intelligence (AI), is rapidly transforming how we train artificial systems. Unlike supervised learning, which relies on labeled data, unsupervised learning allows systems to discover patterns, structures, and anomalies in unlabeled datasets. This capability significantly improves the performance and adaptability of artificial training systems across various applications. This comprehensive guide delves into the intricacies of unsupervised learning, exploring its various techniques, benefits, challenges, and its transformative impact on the future of AI.
What is Unsupervised Learning?
Unsupervised learning is a machine learning technique where an algorithm learns from unlabeled data without any explicit guidance or feedback. The algorithm's goal is to identify inherent structures, relationships, and patterns within the data. This contrasts sharply with supervised learning, where the algorithm learns from labeled data, meaning each data point is associated with a known outcome or category. In essence, unsupervised learning is about letting the data speak for itself.
Key Characteristics of Unsupervised Learning:
- Unlabeled Data: The core characteristic is the absence of labeled data. The algorithm works with raw data without predefined categories or target variables.
- Pattern Discovery: The primary objective is to uncover hidden patterns, structures, and relationships within the data.
- Exploratory Data Analysis: Unsupervised learning serves as a powerful tool for exploratory data analysis, helping to understand data characteristics before applying other machine learning techniques.
- Anomaly Detection: It can identify outliers or anomalies that deviate significantly from the established patterns.
- Dimensionality Reduction: It can reduce the number of variables while retaining important information, simplifying the data for analysis and visualization.
Popular Unsupervised Learning Techniques:
Several powerful techniques drive unsupervised learning, each offering unique capabilities:
1. Clustering: Grouping Similar Data Points
Clustering algorithms group similar data points together based on their inherent characteristics. Popular clustering algorithms include:
- K-Means Clustering: Partitions data into k clusters, where each data point belongs to the cluster with the nearest mean (centroid). Choosing the optimal k is crucial and often involves techniques like the elbow method or silhouette analysis.
- Hierarchical Clustering: Builds a hierarchy of clusters, either agglomerative (bottom-up) or divisive (top-down). This creates a dendrogram, a tree-like structure visualizing the relationships between clusters.
- DBSCAN (Density-Based Spatial Clustering of Applications with Noise): Groups data points based on density, identifying clusters of varying shapes and sizes while handling outliers effectively.
2. Dimensionality Reduction: Simplifying Complex Data
Dimensionality reduction techniques decrease the number of variables while preserving essential information. This simplifies the data, making it easier to analyze and visualize, and reduces computational complexity. Common techniques include:
- Principal Component Analysis (PCA): Transforms data into a new coordinate system where principal components capture the maximum variance. This reduces dimensionality by retaining only the most significant components.
- t-distributed Stochastic Neighbor Embedding (t-SNE): A technique particularly useful for visualizing high-dimensional data in lower dimensions, preserving local neighborhood structures.
3. Association Rule Mining: Discovering Relationships Between Variables
Association rule mining discovers interesting relationships or associations between variables in large datasets. A prominent example is:
- Apriori Algorithm: Efficiently identifies frequent itemsets and generates association rules based on support and confidence measures. This is widely used in market basket analysis, predicting which products are frequently purchased together.
4. Anomaly Detection: Identifying Outliers
Anomaly detection techniques identify data points that deviate significantly from the norm. These outliers can represent errors, fraudulent activities, or other interesting phenomena. Examples include:
- One-Class SVM: Trains a model on "normal" data to identify deviations from this learned pattern.
- Isolation Forest: Isolates anomalies by randomly partitioning the data, with anomalies requiring fewer partitions to be isolated.
Benefits of Unsupervised Learning in Artificial Training Systems:
Unsupervised learning offers numerous benefits, significantly improving the performance and capabilities of artificial training systems:
- Data Exploration and Understanding: It allows for in-depth exploration of data without prior knowledge, revealing hidden patterns and insights.
- Improved Model Accuracy: By identifying underlying structures, it can enhance the accuracy of subsequent supervised learning models.
- Feature Engineering: Unsupervised learning techniques can create new, informative features from existing data, improving model performance.
- Anomaly Detection and Fraud Prevention: It enables the identification of unusual patterns that could indicate fraud, system failures, or other critical events.
- Reduced Data Dimensionality: Simplifies complex datasets, reducing computational costs and improving model efficiency.
