Which Of The Following Are Examples Of Inferential Statistics
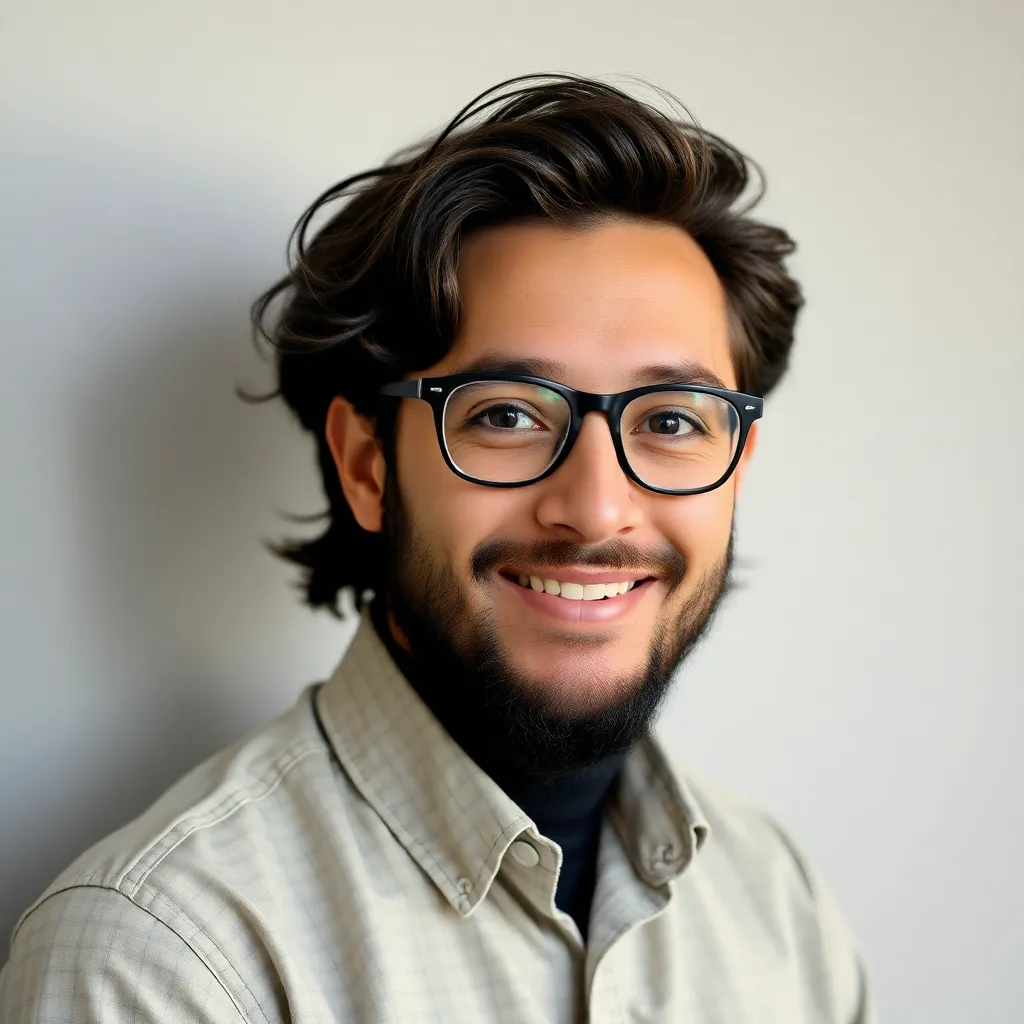
Breaking News Today
May 11, 2025 · 7 min read
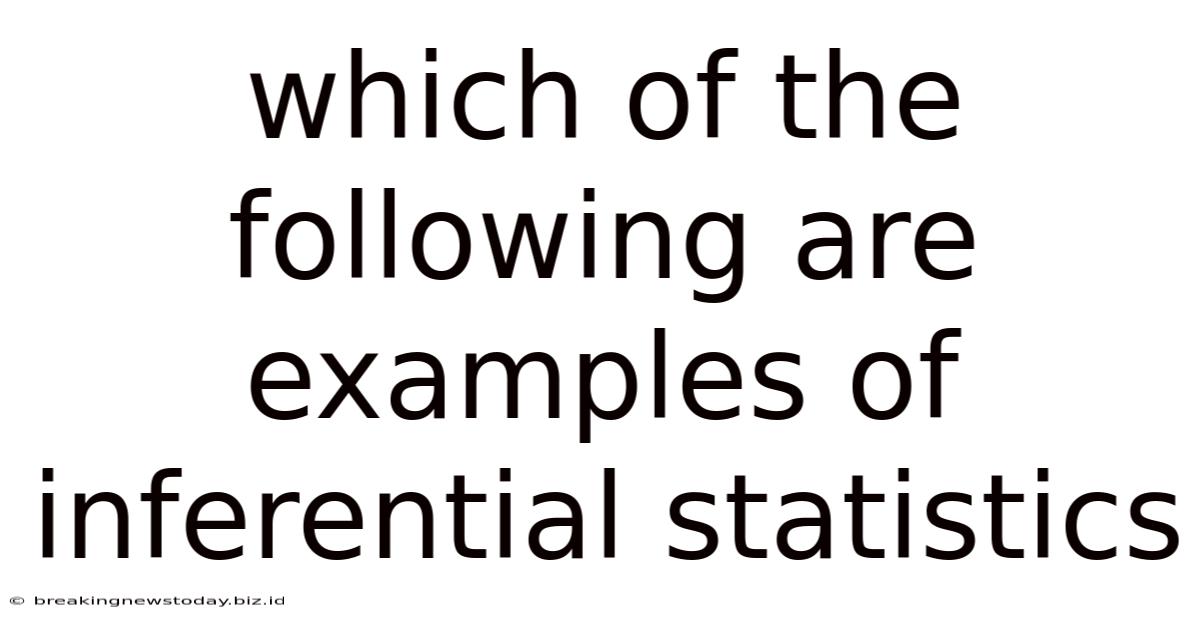
Table of Contents
Which of the Following are Examples of Inferential Statistics?
Inferential statistics plays a crucial role in drawing meaningful conclusions from data. Unlike descriptive statistics, which summarizes data, inferential statistics uses sample data to make inferences about a larger population. This article delves into the core concepts of inferential statistics, exploring various examples to solidify your understanding. We'll clarify what constitutes inferential statistics and differentiate it from descriptive statistics. By the end, you'll be able to confidently identify examples of inferential statistics and understand their application in diverse fields.
Understanding Inferential Statistics: A Foundation
Before diving into examples, let's establish a firm understanding of what constitutes inferential statistics. Inferential statistics employs probability theory to analyze sample data and draw conclusions about the population from which the sample was taken. This process involves making estimations, testing hypotheses, and determining the significance of observed patterns. The goal is to generalize findings from a smaller group (the sample) to a larger group (the population).
Key Characteristics of Inferential Statistics:
- Generalization: The primary purpose is to generalize findings from a sample to a population.
- Probability: Relies heavily on probability theory to quantify uncertainty and assess the reliability of conclusions.
- Hypothesis Testing: Frequently involves testing hypotheses about population parameters.
- Estimation: Uses sample statistics to estimate population parameters, such as the mean or standard deviation.
- Confidence Intervals: Provides a range of values within which a population parameter is likely to fall, along with a specified level of confidence.
- p-values: Quantifies the probability of observing the obtained results (or more extreme results) if the null hypothesis is true.
Differentiating Inferential and Descriptive Statistics
