Watson's Achilles: Second Operation
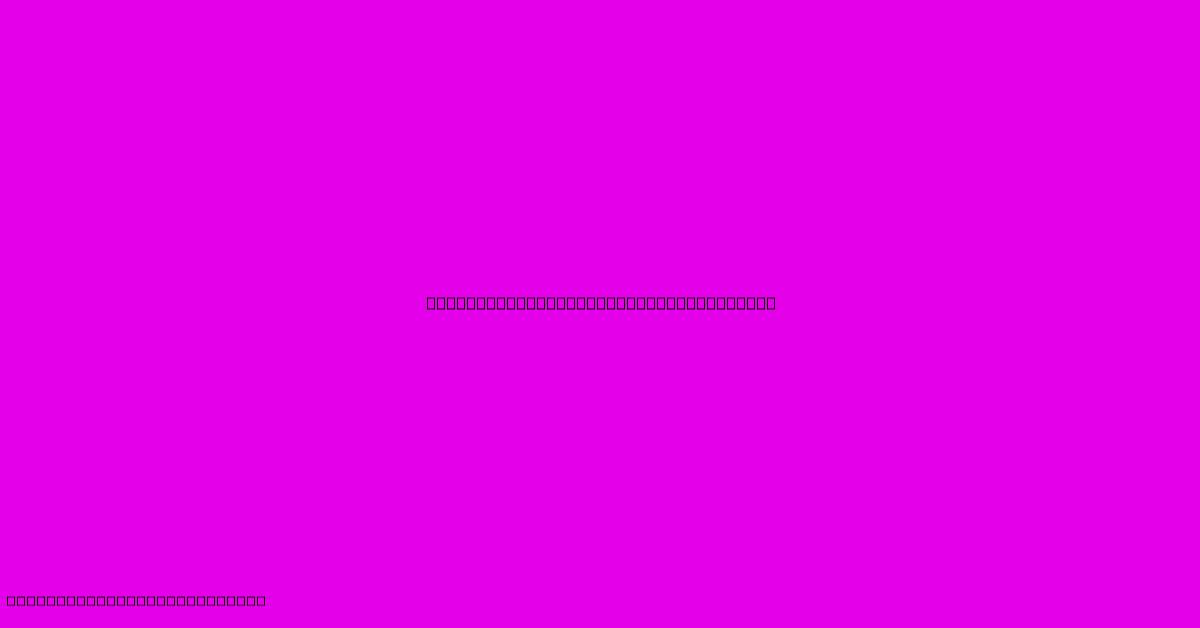
Discover more detailed and exciting information on our website. Click the link below to start your adventure: Visit Best Website breakingnewstoday.biz.id. Don't miss out!
Table of Contents
Watson's Achilles: Second Operation – A Deep Dive into IBM's AI Evolution
IBM Watson. The name conjures images of a powerful, all-knowing AI, capable of conquering complex challenges in medicine, business, and beyond. But even the most sophisticated artificial intelligence has its weaknesses. This article delves into what could be considered Watson's "second operation"—a necessary refocusing and reimagining of its capabilities after its initial, ambitious rollout encountered significant hurdles. We'll explore the reasons behind this evolution, examine the strategic shifts IBM undertook, and analyze the current state of Watson's impact.
The Promise and the Pitfalls of Early Watson
The initial promise of Watson was immense. Leveraging IBM's vast computing power and advanced natural language processing, Watson was presented as a revolutionary tool capable of analyzing vast datasets, identifying patterns, and providing insightful solutions. Early successes, particularly in Jeopardy!, fueled the hype, creating the impression of a near-future where AI would solve humanity's most pressing problems.
However, translating this initial success into real-world applications proved far more challenging. Early attempts to deploy Watson in healthcare, for example, revealed significant limitations. The complexity of medical data, the nuances of human language, and the need for rigorous validation and regulatory compliance all posed major obstacles. Watson's initial deployments in oncology, while generating some positive results in specific areas, often fell short of expectations. The system struggled with the ambiguity of medical language, leading to inaccurate diagnoses and treatment recommendations in certain cases. This led to criticisms regarding its reliability and effectiveness, ultimately impacting its adoption and tarnishing its reputation.
Identifying the Problems: A Critical Analysis
Several key factors contributed to Watson's early struggles:
-
Data Quality and Availability: Watson's performance relies heavily on the quality and quantity of data it is trained on. Inconsistent, incomplete, or biased data sets can lead to inaccurate and unreliable results. This was particularly problematic in the healthcare sector, where data is often fragmented, poorly standardized, and subject to privacy regulations.
-
Overly Ambitious Expectations: The initial hype surrounding Watson created unrealistic expectations about its capabilities. Many believed it would be a standalone, self-sufficient AI system capable of instantly providing perfect solutions. This misconception led to disappointment when Watson failed to live up to these inflated promises.
-
Lack of Integration and Interoperability: Integrating Watson into existing healthcare workflows and systems proved to be a significant challenge. The system often required significant customization and adaptation, leading to increased costs and implementation difficulties. Interoperability with different Electronic Health Record (EHR) systems also posed a substantial hurdle.
-
The Need for Human Expertise: While Watson could process vast quantities of data, it lacked the critical thinking and contextual understanding of human clinicians. The system needed human oversight and interpretation to ensure accurate and appropriate application of its findings.
Watson's Second Operation: A Strategic Realignment
Recognizing the limitations of its initial approach, IBM embarked on a significant strategic shift. This "second operation" involved a reevaluation of Watson's capabilities, a refinement of its applications, and a more realistic assessment of its potential. This shift encompassed several key changes:
-
Focus on Specific Applications: Instead of attempting to be a universal AI solution, IBM focused on developing Watson for specific, well-defined applications. This allowed for more targeted development and more effective integration into existing workflows.
