What Can You Tell About The Mean Of Each Distribution
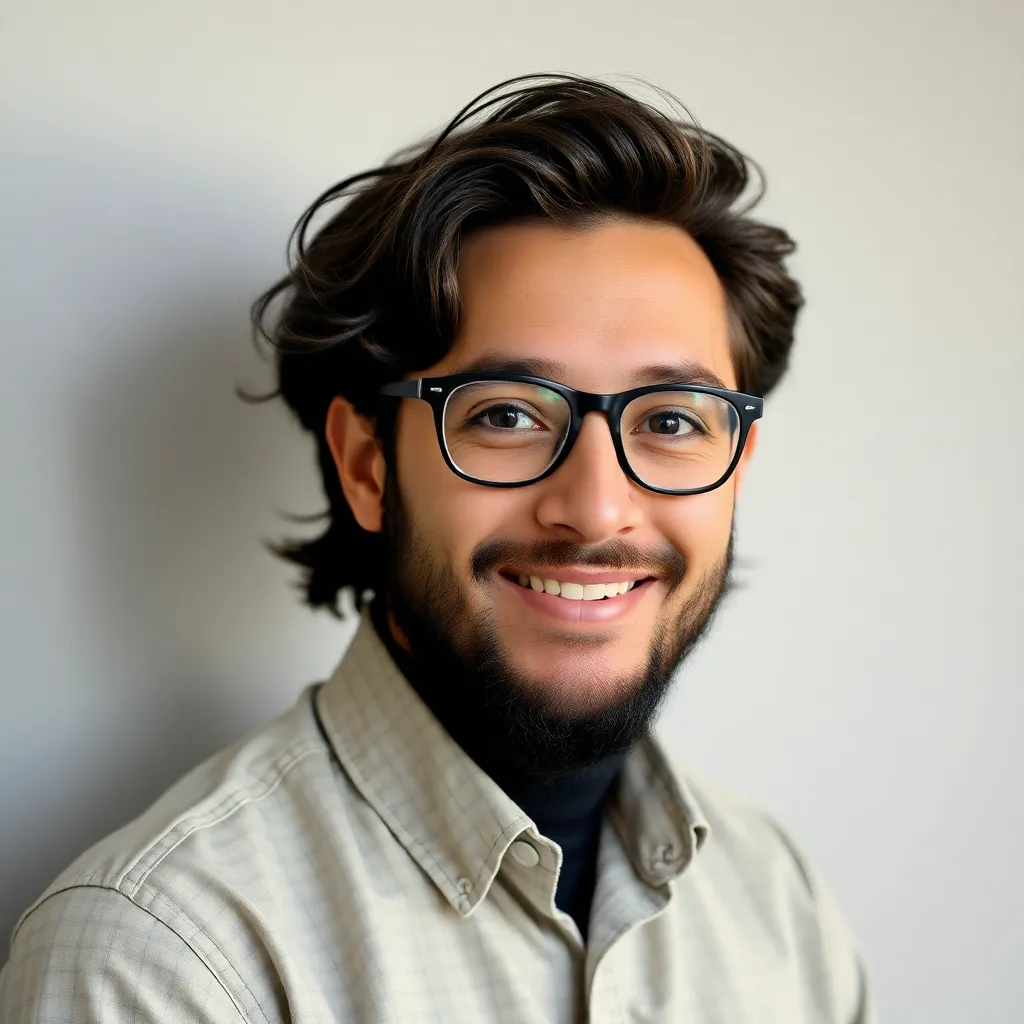
Breaking News Today
May 12, 2025 · 7 min read
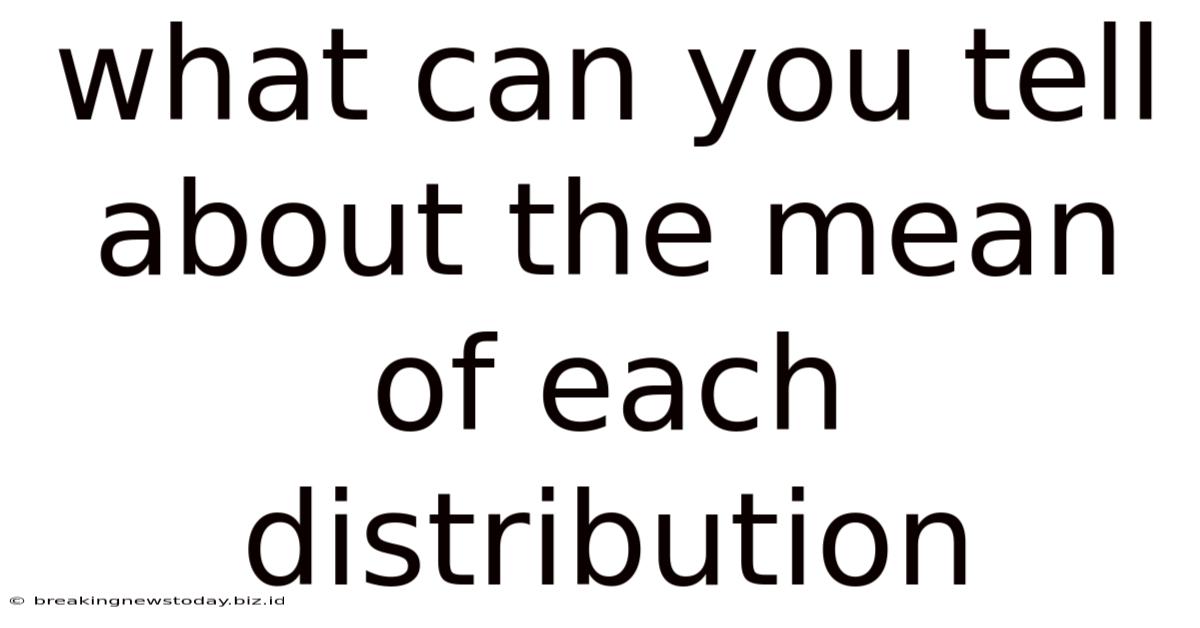
Table of Contents
What Can You Tell About the Mean of Each Distribution?
Understanding the mean, or average, of a distribution is fundamental in statistics. It's a crucial measure of central tendency, offering insights into the typical value of a dataset. However, the interpretation and significance of the mean vary dramatically depending on the type of distribution you're dealing with. This article delves deep into the nuances of the mean across various distributions, emphasizing the importance of considering the distribution's shape and properties before drawing conclusions.
The Mean: A Foundation of Statistical Analysis
Before we explore specific distributions, let's solidify our understanding of the mean itself. The mean (often denoted as μ for a population and x̄ for a sample) is calculated by summing all the values in a dataset and dividing by the number of values. It represents the balancing point of the distribution; if you were to visualize the data points on a number line, the mean would be the point where the distribution is perfectly balanced.
Key Characteristics of the Mean:
- Sensitive to outliers: This is a crucial point. Extreme values, or outliers, can significantly influence the mean, pulling it away from the "typical" value. This is especially important in skewed distributions.
- Only applicable to numerical data: The mean is calculated on numerical data; it cannot be used with categorical data.
- Easily calculated: The mathematical calculation of the mean is straightforward.
- Useful for comparison: Means from different datasets allow for easy comparison of central tendencies.
Exploring the Mean Across Different Distributions
The interpretation of the mean differs significantly based on the type of distribution. Let's examine some common distributions:
