Volume Complexity Knowledge And Uncertainty Are All Qualities Of What
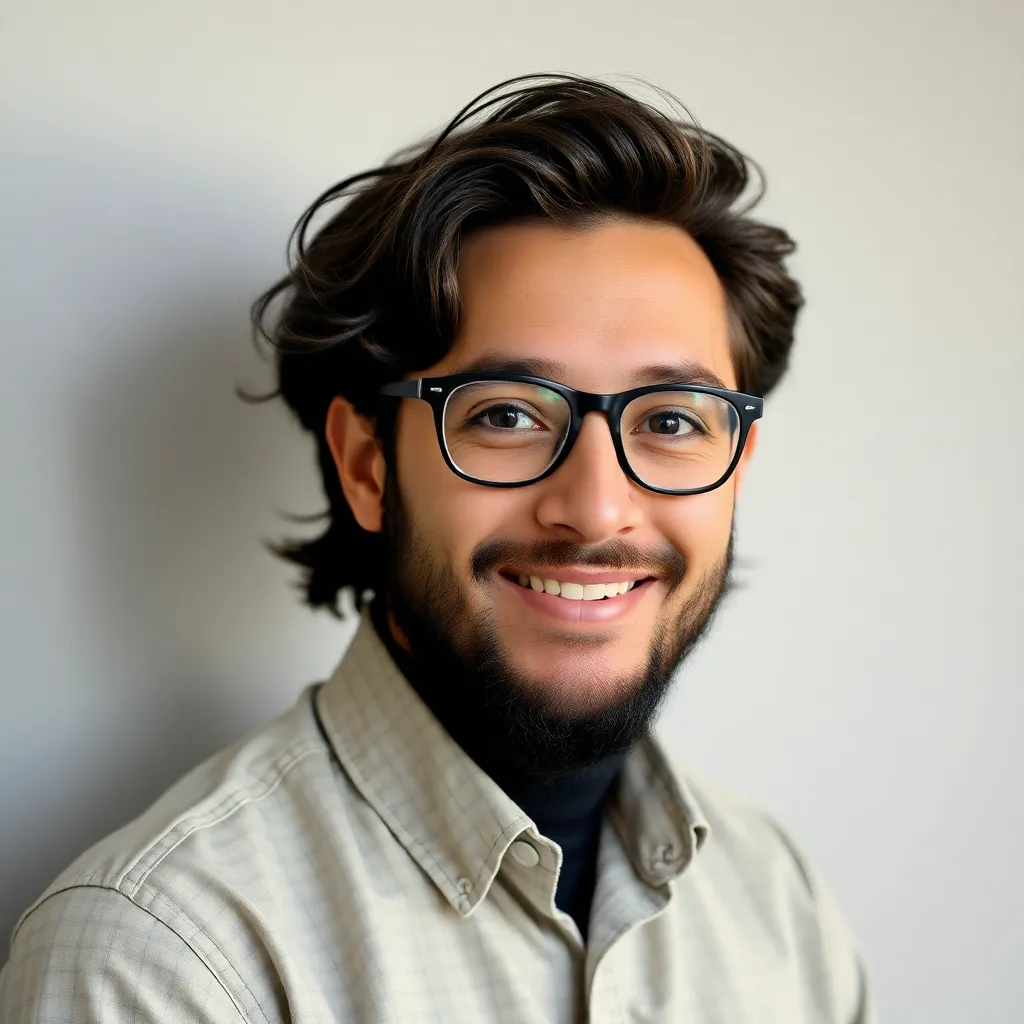
Breaking News Today
May 11, 2025 · 6 min read
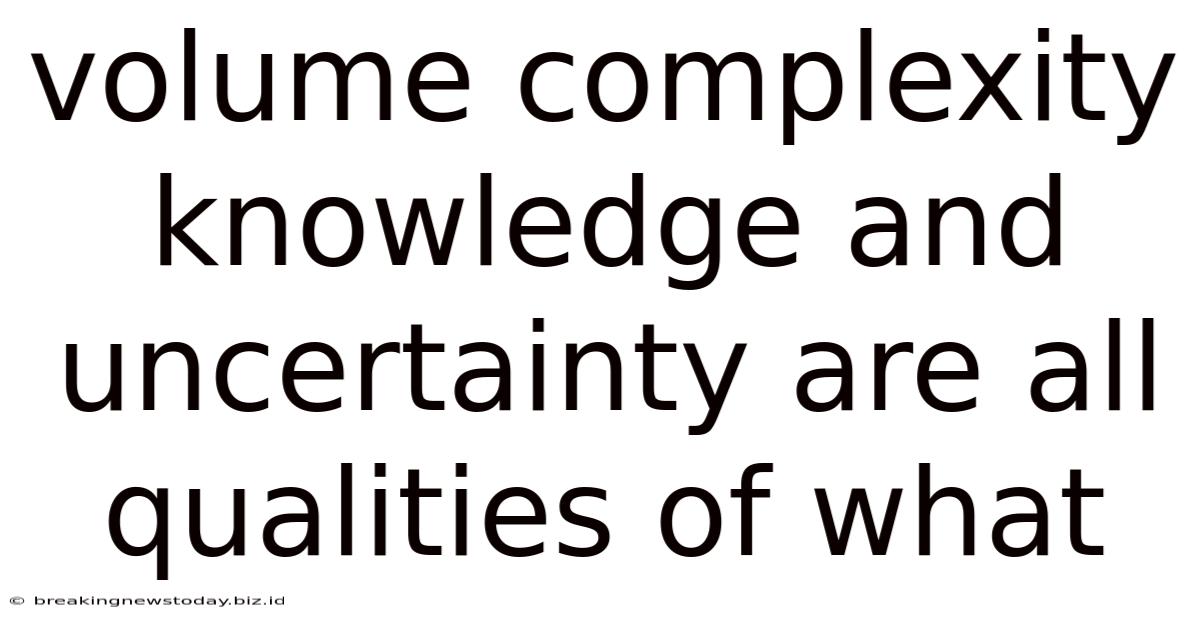
Table of Contents
Volume, Complexity, Knowledge, and Uncertainty: The Defining Qualities of Big Data
The world is drowning in data. From social media interactions to scientific experiments, from financial transactions to satellite imagery, the sheer volume of information generated daily is staggering. This isn't just about having more data; it's about a fundamentally different kind of data, characterized by its volume, complexity, velocity, veracity, and variability – often referred to as the five Vs of Big Data. But at the heart of understanding big data lies the understanding of its inherent qualities: volume, complexity, knowledge, and uncertainty. These four factors intertwine to define the unique challenges and opportunities presented by this revolutionary resource.
Understanding the Four Pillars of Big Data
Let's delve deeper into each of these four pillars:
1. Volume: The Sheer Scale of Information
The sheer volume of data is perhaps the most immediately obvious characteristic of big data. We're talking about datasets measured in petabytes, exabytes, and even zettabytes – magnitudes far beyond the capacity of traditional data processing tools. This scale presents several challenges:
- Storage: Storing such massive datasets requires specialized infrastructure and efficient storage solutions. Traditional databases simply can't handle the load.
- Processing: Analyzing petabytes of data necessitates powerful processing capabilities, often involving distributed computing frameworks like Hadoop and Spark.
- Cost: The cost associated with storing, processing, and managing such vast amounts of data can be substantial.
