Which Of The Following Is False Regarding Control Charts
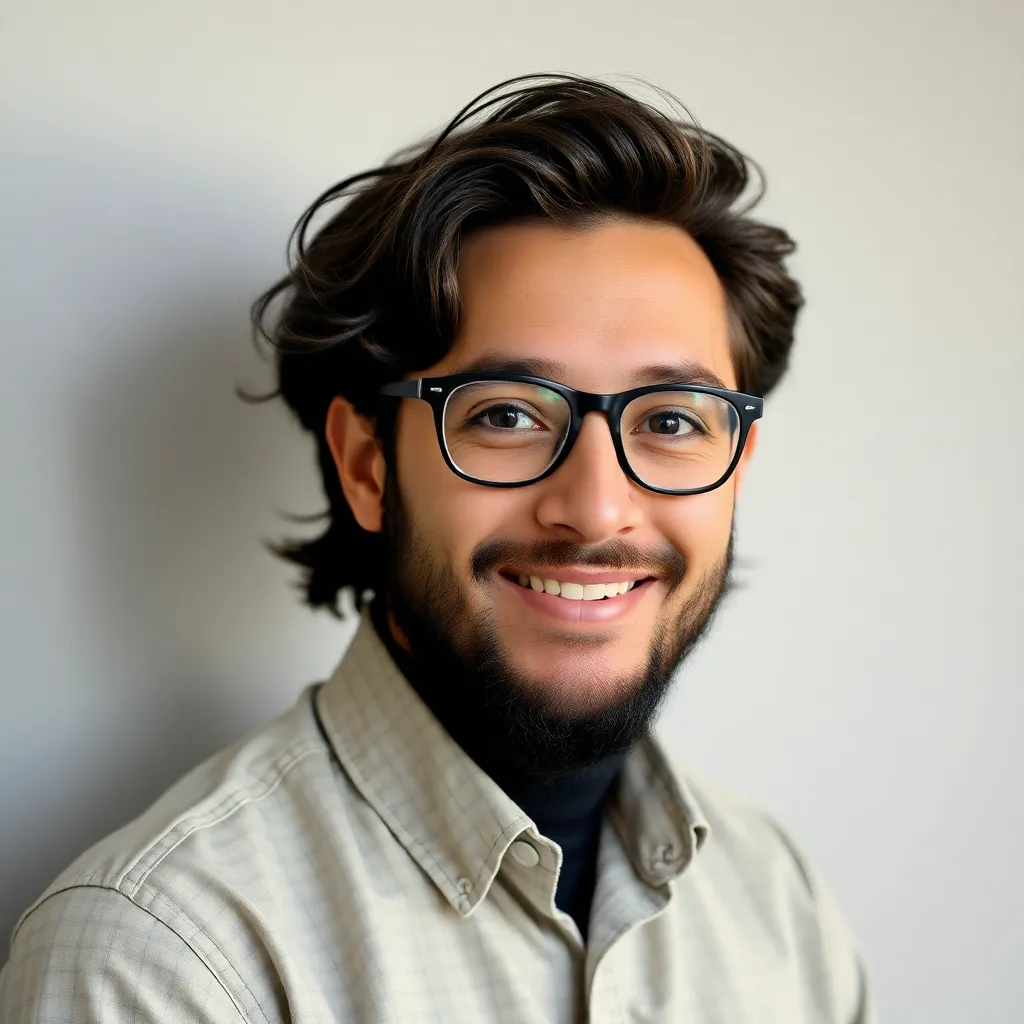
Breaking News Today
May 10, 2025 · 6 min read
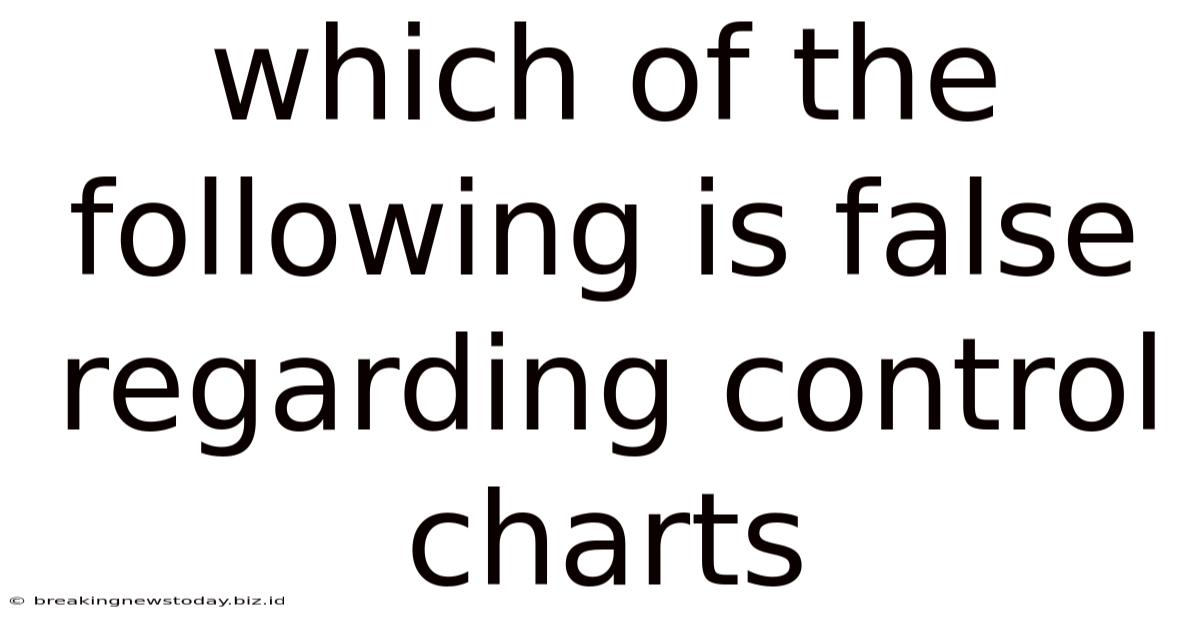
Table of Contents
Which of the Following is False Regarding Control Charts?
Control charts, powerful tools in statistical process control (SPC), are indispensable for monitoring process stability and identifying assignable causes of variation. Understanding their strengths and limitations is crucial for effective implementation. This article delves into common misconceptions surrounding control charts, clarifying which statements regarding them are false and why. We’ll explore the fundamental principles, explore various types, and debunk common myths to solidify your understanding.
Understanding Control Charts: The Basics
Before dissecting false statements, let's establish a solid foundation. Control charts graphically display data collected over time, allowing for the identification of trends and patterns. They consist of:
- Central Line: Represents the average (mean) of the process.
- Upper Control Limit (UCL): The upper boundary beyond which points indicate potential out-of-control conditions.
- Lower Control Limit (LCL): The lower boundary below which points similarly suggest out-of-control conditions.
Data points plotted above the UCL or below the LCL signal potential issues needing investigation. The process is considered "in control" when points fall within these limits and exhibit random variation. Consistent, predictable performance is the hallmark of an in-control process.
Several types of control charts cater to different data types and process characteristics. Common examples include:
- X-bar and R charts: Used for monitoring the average (X-bar) and range (R) of continuous data.
- X-bar and s charts: Similar to X-bar and R charts, but utilize the standard deviation (s) instead of the range. Generally preferred for larger sample sizes (n>10).
