Which Of The Following Represents A Valid Probability Distribution
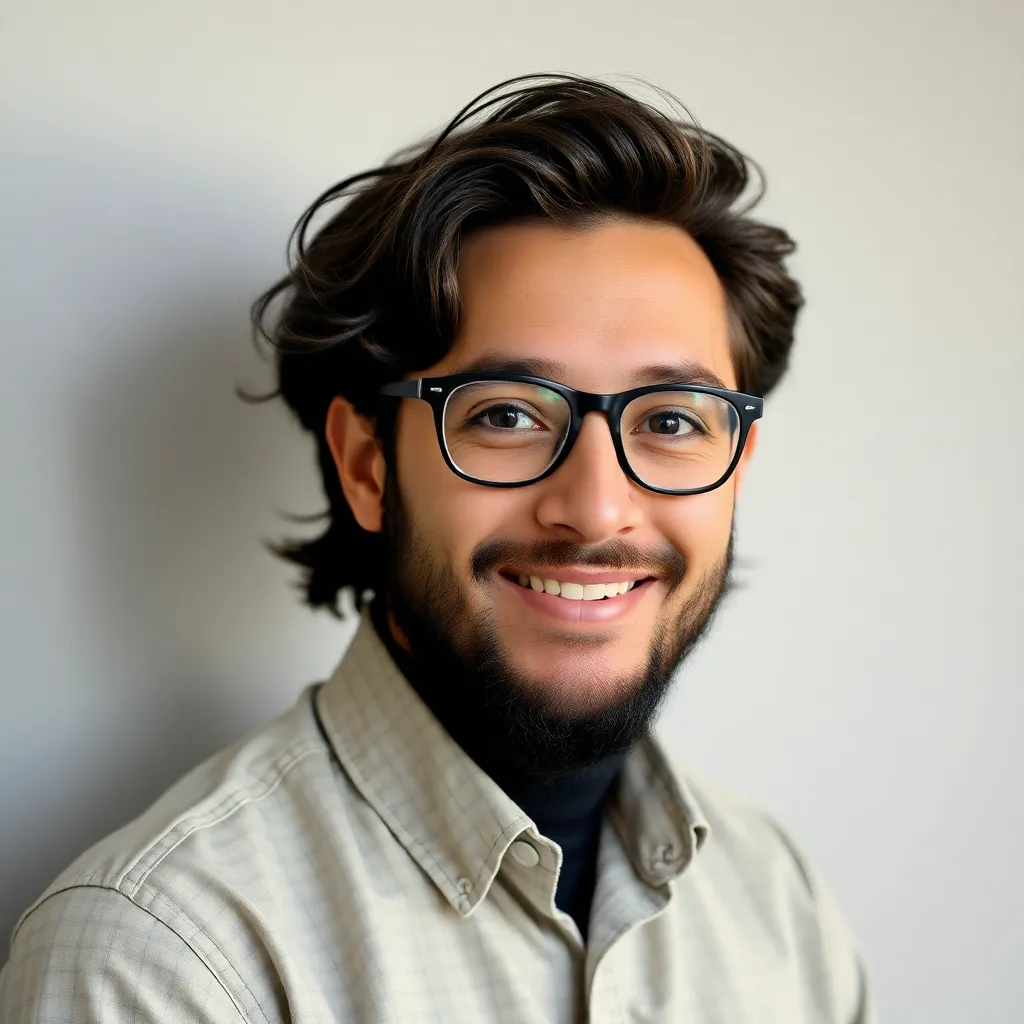
Breaking News Today
Apr 25, 2025 · 6 min read

Table of Contents
Which of the Following Represents a Valid Probability Distribution? A Comprehensive Guide
Understanding probability distributions is fundamental to statistics and numerous applications across various fields, from finance and engineering to medicine and social sciences. A probability distribution describes the likelihood of different outcomes in an experiment. However, not every set of probabilities constitutes a valid probability distribution. This article delves into the criteria that define a valid probability distribution, explores common types, and provides examples to solidify your understanding.
What is a Probability Distribution?
A probability distribution is a mathematical function that provides the probabilities of occurrence of different possible outcomes for an experiment. It's a complete description of the possible outcomes and their associated probabilities. Crucially, these probabilities must adhere to specific rules to form a valid distribution.
Key Characteristics of a Probability Distribution:
- Non-negativity: The probability of each outcome must be greater than or equal to zero (P(x) ≥ 0 for all x). You cannot have a negative probability.
