Using Models To Predict Molecular Structure Lab
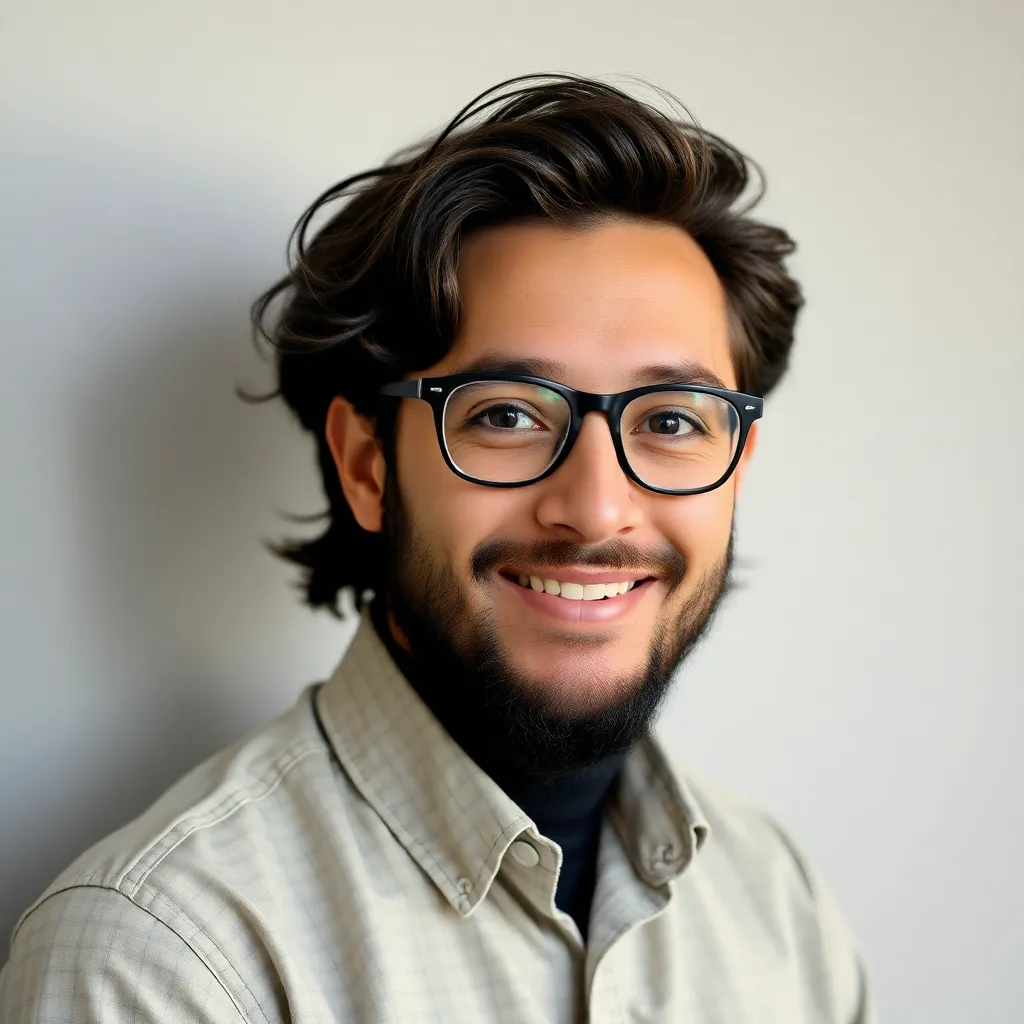
Breaking News Today
Apr 26, 2025 · 7 min read

Table of Contents
Using Models to Predict Molecular Structure: A Comprehensive Guide for Lab Experiments
Predicting molecular structure is crucial in various scientific disciplines, from materials science and drug discovery to environmental chemistry and biochemistry. While traditional experimental techniques like X-ray crystallography and NMR spectroscopy remain indispensable, computational modeling offers a powerful complementary approach, enabling faster, cheaper, and sometimes even more insightful predictions. This article will delve into the utilization of models to predict molecular structure in a laboratory setting, exploring different modeling techniques, their applications, limitations, and practical considerations.