- p-charts: Used for monitoring the proportion of nonconforming units in a sample.
- np-charts: Monitor the number of nonconforming units in a sample of constant size.
- c-charts: Used for monitoring the number of defects per unit.
- u-charts: Monitor the number of defects per unit when the sample size varies.
Debunking Common Misconceptions: False Statements about Control Charts
Now, let’s address common misconceptions often encountered regarding control charts. Identifying the false statements allows for a clearer understanding of their proper application and interpretation.
1. FALSE: Control charts only detect special cause variation; they cannot detect common cause variation.
This is a common misunderstanding. While control charts excel at identifying special cause variation (assignable causes, such as machine malfunction or operator error), they also provide crucial information about common cause variation (inherent variability within the process). A process operating with only common cause variation will exhibit points randomly distributed within the control limits. The consistent presence of points within the limits indicates a stable process driven by common cause variation, not necessarily a perfect one. Understanding the level of common cause variation allows for process improvement efforts focused on reducing this inherent variability.
2. FALSE: A process is always improved if points fall within the control limits.
While a process in control (points within limits and showing random variation) is more predictable and stable, this doesn't automatically equate to an "improved" process. A process can be in control but still perform poorly if the process average (central line) is far from the target value. Control charts indicate process stability, not necessarily process capability. Improvements might involve shifting the central line closer to the desired target, reducing common cause variation, or addressing systematic biases.
