What Conclusion Do The Data In The Table Support
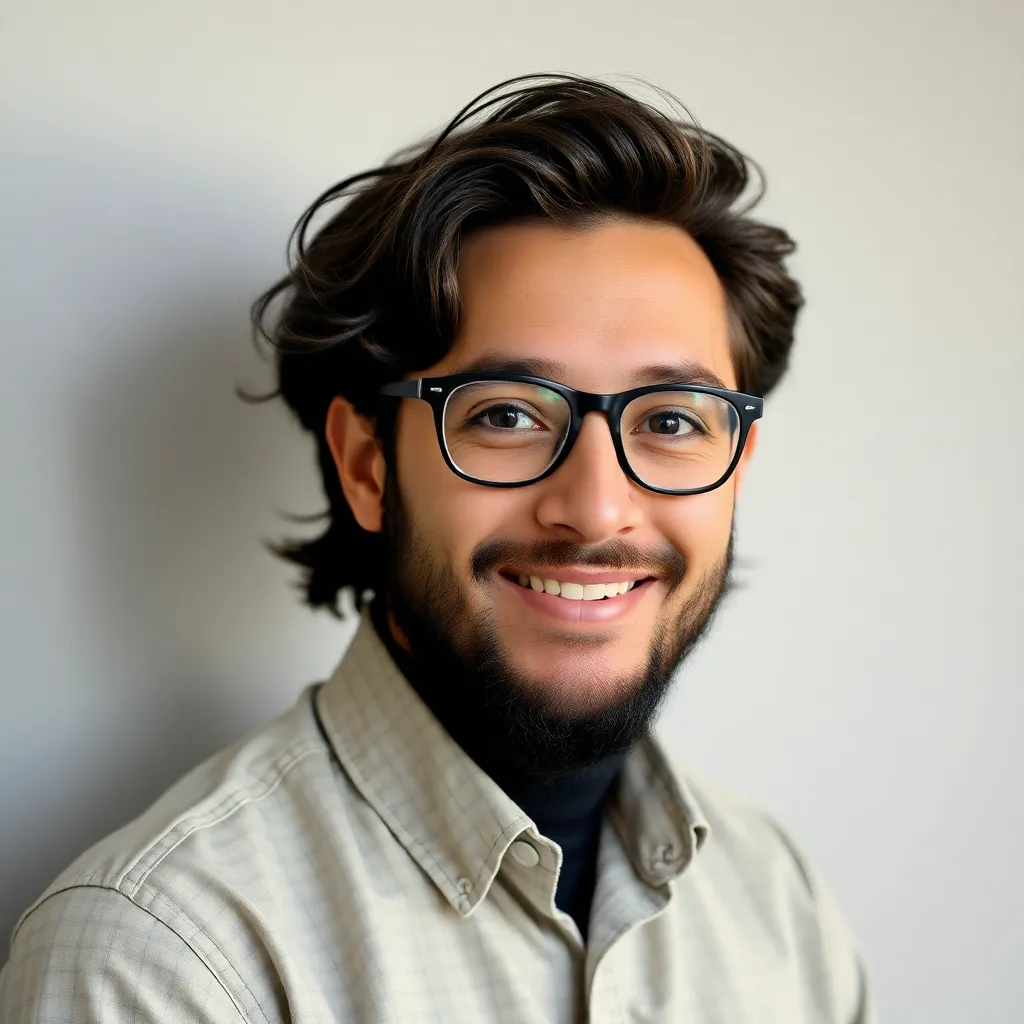
Breaking News Today
May 11, 2025 · 6 min read
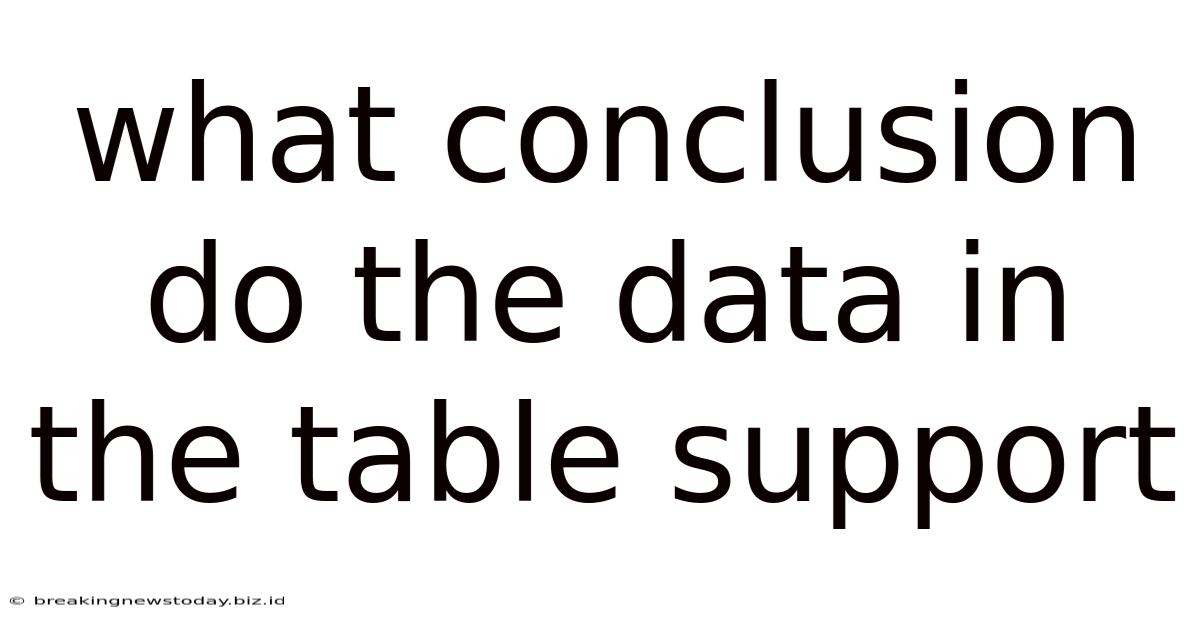
Table of Contents
What Conclusions Do the Data in the Table Support? A Guide to Data Analysis and Interpretation
Analyzing data is a crucial skill across numerous fields, from scientific research to business analytics. Simply collecting data isn't enough; understanding what the data means is where the real insights lie. This article will guide you through the process of drawing meaningful conclusions from tabular data, emphasizing critical thinking, statistical reasoning, and effective communication of findings. We'll explore various analytical approaches, discuss potential pitfalls, and highlight best practices for presenting your conclusions.
Understanding the Context: The Foundation of Data Interpretation
Before diving into the numbers, it's crucial to understand the context surrounding your data. Ask yourself these key questions:
1. What is the research question or objective?
What are you trying to discover or prove with this data? A clearly defined objective guides your analysis and helps you focus on relevant conclusions. For example, if you're analyzing sales data, your objective might be to identify the best-selling product, understand seasonal trends, or pinpoint areas for improvement.
2. How was the data collected?
The data collection method significantly impacts the validity and reliability of your conclusions. Was it a random sample? Were there any biases in the collection process? Understanding the methodology helps you assess the limitations and potential sources of error in your data. A poorly designed survey, for instance, can lead to misleading conclusions.
3. What are the variables involved?
Identify the independent and dependent variables. The independent variable is the factor you manipulate or observe (e.g., advertising spend), while the dependent variable is the outcome you measure (e.g., sales). Understanding the relationships between variables is essential for drawing accurate conclusions.
4. Who is the target audience?
Tailoring your conclusions to your audience is crucial for effective communication. A scientific journal article will require a different level of detail and statistical rigor than a presentation to a board of directors.
Analyzing the Data: Tools and Techniques
Once you understand the context, you can start analyzing the data itself. The specific techniques you use will depend on the type of data you have (categorical, numerical, etc.) and your research questions.
1. Descriptive Statistics: Summarizing the Data
Descriptive statistics provide a summary of the main features of your data. Key measures include:
-
Measures of central tendency: Mean, median, and mode describe the center of your data distribution. The choice of measure depends on the data's distribution (e.g., the median is less sensitive to outliers than the mean).
-
Measures of dispersion: Range, variance, and standard deviation describe the spread or variability of your data. High dispersion indicates greater variability and less certainty in your conclusions.
-
Frequency distributions and histograms: These visually represent the distribution of your data, showing the frequency of different values or ranges of values. They can reveal patterns, outliers, and the overall shape of the distribution (e.g., normal, skewed).
