Which Event Most Likely Occurs At Point K
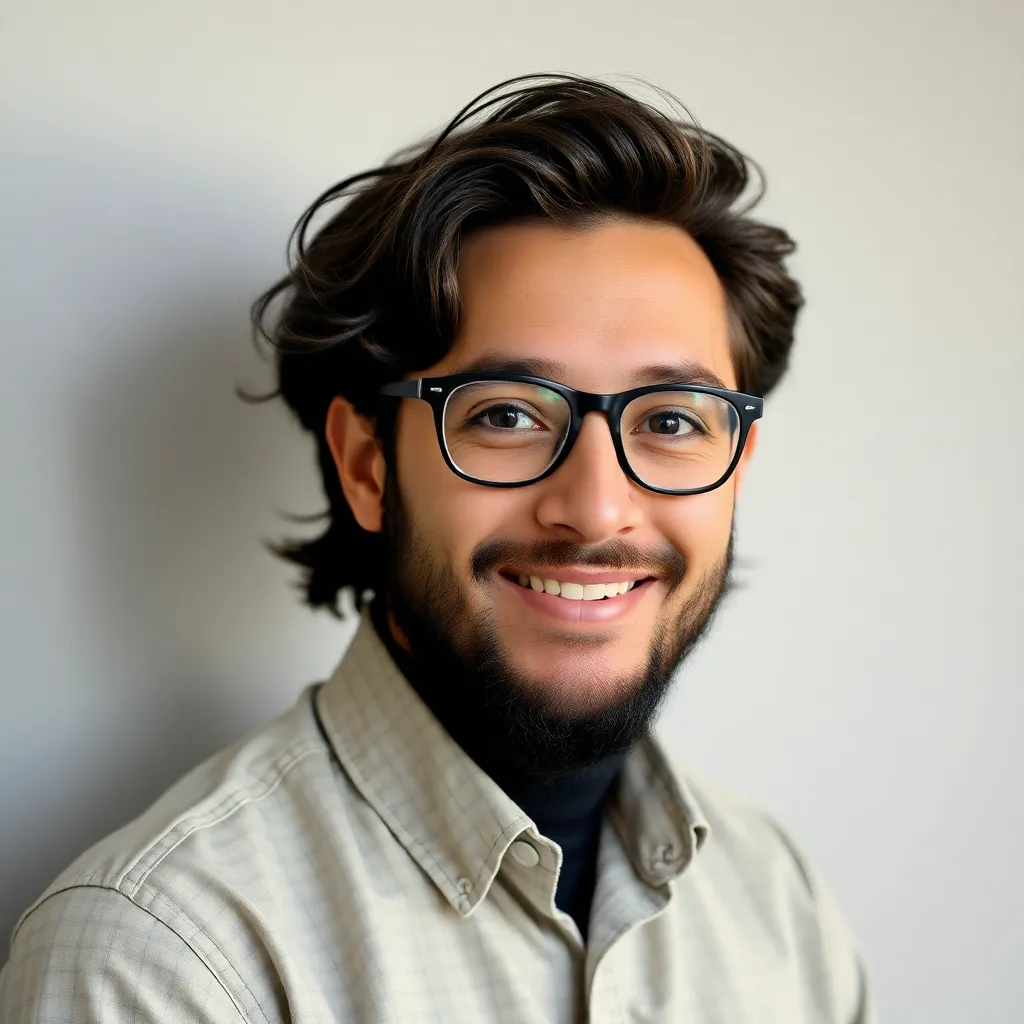
Breaking News Today
May 09, 2025 · 6 min read
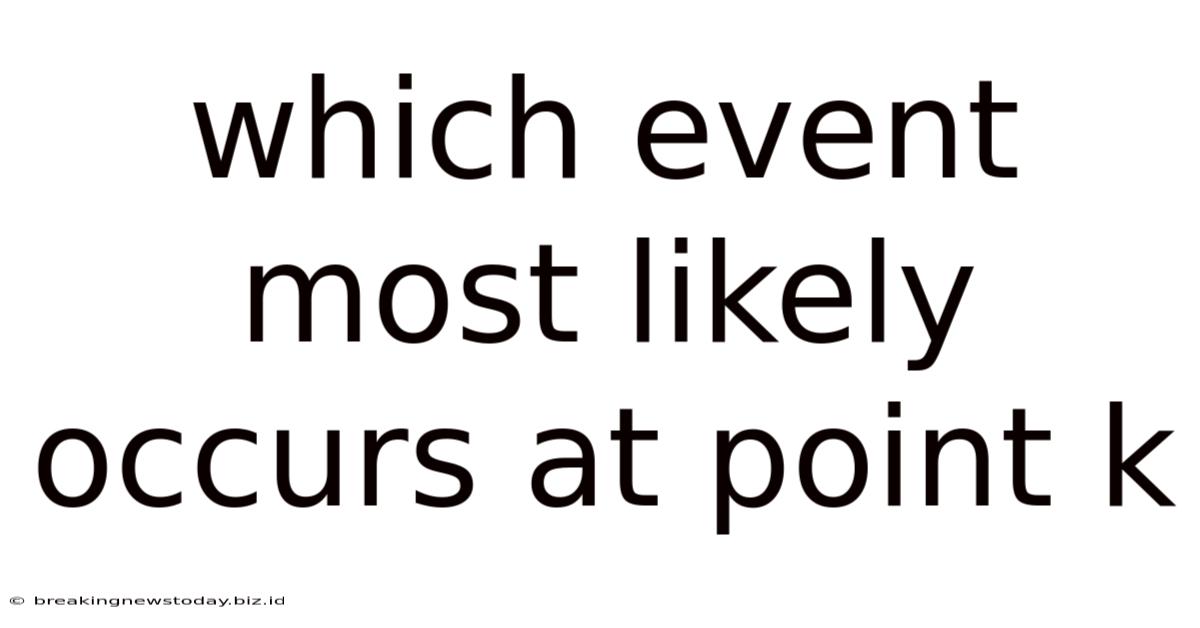
Table of Contents
Which Event Most Likely Occurs at Point K? A Comprehensive Analysis
Determining the most likely event at a specific point, denoted as "Point K," necessitates a deep understanding of the context surrounding this point. Without specific details about the system or process under consideration, providing a definitive answer is impossible. However, we can explore several scenarios and methodologies for analyzing the probability of different events at Point K. This article will delve into various approaches, providing a framework for tackling such problems, regardless of the specific application.
Understanding the Context: The Crucial First Step
Before even attempting to predict the most likely event at Point K, we must thoroughly understand the context. What system are we examining? Is it a physical process, a biological system, a financial model, or something else entirely? Key questions to ask include:
-
What is the nature of Point K? Is it a point in time, a geographical location, a stage in a process, or something else? Understanding the nature of Point K is crucial for defining the relevant events.
-
What are the potential events that could occur at Point K? This requires a comprehensive list of all possibilities, however improbable. This list serves as the basis for our probability analysis.
-
What data or information is available about Point K and its surrounding environment? Data is the lifeblood of any probability analysis. The more data we have, the more accurate our predictions will be. This may include historical data, experimental results, simulations, or expert opinions.
-
What are the underlying assumptions and limitations? All analyses have limitations. It's crucial to explicitly state these limitations to avoid misinterpretations and ensure transparency.
Methods for Analyzing Event Probability at Point K
Several methods can be employed to analyze the probability of different events at Point K, each with its strengths and weaknesses:
1. Frequency Analysis (Empirical Probability):
If historical data is available, frequency analysis is a straightforward approach. This involves counting the number of times each event has occurred at Point K in the past and calculating the relative frequency of each event. The event with the highest relative frequency is considered the most likely.
-
Example: If Point K represents a specific intersection in a city, and historical traffic data shows that accidents occur most frequently on Tuesdays between 5 pm and 6 pm, then an accident is the most likely event at Point K during that time on Tuesdays.
-
Limitations: This approach assumes that the future will resemble the past. This assumption may not always hold true, especially if the system or context is changing.