It's crucial to distinguish between inferential and descriptive statistics. While both are important aspects of data analysis, they serve different purposes:
Descriptive Statistics: Summarizes and describes the main features of a dataset. It focuses on describing what the data shows without making inferences about a larger population. Examples include:
- Mean: The average value of a dataset.
- Median: The middle value in a sorted dataset.
- Mode: The most frequent value in a dataset.
- Standard Deviation: Measures the spread or dispersion of data around the mean.
- Frequency Distribution: Shows how often each value or range of values occurs.
Inferential Statistics: Goes beyond describing the data to make inferences and predictions about a larger population. It uses sample data to draw conclusions about the population from which the sample originated. Examples include:
- t-tests: Compare the means of two groups to determine if there's a statistically significant difference.
- ANOVA (Analysis of Variance): Compares the means of three or more groups.
- Regression Analysis: Examines the relationship between a dependent variable and one or more independent variables.
- Chi-Square Test: Analyzes the association between categorical variables.
Examples of Inferential Statistics in Action
Let's explore diverse scenarios that showcase the application of inferential statistics:
1. A Pharmaceutical Company Testing a New Drug
Imagine a pharmaceutical company developing a new drug to lower blood pressure. They conduct a clinical trial involving 100 participants, randomly assigning 50 to the treatment group (receiving the new drug) and 50 to the control group (receiving a placebo). After a set period, they measure the blood pressure of all participants. To determine if the new drug effectively lowers blood pressure, they would use t-tests (an inferential statistical method) to compare the mean blood pressure reduction in the treatment group versus the control group. The results will indicate whether the observed difference is statistically significant, suggesting the drug's effectiveness. The p-value associated with the t-test helps to determine if the observed difference is likely due to chance or a real effect of the drug.