Understanding the Role of Computational Modeling
Computational modeling serves as a virtual laboratory, allowing researchers to explore molecular structures and properties without the need for extensive, time-consuming, and often expensive experiments. These models leverage the principles of quantum mechanics, molecular mechanics, and statistical thermodynamics to simulate the behavior of molecules. By inputting known information about a molecule, such as its chemical formula or a partial structure, these models can predict its three-dimensional arrangement, including bond lengths, bond angles, and dihedral angles. This information is invaluable for understanding a molecule’s reactivity, stability, and interactions with other molecules.
The Power of Prediction: Advantages of Using Models
The advantages of integrating computational modeling into molecular structure prediction in the lab are numerous:
-
Speed and Efficiency: Modeling can provide preliminary structural insights much faster than traditional experimental techniques. This accelerates the research process, saving significant time and resources.
-
Cost-Effectiveness: Computational modeling is generally less expensive than many experimental methods, especially for complex molecules or systems.
-
Accessibility: Advanced modeling software and computational resources are becoming increasingly accessible, democratizing access to powerful predictive tools.
-
Complementary Nature: Models can complement experimental data, providing insights that are difficult or impossible to obtain experimentally. For example, modeling can predict the structure of transient intermediates or unstable conformers.
-
Hypothesis Generation: Models can be used to generate hypotheses about the structure-activity relationships of molecules, which can then be tested experimentally.
Types of Molecular Modeling Techniques
Several computational methods are employed for molecular structure prediction. The choice of method depends on the system's size, complexity, and the desired level of accuracy.
1. Molecular Mechanics (MM)
MM methods utilize classical mechanics principles to describe molecular systems. They represent atoms as spheres with defined masses and charges, and bonds as springs with defined force constants. The potential energy of the system is calculated as a sum of various energy terms, including bond stretching, angle bending, torsional rotation, and non-bonded interactions (van der Waals forces and electrostatic interactions). MM methods are computationally inexpensive and can handle large systems, making them suitable for conformational analysis, molecular dynamics simulations, and docking studies. However, their accuracy is limited because they do not explicitly consider electron distribution.
2. Quantum Mechanics (QM) Methods
QM methods, also known as ab initio methods, solve the Schrödinger equation to determine the electronic structure of molecules. This provides a more accurate description of molecular properties compared to MM methods. However, QM calculations are computationally expensive and are typically limited to smaller molecules. Popular QM methods include:
-
Hartree-Fock (HF): A relatively simple method that considers electron correlation only approximately.
-
Density Functional Theory (DFT): A widely used method that offers a good balance between accuracy and computational cost. DFT is particularly useful for predicting the structures and energies of ground-state molecules.
-
Post-Hartree-Fock Methods: These methods include configuration interaction (CI) and coupled cluster (CC) methods, providing higher accuracy but at significantly increased computational cost. They are typically used for smaller, high-priority systems.
3. Hybrid QM/MM Methods
Hybrid QM/MM methods combine the strengths of QM and MM methods. The critical part of the molecule, such as the reactive site, is treated with QM methods, while the remaining part of the molecule and the environment are treated with MM methods. This approach allows for the simulation of larger systems with improved accuracy while maintaining computational feasibility.
4. Machine Learning (ML) Methods
Recently, machine learning (ML) has emerged as a powerful tool in molecular structure prediction. ML algorithms are trained on large datasets of known molecular structures and properties to learn patterns and predict new structures. ML methods can be particularly useful for predicting structures of molecules with complex conformations or for handling large datasets. Several types of ML algorithms are being employed, including:
-
Neural Networks: Powerful algorithms that can learn complex relationships between molecular features and structures.
-
Support Vector Machines (SVM): Effective methods for classification and regression tasks in molecular modeling.
-
Graph Neural Networks (GNN): Specifically designed to handle graph-structured data like molecular structures, they've shown promise in predicting properties and structures.