- Personalized Recommendations: Clustering and association rule mining are crucial in developing recommendation systems that cater to individual preferences.
- Customer Segmentation: It helps businesses group customers based on shared characteristics, leading to targeted marketing strategies.
Challenges of Unsupervised Learning:
Despite its advantages, unsupervised learning faces challenges:
- Evaluation Difficulty: Assessing the performance of unsupervised learning algorithms is more challenging than supervised learning due to the lack of ground truth labels. Evaluation often relies on qualitative assessments or domain-specific metrics.
- Interpretability: Understanding the patterns and relationships discovered by unsupervised learning algorithms can be difficult, especially with complex datasets.
- Computational Complexity: Some unsupervised learning techniques, especially those dealing with large datasets, can be computationally expensive.
- Sensitivity to Parameter Tuning: The performance of many unsupervised learning algorithms is sensitive to the choice of parameters, requiring careful tuning and experimentation.
Improving Unsupervised Learning Performance:
Several strategies can enhance the performance of unsupervised learning algorithms:
- Data Preprocessing: Cleaning, transforming, and normalizing data are crucial steps to improve algorithm accuracy and efficiency. Handling missing values and outliers is particularly important.
- Feature Selection/Engineering: Carefully selecting or creating relevant features can significantly improve performance.
- Algorithm Selection: Choosing the appropriate algorithm based on the data characteristics and the specific task is critical.
- Parameter Tuning: Experimenting with different parameter settings to optimize algorithm performance is essential.
- Ensemble Methods: Combining multiple unsupervised learning algorithms can improve overall performance and robustness.
- Hybrid Approaches: Integrating unsupervised learning with supervised learning techniques can leverage the strengths of both approaches. For example, using clustering to preprocess data before applying a supervised classifier.
Unsupervised Learning in Specific Applications:
Unsupervised learning plays a vital role in diverse fields:
- Image Recognition: Clustering techniques group similar images based on visual features, improving image retrieval and object recognition.
- Natural Language Processing (NLP): Topic modeling helps uncover latent topics within large text corpora, useful for document summarization and sentiment analysis.
- Anomaly Detection in Cybersecurity: Identifies unusual network traffic patterns that might indicate cyberattacks.
- Recommendation Systems: Clustering and association rule mining personalize recommendations for users based on their preferences and past behavior.
- Medical Diagnosis: Identifies patterns in medical data to aid in the diagnosis of diseases.
- Financial Modeling: Detects fraudulent transactions and predicts market trends.
The Future of Unsupervised Learning:
Unsupervised learning is a rapidly evolving field with significant potential for future advancements. Research focuses on:
- Developing more robust and scalable algorithms: Addressing the computational complexity and scalability issues of current algorithms.
- Improving interpretability and explainability: Making it easier to understand the patterns and insights discovered by unsupervised learning algorithms.
- Hybrid approaches combining unsupervised and supervised learning: Leveraging the strengths of both paradigms to improve overall performance.
- Applications in new domains: Expanding the use of unsupervised learning in emerging areas like robotics, autonomous driving, and personalized medicine.
Conclusion:
Unsupervised learning is a powerful and versatile machine learning technique with far-reaching applications. While challenges remain, ongoing research and advancements are continuously improving its performance and expanding its capabilities. By understanding its principles, techniques, and limitations, we can harness its transformative potential to gain valuable insights from data, build more intelligent artificial training systems, and drive innovation across various industries. The future of AI is inextricably linked to the continued development and refinement of unsupervised learning methodologies, offering a path towards more autonomous, adaptable, and insightful artificial systems.
Latest Posts
Latest Posts
-
Match Each Business Structure With Its Description
Apr 17, 2025
-
The Horizontal Transfer Process Known As Transduction
Apr 17, 2025
-
Como Son Los Hermanos De Pilar Simpaticos
Apr 17, 2025
-
What Is The Primary Visible Color Of An Emission Nebula
Apr 17, 2025
-
The Three Match Types Vary In How Close
Apr 17, 2025
Related Post
Thank you for visiting our website which covers about Unsupervised Learning In An Artificial Training System Improves . We hope the information provided has been useful to you. Feel free to contact us if you have any questions or need further assistance. See you next time and don't miss to bookmark.