What Is Your Prediction For This Experiment
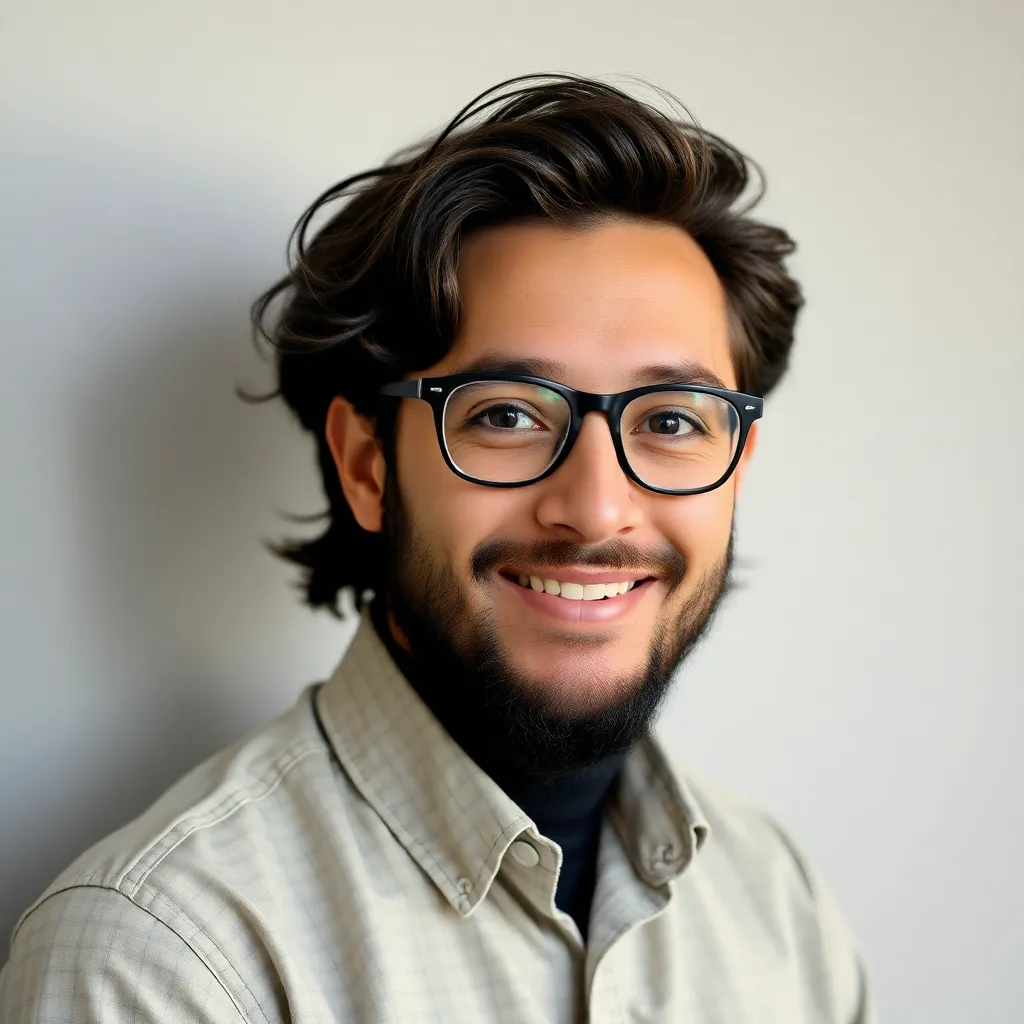
Breaking News Today
May 11, 2025 · 5 min read
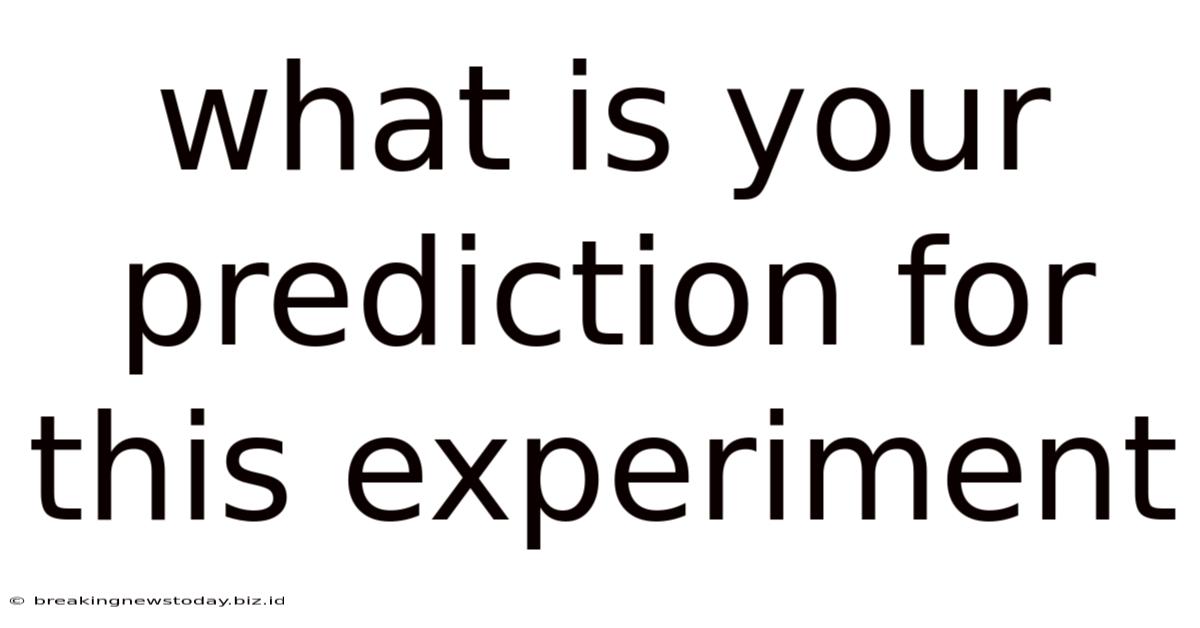
Table of Contents
Predicting the Future: An Analysis of Experimental Outcomes and Forecasting Techniques
Predicting the outcome of an experiment, any experiment, is a complex task fraught with uncertainty. While a well-designed experiment aims to minimize randomness and isolate specific variables, inherent unpredictability remains. This article delves into the various factors influencing experimental predictions, exploring different forecasting techniques and the limitations inherent in projecting future results. We’ll explore both quantitative and qualitative approaches, ultimately highlighting the crucial role of understanding the underlying mechanisms and acknowledging the inherent uncertainties involved.
Understanding the Experiment's Context: The Foundation of Prediction
Before we delve into specific prediction methods, it's critical to fully understand the nature of the experiment itself. This involves a thorough examination of several key aspects:
1. Defining the Objectives and Hypotheses: Clarity is Key
The experiment's objectives and hypotheses form the bedrock of any predictive analysis. A clearly defined objective provides a target for our predictions. Similarly, well-formulated hypotheses suggest potential outcomes and pathways to explore. Vague objectives and poorly defined hypotheses lead to imprecise, unreliable predictions. For example, an experiment aimed at determining the effect of a new fertilizer on crop yield needs clearly defined metrics (e.g., yield in kilograms per hectare) and a specific hypothesis (e.g., the new fertilizer will increase yield by at least 15%).
2. Identifying Variables and Their Interactions: Unraveling Complexity