Practical Considerations in the Lab
Implementing computational modeling in a lab setting involves several practical considerations:
-
Software Selection: Several software packages are available for molecular modeling, each with its strengths and weaknesses. The choice of software depends on the specific needs of the research project, the user's expertise, and available computational resources. Popular packages include Gaussian, GAMESS, ORCA, and others that incorporate various QM and MM methods, as well as specialized software focused on ML techniques.
-
Computational Resources: Performing complex molecular modeling calculations requires significant computational resources, such as powerful computers and specialized software. Cloud computing resources can provide cost-effective access to high-performance computing clusters.
-
Data Preparation: Accurate input data is crucial for successful modeling. This involves obtaining high-quality experimental data and preparing appropriate input files for the chosen software.
-
Model Validation: It's essential to validate the accuracy of the model by comparing its predictions to experimental data. Several validation metrics are available, including root-mean-square deviation (RMSD) and correlation coefficients.
-
Interpretation of Results: Interpreting the results of molecular modeling requires a thorough understanding of the chosen methodology and its limitations. Careful consideration is needed to avoid overinterpreting results.
Applications in Different Research Areas
The application of molecular modeling in predicting molecular structure extends across numerous scientific disciplines:
-
Drug Discovery: Modeling is crucial for designing and optimizing drug candidates. It allows researchers to predict the binding affinity and efficacy of potential drugs, speeding up the drug development process and reducing the cost of experimental screening.
-
Materials Science: Modeling assists in designing novel materials with desired properties. It can help in predicting the structure and stability of materials, leading to the development of new polymers, catalysts, and other materials with tailored characteristics.
-
Biochemistry: Molecular modeling is indispensable in studying biological molecules such as proteins and nucleic acids. It can help to understand the structure-function relationships of these molecules and predict their interactions with other molecules.
-
Environmental Chemistry: Modeling is used to study the fate and transport of pollutants in the environment. This allows for the prediction of the environmental impact of chemical substances and the development of effective remediation strategies.
Future Trends and Challenges
The field of molecular structure prediction is constantly evolving. Several emerging trends will shape the future of this field:
-
Increased integration of ML and AI: Machine learning is becoming increasingly important in molecular modeling, enabling the prediction of complex structures and properties with greater speed and accuracy. The development of more advanced algorithms and the availability of larger datasets will further enhance the power of ML in molecular structure prediction.
-
Development of more accurate and efficient QM methods: Research is ongoing to develop more accurate and computationally efficient QM methods that can handle larger systems.
-
Enhanced visualization and interpretation tools: Advancements in visualization techniques will facilitate better interpretation of the complex data generated by molecular modeling simulations.
-
Integration with experimental techniques: The combined use of computational modeling and experimental techniques will continue to drive progress in the field. This synergistic approach allows for validation of theoretical predictions and further refinement of computational models.
Conclusion
Computational modeling plays a vital role in predicting molecular structures in laboratory experiments. It offers numerous advantages over traditional experimental methods, including speed, efficiency, and cost-effectiveness. The choice of the appropriate modeling technique depends on several factors, including the size and complexity of the molecule and the required accuracy. Integrating computational modeling into a lab's research workflow can significantly enhance the efficiency and effectiveness of molecular structure determination, leading to advancements across various scientific disciplines. However, it is crucial to remember the limitations of each method and to always validate predictions with experimental data for reliable results. The future of this field lies in the continued development and integration of advanced algorithms, computational resources, and innovative experimental approaches.
Latest Posts
Latest Posts
-
Stockholders Have The Right To At Stockholders Meetings
Apr 26, 2025
-
The Advantages To The Corporate Form Of Business Include
Apr 26, 2025
-
Regularly Calibrating The Thermometers Used To Check Food Temperatures
Apr 26, 2025
-
Which Statement About File History Is Incorrect
Apr 26, 2025
-
Identify And Describe Three Reasons For The Growth Of Conservatism
Apr 26, 2025
Related Post
Thank you for visiting our website which covers about Using Models To Predict Molecular Structure Lab . We hope the information provided has been useful to you. Feel free to contact us if you have any questions or need further assistance. See you next time and don't miss to bookmark.