1. Normal Distribution (Gaussian Distribution)
The normal distribution is arguably the most famous and widely used distribution in statistics. It's characterized by its bell shape, symmetry around the mean, and a defined standard deviation that describes its spread.
Mean in a Normal Distribution:
In a perfectly normal distribution, the mean, median, and mode are all equal. This means the mean perfectly represents the center of the distribution, providing a robust measure of the typical value. The mean's position on the x-axis defines the center of the bell curve. Knowing the mean and standard deviation completely defines the normal distribution.
Example: The heights of adult women often follow an approximately normal distribution. The mean height would represent the average height of adult women.
2. Uniform Distribution
A uniform distribution is characterized by its constant probability density over a specified range. Every value within the range has an equal chance of occurring.
Mean in a Uniform Distribution:
The mean of a uniform distribution is simply the midpoint of the range. This is because each value contributes equally to the average.
Example: Generating a random number between 0 and 10 using a computer's random number generator would follow a uniform distribution. The mean would be 5.
3. Skewed Distributions (Positive and Negative Skew)
Skewed distributions are asymmetrical; they have a "tail" extending to one side. There are two types: positively skewed (right-skewed) and negatively skewed (left-skewed).
Mean in Skewed Distributions:
-
Positively Skewed: In a positively skewed distribution, the mean is greater than the median and mode. The long tail on the right, containing outliers, pulls the mean towards higher values. The mean is not a reliable representation of the typical value in this case. The median is usually a better measure of central tendency.