The identification and understanding of independent, dependent, and confounding variables are crucial. Independent variables are those manipulated by the experimenter, while dependent variables are the measured outcomes. Confounding variables are extraneous factors that can influence the dependent variable, potentially distorting the results. A detailed understanding of these variables and their potential interactions is paramount for accurate prediction. Consider an experiment studying the effect of a new drug on blood pressure. Confounding variables could include age, diet, exercise levels, and pre-existing health conditions. Failing to account for these can severely impact the predictive accuracy.
3. Review of Existing Literature and Similar Experiments: Learning from the Past
A comprehensive literature review is essential. Examining previous research on similar experiments, methodologies, and related phenomena provides valuable insights and context. This review can identify potential pitfalls, suggest appropriate analytical techniques, and provide a baseline for comparison. For example, if an experiment involves a novel material, reviewing studies on materials with similar properties can inform predictions about its behavior under specific conditions.
4. Assessing the Experimental Design: Robustness and Reliability
The experimental design itself profoundly impacts predictive accuracy. A well-designed experiment employs rigorous controls, minimizes bias, and maximizes statistical power. Factors such as sample size, randomization techniques, and control groups all influence the reliability of the results and, consequently, the confidence in any predictions. A robust experimental design is essential for generating reliable predictions, and weaknesses in the design can lead to inaccurate projections.