2. Bayesian Analysis:
Bayesian analysis is a powerful tool for updating probabilities based on new evidence. It starts with a prior probability (initial belief about the likelihood of each event) and then updates this probability based on new data using Bayes' theorem. This is particularly useful when dealing with uncertainty and limited data.
-
Example: Suppose Point K represents a machine in a factory. We might have a prior probability based on the machine's historical failure rate. If we observe some anomaly in the machine's performance at Point K, we can update the probability of failure using Bayesian analysis.
-
Limitations: The accuracy of Bayesian analysis depends on the accuracy of the prior probability and the quality of the new data.
3. Markov Chains:
If the events at Point K are sequential and dependent on previous events, a Markov chain model can be used. This model describes the probability of transitioning from one state (event) to another. By analyzing the transition probabilities, we can predict the most likely event at Point K given the current state.
-
Example: If Point K represents a step in a manufacturing process, and the outcome of each step depends on the outcome of the previous step, a Markov chain model can predict the most likely outcome at Point K given the current state of the process.
-
Limitations: Markov chains assume that the future state depends only on the present state and not on the past. This assumption may not always hold true in complex systems.
4. Simulation:
If a mathematical model of the system is available, simulation can be used to generate many possible scenarios and estimate the probability of each event at Point K. This approach is particularly useful when analytical methods are intractable.
-
Example: If Point K represents a point in a complex chemical reaction, simulation can be used to generate many possible reaction pathways and estimate the probability of each outcome at Point K.
-
Limitations: The accuracy of the simulation depends on the accuracy of the underlying model. Furthermore, simulations can be computationally expensive, especially for complex systems.
5. Expert Elicitation:
In situations where data is scarce or unavailable, expert opinions can be used to estimate the probability of different events at Point K. This involves gathering opinions from experts in the relevant field and combining them using appropriate aggregation techniques.
-
Example: If Point K represents a geological event, such as an earthquake, expert opinions from seismologists can be used to estimate the probability of different magnitudes of earthquakes at Point K.
-
Limitations: Expert opinions can be subjective and may vary significantly among experts.
Choosing the Right Method:
The best method for determining the most likely event at Point K depends on several factors, including:
-
Availability of data: If abundant historical data is available, frequency analysis might be suitable. If data is scarce, Bayesian analysis or expert elicitation might be more appropriate.
-
Complexity of the system: For simple systems, frequency analysis or a simple probability model might suffice. For complex systems, simulation or Markov chains might be necessary.
-
Time constraints: Some methods, such as simulation, can be computationally expensive and time-consuming.
-
Resources available: The choice of method may be constrained by the resources available, including computational power, expertise, and data.
Conclusion:
Identifying the most probable event at Point K requires a systematic approach that considers the context, available data, and the strengths and weaknesses of various analytical methods. This article has provided a framework for tackling such problems, highlighting the importance of careful planning, thorough data analysis, and the selection of an appropriate analytical methodology. Remember, the key to success lies in a deep understanding of the problem and a rigorous application of appropriate analytical techniques. By combining these elements, you can increase your confidence in predicting the most likely event at Point K, regardless of the specific system or process under investigation. This framework should provide a strong foundation for future analyses of similar problems. The process of identifying the most probable event is often iterative, requiring refinement and adjustments based on new information and insights gained during the analysis. Continuous monitoring and evaluation are crucial for ensuring the accuracy and relevance of the conclusions drawn.
Latest Posts
Latest Posts
-
Organisms That Feed On Dead Organisms For Nutrients Are Called
May 09, 2025
-
When You Merge Onto A Freeway You Should
May 09, 2025
-
Which Of The Following Is Associated With Passive Immunity
May 09, 2025
-
Label The Parts Of A Motor Neuron
May 09, 2025
-
In A Typical Rear End Collision The Victims
May 09, 2025
Related Post
Thank you for visiting our website which covers about Which Event Most Likely Occurs At Point K . We hope the information provided has been useful to you. Feel free to contact us if you have any questions or need further assistance. See you next time and don't miss to bookmark.