What Is The Mean Of The Normal Distribution Shown Below
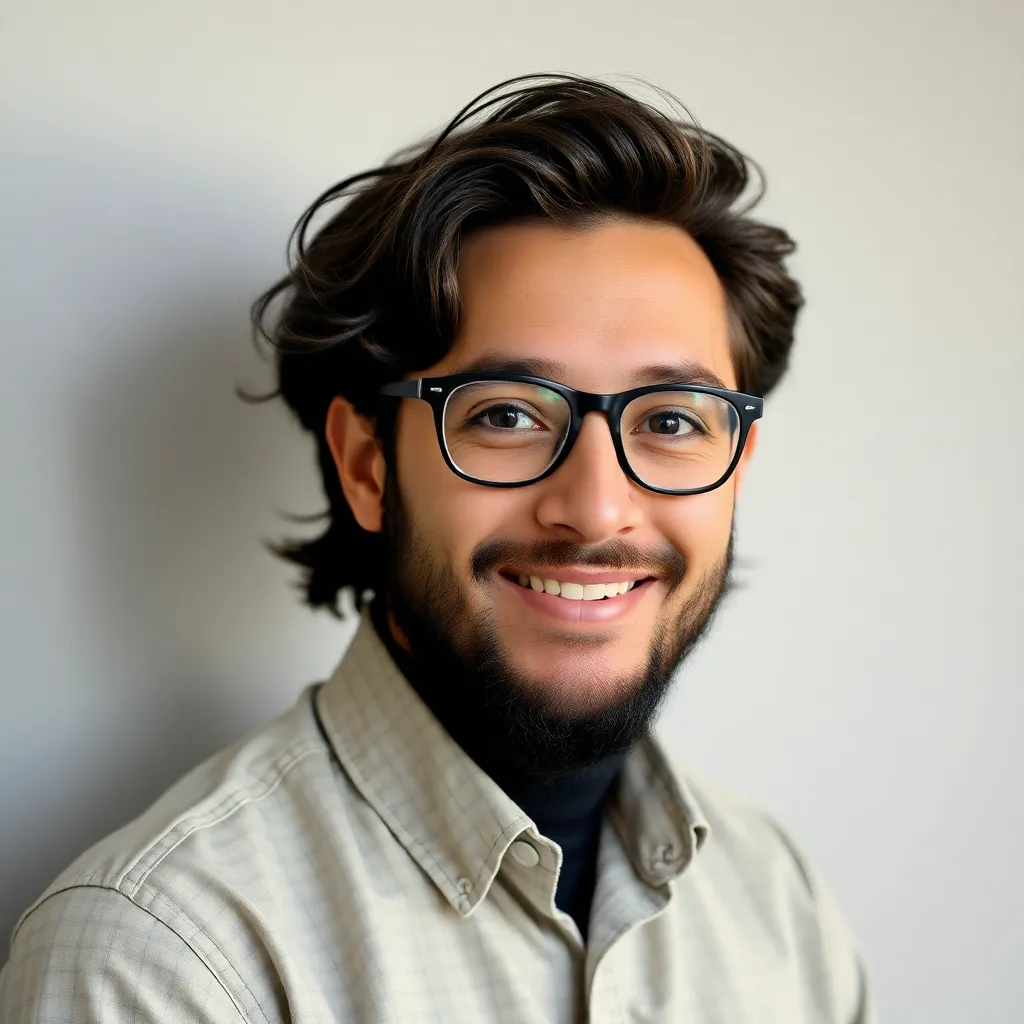
Breaking News Today
Mar 30, 2025 · 5 min read
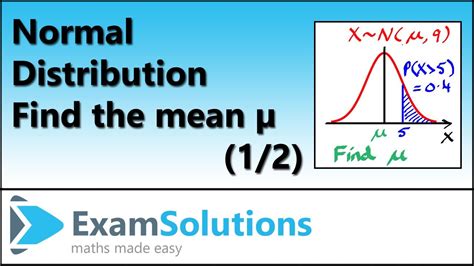
Table of Contents
Decoding the Normal Distribution: Understanding the Bell Curve
The normal distribution, often visualized as the bell curve, is a fundamental concept in statistics and probability. Understanding its meaning and implications is crucial across numerous fields, from finance and medicine to engineering and social sciences. This comprehensive guide will delve deep into the intricacies of the normal distribution, exploring its characteristics, applications, and significance.
What is a Normal Distribution?
A normal distribution, also known as Gaussian distribution, is a probability distribution that is symmetrical around its mean, which is also its median and mode. This means that the data is evenly distributed around the central value, with most observations clustering around the mean and fewer observations occurring further away. The curve's shape resembles a bell, hence the name "bell curve."
Key Characteristics of the Normal Distribution:
- Symmetry: The distribution is perfectly symmetrical around the mean. The left and right halves are mirror images of each other.
- Mean, Median, and Mode: These three measures of central tendency are all equal in a normal distribution.
- Empirical Rule (68-95-99.7 Rule): This rule states that approximately 68% of the data falls within one standard deviation of the mean, 95% within two standard deviations, and 99.7% within three standard deviations. This characteristic is incredibly useful for understanding the spread of data.
- Standard Deviation: The standard deviation measures the spread or dispersion of the data around the mean. A larger standard deviation indicates a wider spread, while a smaller standard deviation indicates a narrower spread.