Prediction Methods: A Multifaceted Approach
Once the experimental context is thoroughly understood, various prediction methods can be employed. These methods range from simple extrapolations to sophisticated statistical modeling techniques:
1. Extrapolation and Interpolation: Simple yet Powerful
For experiments involving trends or patterns, simple extrapolation or interpolation techniques can be effective. Extrapolation involves projecting the trend beyond the observed data points, while interpolation involves estimating values within the range of observed data. This approach works best when a clear, consistent trend is evident. However, it's essential to acknowledge the inherent uncertainty when extrapolating far beyond the observed data.
2. Statistical Modeling: Leveraging Data and Theory
Statistical modeling techniques, such as regression analysis, ANOVA, and time series analysis, provide more sophisticated methods for prediction. These techniques utilize statistical relationships between variables to predict the dependent variable's value based on the values of the independent variables. Regression analysis, for example, allows us to model the relationship between variables and predict the outcome based on the model's parameters. The choice of statistical model depends on the nature of the data and the research questions.
3. Machine Learning: Harnessing the Power of Algorithms
Machine learning algorithms, particularly those based on artificial neural networks and support vector machines, offer powerful tools for prediction. These algorithms can identify complex patterns and relationships in data that may be missed by traditional statistical models. They are particularly useful when dealing with large datasets and high dimensionality. However, the "black box" nature of some machine learning algorithms can make it difficult to interpret the underlying mechanisms driving the predictions.
4. Simulation and Modeling: Exploring Hypothetical Scenarios
For complex experiments involving multiple interacting factors, simulation and modeling techniques can be invaluable. These techniques involve creating a computational model of the experimental system and simulating different scenarios to predict the outcomes. This approach allows us to explore the impact of various factors and test different hypotheses without the need for extensive physical experimentation. Agent-based modeling, for instance, can simulate the interactions between individual agents to predict emergent system-level behavior.
Assessing Prediction Uncertainty: Acknowledging Limitations
Regardless of the chosen prediction method, it is crucial to acknowledge the inherent uncertainties involved. No prediction is guaranteed to be perfectly accurate. The uncertainty arises from several sources:
- Random Variation: Inherent randomness in the experimental process leads to variations in the results.
- Measurement Error: Imperfect measurement instruments and techniques introduce errors into the data.
- Model Limitations: All models are simplifications of reality, and their limitations affect the accuracy of predictions.
- Unforeseen Events: Unanticipated factors or external events can impact the experimental outcome.
Quantifying this uncertainty is critical. This can be done through confidence intervals, prediction intervals, or probabilistic forecasting techniques. Providing a range of possible outcomes, along with their associated probabilities, gives a more realistic and informative prediction than a single point estimate.
Conclusion: A Holistic Approach to Prediction
Predicting the outcome of an experiment requires a multifaceted approach. It begins with a thorough understanding of the experimental context, including its objectives, hypotheses, variables, and design. Various prediction methods, from simple extrapolation to sophisticated machine learning algorithms, can then be employed. However, it is crucial to acknowledge and quantify the inherent uncertainties involved. By integrating quantitative and qualitative methods, critically evaluating assumptions, and acknowledging limitations, we can generate more robust and reliable predictions. The ultimate goal is not to predict with perfect accuracy, but rather to generate informed estimates that guide future research, decision-making, and resource allocation. A holistic, iterative approach, incorporating feedback from experimental results and ongoing refinement of prediction models, will ultimately lead to improved predictive capabilities.
Latest Posts
Latest Posts
-
Mrs Wang Wants To Know Generally How The Benefits
May 11, 2025
-
The Benefits In Medical Expense Insurance Are
May 11, 2025
-
What Is The Unit Of Measurement For Voltage
May 11, 2025
-
Osha Standards Come From All Of The Following Sources Except
May 11, 2025
-
Match The Assessment Tools With Their Designed Outcomes
May 11, 2025
Related Post
Thank you for visiting our website which covers about What Is Your Prediction For This Experiment . We hope the information provided has been useful to you. Feel free to contact us if you have any questions or need further assistance. See you next time and don't miss to bookmark.