2. Analyzing Voter Preferences Before an Election
A political polling agency wants to predict the outcome of an upcoming election. They conduct a survey of 1000 randomly selected voters, asking them about their preferred candidate. Using proportions and confidence intervals, they can estimate the percentage of the entire electorate who support each candidate. This prediction, although based on a sample, allows them to make inferences about the likely outcome of the election in the entire population of voters. The margin of error associated with the confidence interval indicates the uncertainty inherent in the estimation process.
3. Examining the Relationship Between Education and Income
Researchers are interested in understanding the relationship between years of education and annual income. They collect data on a sample of individuals, including their years of education and their annual income. Using regression analysis, they can model the relationship between these two variables, determining if there is a statistically significant association and the strength of that association. The regression model allows them to predict income based on years of education, making an inference about the larger population based on the sample data.
4. Assessing Customer Satisfaction with a Product
A company wants to gauge customer satisfaction with its new product. They send out surveys to a random sample of their customers, asking them to rate their satisfaction on a scale of 1 to 5. They can then use descriptive statistics to summarize the responses (e.g., calculating the average satisfaction score). However, to make inferences about the overall customer satisfaction level for the entire population of customers, they would utilize inferential statistics, perhaps calculating a confidence interval for the mean satisfaction score. This would give them a range of values within which the true population mean satisfaction score likely falls.
5. Determining the Effectiveness of a New Teaching Method
Educators want to determine if a new teaching method improves student performance. They randomly assign students to two groups: one using the new method and the other using the traditional method. After the intervention period, they compare the test scores of the two groups. ANOVA (Analysis of Variance), an inferential statistical technique, would be used to determine if there's a statistically significant difference in test scores between the two groups. The results would provide evidence for or against the new teaching method’s effectiveness.
6. Analyzing the Association Between Smoking and Lung Cancer
Researchers investigate the association between smoking and lung cancer. They collect data on a sample of individuals, noting whether they smoke and whether they have lung cancer. The Chi-Square test, an inferential statistical method, is used to analyze the association between these two categorical variables. This test determines if there is a statistically significant association between smoking and the risk of developing lung cancer. The results from this sample are then generalized to make inferences about the population.
Beyond the Examples: Key Considerations
While the examples above illustrate the versatility of inferential statistics, it's vital to remember certain crucial considerations:
- Sample Representativeness: The accuracy of inferences depends heavily on the representativeness of the sample. A biased or non-random sample can lead to misleading conclusions.
- Sample Size: Larger sample sizes generally lead to more reliable inferences.
- Statistical Significance vs. Practical Significance: A statistically significant result doesn't automatically imply practical significance. A small difference might be statistically significant but inconsequential in real-world terms.
- Assumptions: Many inferential statistical methods rely on certain assumptions about the data (e.g., normality, independence). Violation of these assumptions can affect the validity of the results.
Conclusion
Inferential statistics is a powerful toolkit for drawing meaningful conclusions from data, enabling us to make predictions and generalizations about populations based on sample data. Understanding its core principles and the various techniques it encompasses is crucial for informed decision-making across various disciplines. By mastering the concepts presented here, you can confidently identify examples of inferential statistics and appreciate their indispensable role in scientific research, business analysis, and many other fields. Remember that the accuracy and reliability of inferential statistics depend on careful experimental design, appropriate data collection methods, and a robust understanding of the chosen statistical techniques. Always critically assess the limitations and assumptions underlying any inferential statistical analysis.
Latest Posts
Latest Posts
-
Label The Digestive Abdominal Contents Using The Hints If Provided
May 11, 2025
-
How Does Its Average Distance Compare To That Of Pluto
May 11, 2025
-
My Heart Was A Heavy Rock In My Chest
May 11, 2025
-
Mrs Wang Wants To Know Generally How The Benefits
May 11, 2025
-
The Benefits In Medical Expense Insurance Are
May 11, 2025
Related Post
Thank you for visiting our website which covers about Which Of The Following Are Examples Of Inferential Statistics . We hope the information provided has been useful to you. Feel free to contact us if you have any questions or need further assistance. See you next time and don't miss to bookmark.