- Infinite Range: The normal distribution extends infinitely in both directions along the x-axis, although the probability density rapidly approaches zero as you move away from the mean.
Mathematical Representation:
The normal distribution is defined by its mean (μ) and standard deviation (σ). Its probability density function (PDF) is given by:
f(x) = (1/√(2πσ²)) * e^(-(x-μ)²/(2σ²))
While this formula may seem daunting, the key takeaway is that it precisely describes the probability of observing a value within a given range. The parameters μ and σ completely define the shape and location of the curve.
Why is the Normal Distribution Important?
The normal distribution's importance stems from its frequent occurrence in natural phenomena and its valuable properties in statistical analysis:
- Central Limit Theorem: This theorem states that the average of many independent and identically distributed random variables will approximate a normal distribution, regardless of the original distribution's shape. This is crucial because it allows us to use normal distribution properties to analyze data even when the underlying data isn't normally distributed.
- Statistical Inference: Many statistical tests and procedures assume that the data is normally distributed or can be transformed to be approximately normal. This assumption allows for reliable estimations and hypothesis testing.
- Modeling Real-world Phenomena: Many natural phenomena, such as height, weight, blood pressure, and IQ scores, follow an approximately normal distribution.
- Process Control: In industrial settings, the normal distribution is used for quality control and process monitoring. Understanding the variability of a process helps in identifying and correcting deviations from the desired outcome.
- Financial Modeling: In finance, the normal distribution is used to model asset returns, although this assumption has been debated extensively in recent years due to the occurrence of "fat tails" (extreme events) that are not well-captured by the normal distribution.
Applications of the Normal Distribution:
The normal distribution finds applications in a vast range of fields:
- Healthcare: Analyzing patient data, such as blood pressure or cholesterol levels, to identify outliers or diagnose conditions. Understanding the distribution of these variables helps in establishing normal ranges and interpreting test results.
- Education: Analyzing student test scores to assess performance and identify areas for improvement. The normal distribution can help in understanding the distribution of scores and establishing grading curves.
- Engineering: Quality control and process improvement in manufacturing processes. Ensuring products meet specific standards requires understanding the distribution of product characteristics.
- Meteorology: Modeling weather patterns, such as temperature and rainfall. Understanding the distribution of these variables helps in forecasting and planning.
- Social Sciences: Analyzing survey data, such as opinions or attitudes. The normal distribution can help in understanding the distribution of responses and identifying trends.
Limitations of the Normal Distribution:
While incredibly useful, the normal distribution has some limitations:
- Real-world data is rarely perfectly normal: Many real-world datasets only approximate a normal distribution. Deviations from normality can significantly impact the results of statistical tests.
- Sensitivity to outliers: Extreme values (outliers) can significantly affect the mean and standard deviation of a normal distribution, leading to skewed results.
- Assumption of independence: The central limit theorem relies on the assumption of independence between data points. If data points are correlated, the normal approximation may not hold.
- Inability to capture extreme events: The normal distribution's "thin tails" (low probability of extreme values) make it unsuitable for modeling phenomena with frequent extreme events, such as financial market crashes or natural disasters.
Beyond the Basic Normal Distribution:
While the standard normal distribution (mean = 0, standard deviation = 1) is commonly used, variations exist:
- Standardization (z-scores): Any normally distributed variable can be transformed into a standard normal variable by standardizing it, which involves subtracting the mean and dividing by the standard deviation. This allows comparison across different datasets.
- Other Distributions Derived from the Normal: Several other distributions, such as the chi-squared, t-distribution, and F-distribution, are closely related to the normal distribution and are crucial in statistical inference.
Testing for Normality:
Several statistical tests can assess whether a dataset follows a normal distribution. These include:
- Histograms and Q-Q plots: Visual inspection of the data's distribution using histograms and quantile-quantile (Q-Q) plots can provide an initial assessment of normality.
- Shapiro-Wilk test: A formal statistical test for normality.
- Kolmogorov-Smirnov test: Another formal statistical test for normality, often used as an alternative to the Shapiro-Wilk test.
Conclusion:
The normal distribution is a cornerstone of statistical analysis and probability theory. Its symmetrical bell-shaped curve, characterized by its mean and standard deviation, provides a powerful tool for understanding and modeling various phenomena. While not perfectly applicable to all real-world datasets, its importance stems from its frequent occurrence, the central limit theorem, and its role in numerous statistical procedures. Understanding its properties, limitations, and applications is essential for anyone working with data analysis, statistical modeling, or any field that involves understanding variability and probability. Remember that careful consideration of data characteristics and the appropriate choice of statistical methods are paramount for valid conclusions.
Latest Posts
Latest Posts
-
How Should Data In Medical Billing Programs Be Entered
Apr 01, 2025
-
A Benefit Of A Model Eeo Program Is
Apr 01, 2025
-
In The References To An Old Opera Tune
Apr 01, 2025
-
Is It Illegal To Pass Another Vehicle At An Intersection
Apr 01, 2025
-
How Do Healthy Family Relationships Affect Members Of A Community
Apr 01, 2025
Related Post
Thank you for visiting our website which covers about What Is The Mean Of The Normal Distribution Shown Below . We hope the information provided has been useful to you. Feel free to contact us if you have any questions or need further assistance. See you next time and don't miss to bookmark.