However, this immense volume also presents opportunities. The more data we have, the more accurate and insightful our analyses can be. Patterns and trends that would be invisible in smaller datasets can emerge with greater clarity in the sheer volume of big data.
2. Complexity: The Intricacies of Data Structures
Beyond sheer size, the complexity of big data is equally significant. This complexity manifests in several ways:
- Data Variety: Big data comes in many forms – structured (e.g., relational databases), semi-structured (e.g., XML, JSON), and unstructured (e.g., text, images, videos). Integrating and analyzing data from these disparate sources requires sophisticated techniques.
- Data Velocity: The speed at which data is generated and processed is crucial. Real-time analytics, often a requirement for big data applications, demand efficient processing pipelines capable of handling high-velocity data streams.
- Data Veracity: Ensuring the accuracy and reliability of data is paramount. Big data often comes from diverse and potentially unreliable sources, necessitating careful data cleaning, validation, and integration processes.
- Data Variability: The inherent changeability of data is another facet of its complexity. Data patterns and trends can shift over time, requiring adaptive analytical techniques capable of capturing these dynamic changes.
This complexity demands advanced analytical methods beyond traditional statistical techniques. Machine learning, deep learning, and other artificial intelligence (AI) techniques are becoming increasingly crucial for extracting meaningful insights from complex big data.
3. Knowledge: Extracting Meaning from Data
The ultimate goal of analyzing big data is to gain knowledge. Raw data, however voluminous or complex, is useless without interpretation. Knowledge extraction involves several key steps:
- Data Mining: This involves using algorithms to discover hidden patterns, anomalies, and trends within the data.