2. Inferential Statistics: Drawing Generalizations
If your goal is to generalize your findings beyond the specific dataset, you'll need inferential statistics. These techniques allow you to make inferences about a population based on a sample of data. Common inferential methods include:
-
Hypothesis testing: This involves formulating a hypothesis (a testable statement) and using statistical tests to determine whether the data supports or refutes it. Examples include t-tests, ANOVA, and chi-square tests.
-
Regression analysis: This technique examines the relationship between a dependent variable and one or more independent variables. It can be used to predict future outcomes or understand the impact of different factors.
-
Correlation analysis: This assesses the strength and direction of the linear relationship between two variables. Correlation does not imply causation.
3. Visualization: Communicating Insights Effectively
Visualizations are essential for communicating your findings clearly and concisely. Effective visualizations make it easier to identify patterns, trends, and outliers that might be missed in raw data. Common visualization techniques include:
-
Bar charts: Useful for comparing categorical data.
-
Line graphs: Show trends over time.
-
Scatter plots: Illustrate the relationship between two numerical variables.
-
Pie charts: Represent proportions of a whole.
Drawing Conclusions: A Cautious Approach
Drawing conclusions from data requires careful consideration and a cautious approach. Avoid these common pitfalls:
1. Correlation vs. Causation: Don't Assume Causality
Just because two variables are correlated doesn't mean one causes the other. There may be a third, unobserved variable influencing both. For example, ice cream sales and crime rates might be positively correlated, but this doesn't mean ice cream causes crime. Both are likely influenced by a third variable – warmer weather.
2. Overgeneralization: Respect the Limitations of Your Data
Your conclusions should be limited to the scope of your data. Avoid generalizing your findings to populations or situations not represented in your dataset. For instance, conclusions drawn from a study of college students may not apply to the general population.
3. Ignoring Outliers: Investigate Unusual Data Points
Outliers are data points that are significantly different from other data points. They can be due to errors in data collection or represent genuine anomalies. Don't automatically discard outliers; investigate them to understand their potential causes and impact on your conclusions.
4. Confirmation Bias: Seek Objective Analysis
Confirmation bias is the tendency to favor information that confirms your pre-existing beliefs. To avoid this, approach your analysis objectively, considering all possible explanations, not just those that support your initial hypotheses.
Communicating Your Conclusions: Clarity and Precision
Once you've drawn your conclusions, communicate them clearly and precisely to your intended audience. Your presentation should include:
-
A clear statement of your findings: Summarize your key conclusions in a concise and easy-to-understand manner.
-
Supporting evidence: Provide evidence from your data analysis to support your conclusions. Include relevant tables, graphs, and statistical results.
-
Limitations of the study: Acknowledge any limitations of your data or analysis that might affect the validity of your conclusions.
-
Implications of your findings: Discuss the practical implications of your findings and their potential impact.
-
Suggestions for future research: Suggest areas for future research based on your findings and the limitations of your study.
Example: Analyzing Sales Data
Let's say you have a table showing monthly sales figures for different product categories over a year. You want to determine which product category performed best and identify any seasonal trends.
You would start by calculating descriptive statistics for each product category (mean, median, standard deviation). You might then create line graphs to visualize the sales trends over time for each category. This visual representation would help you identify peak sales periods and any seasonal variations. By comparing the mean sales figures and observing the trends, you could conclude which product category performed best overall and during specific months.
Remember to consider potential confounding factors, such as advertising campaigns or changes in pricing, that might influence your results. Your final conclusions should be clearly stated, supported by the data analysis, and acknowledge any limitations of the study.
Conclusion: The Power of Data-Driven Decisions
Drawing meaningful conclusions from data is a crucial skill that empowers informed decision-making across various fields. By carefully considering the context, employing appropriate analytical techniques, and communicating findings effectively, you can unlock the valuable insights hidden within your data, driving innovation and progress. Remember to always approach data analysis with a critical and cautious mindset, ensuring your conclusions are well-supported, objective, and contribute meaningfully to your overall goals.
Latest Posts
Related Post
Thank you for visiting our website which covers about What Conclusion Do The Data In The Table Support . We hope the information provided has been useful to you. Feel free to contact us if you have any questions or need further assistance. See you next time and don't miss to bookmark.