-
Negatively Skewed: In a negatively skewed distribution, the mean is less than the median and mode. The long tail on the left, again influenced by outliers, pulls the mean towards lower values. Similar to positive skew, the mean is less representative of the typical value, and the median is preferred.
Example: Income distribution often shows a positive skew, with a few high earners pulling the mean higher than the typical income level.
4. Exponential Distribution
The exponential distribution is often used to model the time until an event occurs in a Poisson process (events happening at a constant average rate).
Mean in an Exponential Distribution:
The mean of an exponential distribution is inversely proportional to the rate parameter (λ). A higher rate parameter means a lower mean, indicating events occur more frequently. The mean is equal to the reciprocal of lambda (1/λ).
Example: The time until a machine breaks down, if breakdowns occur at a constant average rate, could be modeled by an exponential distribution. The mean would represent the average time until breakdown.
5. Binomial Distribution
The binomial distribution describes the probability of obtaining a certain number of successes in a fixed number of independent Bernoulli trials (trials with only two possible outcomes, success or failure).
Mean in a Binomial Distribution:
The mean of a binomial distribution is simply the product of the number of trials (n) and the probability of success in each trial (p). It represents the expected number of successes. Mean = n*p
Example: Flipping a coin 10 times (n=10) and counting the number of heads (p=0.5). The mean would be 5, meaning you would expect 5 heads on average.
6. Poisson Distribution
The Poisson distribution models the probability of a given number of events occurring in a fixed interval of time or space if these events occur with a known average rate and independently of the time since the last event.
Mean in a Poisson Distribution:
The mean of a Poisson distribution is equal to its parameter, λ (lambda), which represents the average rate of events.
Example: The number of cars passing a certain point on a highway in an hour. If the average rate is 10 cars per hour, then the mean of the Poisson distribution is 10.
7. Beta Distribution
The beta distribution is a continuous probability distribution defined on the interval [0, 1], making it useful for modeling proportions or probabilities.
Mean in a Beta Distribution:
The mean of a beta distribution with parameters α and β is α / (α + β).
Example: Modeling the probability of success in a binary event, where α and β represent the number of successes and failures observed.
8. Gamma Distribution
The gamma distribution is a flexible distribution used to model positive, continuous data, often related to waiting times or durations.
Mean in a Gamma Distribution:
The mean of a gamma distribution with shape parameter k and scale parameter θ is kθ.
Example: Modeling the time until failure of a component, where k and θ are parameters defining the shape and scale of the distribution.
Importance of Understanding Distribution Shape
The shape of the distribution profoundly impacts the interpretation of the mean. In symmetrical distributions like the normal distribution, the mean provides a central and reliable measure. However, in skewed distributions, the mean can be heavily influenced by outliers, making it less representative of the typical value. In these situations, the median or mode often provide a more accurate reflection of the central tendency.
Choosing the Right Measure of Central Tendency
The choice between mean, median, and mode depends entirely on the data and the research question.
- Use the mean: When the data is approximately normally distributed and there are no significant outliers.
- Use the median: When the data is skewed or contains outliers, as it is less sensitive to extreme values.
- Use the mode: When dealing with categorical data or when identifying the most frequent value is the primary interest.
Conclusion: The Mean in Context
The mean is a powerful tool in statistical analysis, but its interpretation must always be considered within the context of the underlying distribution. Understanding the distribution's shape, presence of outliers, and the specific characteristics of the distribution type (normal, skewed, exponential, etc.) is crucial for drawing meaningful conclusions from the mean. By carefully considering these factors, you can use the mean effectively to gain valuable insights into your data. Remember that the mean is just one piece of the puzzle; combining it with other descriptive statistics and visualization techniques provides a more complete and nuanced understanding of the data.
Latest Posts
Latest Posts
-
A Partial Bath Includes Washing A Residents
May 12, 2025
-
Which Of The Following Describes A Net Lease
May 12, 2025
-
Nurse Logic 2 0 Knowledge And Clinical Judgment
May 12, 2025
-
Panic Disorder Is Characterized By All Of The Following Except
May 12, 2025
-
Positive Individual Traits Can Be Taught A True B False
May 12, 2025
Related Post
Thank you for visiting our website which covers about What Can You Tell About The Mean Of Each Distribution . We hope the information provided has been useful to you. Feel free to contact us if you have any questions or need further assistance. See you next time and don't miss to bookmark.