- Data Visualization: Presenting data in a clear and understandable way is crucial for communicating insights to stakeholders. Effective visualizations can reveal patterns that would be invisible in raw data.
- Predictive Modeling: Big data can be used to build models that predict future events or behaviors, providing valuable insights for decision-making.
- Knowledge Representation: Organizing and structuring the extracted knowledge into a usable format, such as knowledge graphs or ontologies, is crucial for efficient access and retrieval.
The ability to extract meaningful knowledge from big data has profound implications across various fields, from healthcare and finance to manufacturing and environmental science.
4. Uncertainty: Dealing with Imperfect Information
Despite the vast potential of big data, it's crucial to acknowledge the inherent uncertainty associated with it. This uncertainty stems from several sources:
- Incomplete Data: Datasets are rarely complete; missing values are common.
- Inconsistent Data: Data from different sources may have different formats and definitions, leading to inconsistencies.
- Noisy Data: Data can be contaminated with errors or inaccuracies.
- Ambiguous Data: The meaning of data may not always be clear.
Dealing with uncertainty requires robust statistical methods and AI techniques that can handle incomplete, inconsistent, and noisy data. Probabilistic models, Bayesian inference, and fuzzy logic are all valuable tools for managing uncertainty in big data analysis. Understanding and quantifying uncertainty is critical for making reliable and informed decisions based on big data insights.
The Interplay of Volume, Complexity, Knowledge, and Uncertainty
These four elements are inextricably linked. The sheer volume of data increases its complexity, making the extraction of knowledge more challenging and introducing more uncertainty. Furthermore, attempts to reduce uncertainty can increase the complexity of analysis and the volume of data considered. This intricate interplay highlights the need for sophisticated analytical techniques and robust data management strategies.
Big Data's Impact Across Industries
The implications of big data extend far beyond the realm of data science. Its transformative power is reshaping industries globally:
- Healthcare: Big data is revolutionizing healthcare through improved diagnostics, personalized medicine, drug discovery, and public health surveillance.
- Finance: Banks and financial institutions leverage big data for fraud detection, risk management, algorithmic trading, and customer relationship management.
- Retail: Retailers use big data to understand customer preferences, optimize pricing strategies, personalize marketing campaigns, and improve supply chain management.
- Manufacturing: Big data enables predictive maintenance, quality control improvements, and optimization of production processes in manufacturing.
- Transportation: Big data drives innovations in traffic management, logistics optimization, and autonomous driving.
These are just a few examples; the applications of big data are virtually limitless.
Addressing the Challenges of Big Data
While the potential benefits of big data are immense, several challenges need to be addressed:
- Data Security and Privacy: Protecting sensitive data is crucial. Big data applications must comply with data privacy regulations and employ robust security measures.
- Data Governance: Establishing clear policies and procedures for managing and governing big data is essential for ensuring data quality, consistency, and compliance.
- Skill Gap: The demand for skilled data scientists, data engineers, and other big data professionals far exceeds the supply.
- Ethical Considerations: The ethical implications of big data, such as algorithmic bias and discrimination, must be carefully considered.
Overcoming these challenges requires collaboration between researchers, industry professionals, policymakers, and the public.
The Future of Big Data
The future of big data is bright, with ongoing advancements in data storage, processing, and analytics technologies. The convergence of big data with artificial intelligence, machine learning, and the Internet of Things (IoT) promises even more transformative possibilities. As data volumes continue to explode and analytical techniques become more sophisticated, the potential for extracting valuable knowledge and insights from big data will only grow.
In conclusion, volume, complexity, knowledge, and uncertainty are the defining qualities of big data. Understanding these interconnected aspects is essential for leveraging the power of big data to solve complex problems, drive innovation, and create value across industries. The journey into the realm of big data is a continuous exploration, demanding adaptability, innovation, and a commitment to responsible data management.
Latest Posts
Latest Posts
-
Which Of The Following Is Shown In The Picture
May 12, 2025
-
You May Turn Left On A Red Light If
May 12, 2025
-
A Presidents Power Has Largely Depended On
May 12, 2025
-
The Aria Lucevan Le Stelle Is Composed For A An
May 12, 2025
-
Saving Information For Future Use Is Called
May 12, 2025
Related Post
Thank you for visiting our website which covers about Volume Complexity Knowledge And Uncertainty Are All Qualities Of What . We hope the information provided has been useful to you. Feel free to contact us if you have any questions or need further assistance. See you next time and don't miss to bookmark.