3. FALSE: Control charts are only useful for manufacturing processes.
This statement is false. Control charts are versatile tools applicable across diverse fields beyond manufacturing. Their application extends to:
- Healthcare: Monitoring infection rates, patient wait times, or medication errors.
- Finance: Tracking investment returns, customer churn, or transaction processing times.
- Service industries: Monitoring customer satisfaction, call center handling times, or order fulfillment speed.
- Software development: Tracking bug rates, code defects, or deployment success rates.
The adaptability of control charts makes them valuable tools for monitoring and improving processes in almost any industry. The key is selecting the appropriate chart type based on the nature of the data.
4. FALSE: Only trained statisticians can effectively use control charts.
While statistical knowledge is helpful for designing and interpreting control charts, their application doesn't require advanced statistical expertise. Many software packages automate the creation and analysis of control charts, making them accessible to a wider audience. However, understanding the underlying principles, limitations, and interpretation of results remains crucial for effective use. While specialized training is beneficial, basic understanding is sufficient for everyday application and interpreting the key outputs (points outside control limits, trends, etc.).
5. FALSE: Once a process is in control, no further monitoring is needed.
Maintaining process control is an ongoing effort, not a one-time achievement. Even a stable process can drift out of control over time due to unforeseen changes or subtle shifts. Continuous monitoring is vital to identify and address potential issues proactively. Regularly updating control charts and analyzing patterns remains necessary for sustained process improvement.
6. FALSE: Control charts guarantee the elimination of all defects.
Control charts help to identify and reduce defects, but they don't offer a guarantee of their complete elimination. The inherent variability within any process means some level of defects will always exist. The goal is to minimize defects to acceptable levels and maintain consistent process performance.
7. FALSE: Control limits should always be set at three standard deviations.
While three-sigma limits (three standard deviations from the mean) are commonly used, they are not universally applicable. The choice of control limits depends on factors like the process's inherent variability, the acceptable risk of false alarms, and the consequences of missing a signal. Narrower control limits can lead to more frequent false alarms, while wider limits may increase the risk of missing significant shifts in the process. The selection of an appropriate limit requires careful consideration of the specific context.
8. FALSE: All points outside the control limits indicate a problem.
Points outside the control limits raise concerns, but they aren't always definitive proof of a problem. Rarely, random variation can produce points outside the limits. A thorough investigation is always necessary to confirm the cause before making any changes to the process. This often involves examining related data, investigating potential assignable causes, and ensuring that the point is not an outlier due to error or unusual circumstances.
9. FALSE: Control charts only deal with individual data points; they cannot handle subgroups of data.
This statement is incorrect. Many control chart designs, especially the X-bar and R charts, specifically leverage data subgroups to provide more insightful information about the process variation over time. Analyzing subgroups allows for a more effective separation of common and special cause variation, providing a clearer picture of the process stability.
10. FALSE: Ignoring non-random patterns within control limits is acceptable.
Even if all data points remain within the control limits, non-random patterns (trends, cycles, stratification) can indicate potential issues. Ignoring these patterns can mask underlying problems that may eventually lead to out-of-control situations. Careful examination of the control chart for these non-random patterns is critical, even if all points remain within the limits. These patterns might be related to tool wear, environmental factors, or subtle shifts in the process that warrant investigation and corrective action.
Conclusion: Effective Control Chart Implementation
Control charts are invaluable tools for process monitoring and improvement. Understanding their strengths, limitations, and the common misconceptions surrounding their use is crucial for effective implementation. By debunking these false statements, we can ensure that control charts are used appropriately, leading to more accurate process assessment and effective continuous improvement initiatives. Remember that the successful application of control charts requires not just the correct technical implementation but also a strong understanding of the process itself, a systematic approach to investigation, and a commitment to ongoing monitoring and improvement.
Latest Posts
Latest Posts
-
A Nurse Is Teaching About Self Administration Of Transdermal Medication
May 10, 2025
-
Which Of The Following Is Not A Stimulus For Breathing
May 10, 2025
-
You Want To Turn Right At The Next Intersection
May 10, 2025
-
Which Of The Following Is True Of High Pressure Areas
May 10, 2025
-
Implicit Biases Can Directly Conflict With Someones Stated Beliefs
May 10, 2025
Related Post
Thank you for visiting our website which covers about Which Of The Following Is False Regarding Control Charts . We hope the information provided has been useful to you. Feel free to contact us if you have any questions or need further assistance. See you next time and don't miss to bookmark.