-
Emphasis on Collaboration with Human Experts: IBM recognized the crucial role of human expertise in maximizing Watson's potential. The focus shifted towards developing AI systems that augment human capabilities rather than replacing them. This collaborative approach emphasized human-in-the-loop systems where clinicians guide and validate Watson's recommendations.
-
Improved Data Management and Validation: IBM invested heavily in improving the quality and accessibility of data used to train Watson. This included implementing robust data validation processes and developing tools to standardize and integrate data from diverse sources.
-
Enhanced Explainability and Transparency: IBM worked to make Watson's decision-making processes more transparent and understandable. This involved developing techniques to explain how Watson arrives at its conclusions, increasing trust and facilitating collaboration with human experts.
Current Applications and Future Directions
Today, Watson is deployed across a broader range of industries, focusing on more specific and achievable goals. Examples include:
-
Financial Services: Watson is used to analyze market trends, detect fraud, and personalize customer experiences.
-
Customer Service: Watson powers chatbots and virtual assistants, providing automated customer support.
-
Research and Development: Watson is utilized to accelerate scientific discovery by analyzing large datasets and identifying new patterns.
While the initial grand vision of a universal AI problem-solver has been tempered, Watson continues to evolve and adapt. IBM's emphasis on collaboration with human experts, a refined focus on specific applications, and improved data management techniques have contributed to its increased effectiveness and broader adoption.
The Lessons Learned
Watson's journey serves as a valuable case study in the development and deployment of complex AI systems. It highlights the importance of:
-
Realistic Expectations: Avoiding over-hyping AI capabilities and setting achievable goals.
-
Data Quality: Investing in robust data management and validation processes.
-
Human-in-the-Loop Systems: Recognizing the crucial role of human expertise and collaboration.
-
Iterative Development: Embracing a continuous improvement approach and adapting to evolving challenges.
Watson's "second operation" represents a significant turning point in its development. By acknowledging its limitations and adapting its strategy, IBM has laid the foundation for a more sustainable and impactful future for this powerful AI technology. The journey underscores that even the most advanced AI systems require continuous refinement and adaptation to achieve their full potential. The future of Watson, and indeed the future of AI more broadly, lies in embracing collaboration, managing expectations, and fostering responsible innovation.
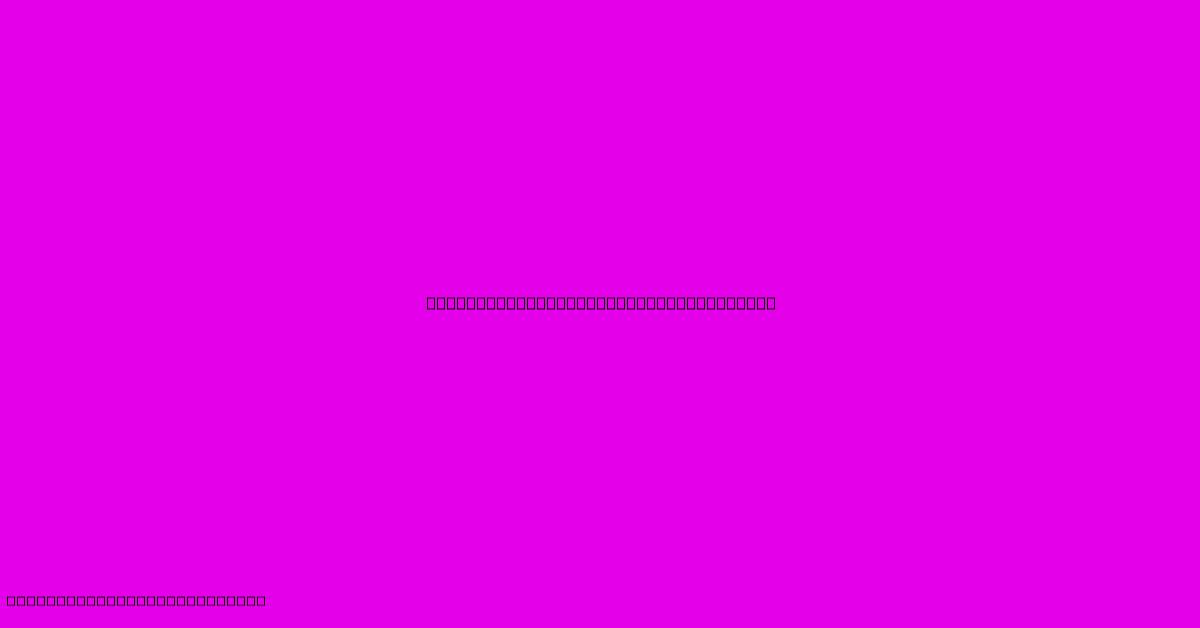
Thank you for visiting our website wich cover about Watson's Achilles: Second Operation. We hope the information provided has been useful to you. Feel free to contact us if you have any questions or need further assistance. See you next time and dont miss to bookmark.
Also read the following articles
Article Title | Date |
---|---|
Downtown San Francisco Earthquake Tremors | Jan 12, 2025 |
Cotton Bowl Mannings Fourth Down Saves Texas | Jan 12, 2025 |
Watsons Second Achilles Surgery | Jan 12, 2025 |
New 2025 Tesla Model Y Juniper Unveiled | Jan 12, 2025 |
See The 2025 Tesla Model Y Juniper | Jan 12, 2025 |