- Normalization: The sum of the probabilities of all possible outcomes must equal one (ΣP(x) = 1). This reflects the certainty that one of the outcomes will occur.
These two conditions are the cornerstone of any valid probability distribution. If a set of probabilities fails to meet either of these, it's not a valid probability distribution.
Types of Probability Distributions
There are numerous types of probability distributions, each suitable for modeling different types of data and phenomena. Some of the most common include:
1. Discrete Probability Distributions:
Discrete probability distributions deal with discrete random variables – variables that can only take on a finite number of values or a countably infinite number of values. Examples include:
- Bernoulli Distribution: Models a single trial with two possible outcomes (success or failure), each with a fixed probability.
- Binomial Distribution: Describes the probability of getting a certain number of successes in a fixed number of independent Bernoulli trials.
- Poisson Distribution: Models the probability of a given number of events occurring in a fixed interval of time or space if these events occur with a known average rate and independently of the time since the last event.
- Geometric Distribution: Models the probability of the number of trials needed to get the first success in a sequence of independent Bernoulli trials.
- Negative Binomial Distribution: Generalizes the geometric distribution, modeling the number of trials needed to obtain a specified number of successes.
2. Continuous Probability Distributions:
Continuous probability distributions deal with continuous random variables – variables that can take on any value within a given range. Examples include:
- Uniform Distribution: Assigns equal probability to all outcomes within a specified range.
- Normal (Gaussian) Distribution: A bell-shaped curve, incredibly common in many natural phenomena, characterized by its mean and standard deviation.
- Exponential Distribution: Models the time until an event occurs in a Poisson process.
- Gamma Distribution: A generalization of the exponential distribution, often used to model waiting times or the sum of exponential random variables.
- Beta Distribution: Defined on the interval [0, 1], often used to model probabilities or proportions.
- Chi-Squared Distribution: Frequently used in hypothesis testing and statistical inference.
- t-Distribution: Similar to the normal distribution, but with heavier tails, often used in small sample size scenarios.
- F-Distribution: Used in ANOVA (analysis of variance) and other statistical tests.
Identifying a Valid Probability Distribution: Examples and Exercises
Let's examine some examples to illustrate the principles of validating a probability distribution.
Example 1:
Consider the following probability distribution for a discrete random variable X:
X | P(X) |
---|---|
1 | 0.2 |
2 | 0.3 |
3 | 0.5 |
Is this a valid probability distribution?
Solution:
- Non-negativity: All probabilities (0.2, 0.3, 0.5) are greater than or equal to zero. This condition is met.
- Normalization: The sum of probabilities is 0.2 + 0.3 + 0.5 = 1. This condition is also met.
Conclusion: This is a valid probability distribution.
Example 2:
Consider this set of probabilities:
X | P(X) |
---|---|
1 | 0.4 |
2 | 0.3 |
3 | 0.4 |
Is this a valid probability distribution?
Solution:
- Non-negativity: All probabilities are non-negative.
- Normalization: The sum of probabilities is 0.4 + 0.3 + 0.4 = 1.1. This exceeds 1.
Conclusion: This is not a valid probability distribution because it violates the normalization condition.
Example 3:
Consider the following probabilities:
X | P(X) |
---|---|
1 | 0.2 |
2 | -0.1 |
3 | 0.8 |
Is this a valid probability distribution?
Solution:
- Non-negativity: P(X=2) = -0.1, which is negative.
Conclusion: This is not a valid probability distribution because it violates the non-negativity condition.
Example 4: Continuous Case
Let's consider a continuous probability distribution defined by the function f(x) = 2x for 0 ≤ x ≤ 1, and f(x) = 0 elsewhere. Is this a valid probability density function?
Solution:
To check for validity, we need to ensure that the integral of f(x) over its entire range equals 1:
∫₀¹ 2x dx = [x²]₀¹ = 1 - 0 = 1
Also, f(x) is non-negative within the specified range.
Conclusion: This is a valid probability density function.
Advanced Considerations
In more complex scenarios, you might encounter situations with conditional probabilities, joint probability distributions (describing the probability of multiple variables simultaneously), or marginal probability distributions (obtained by summing or integrating over a subset of variables). The principles of non-negativity and normalization still apply, but the calculations can become significantly more involved.
Applications of Probability Distributions
Understanding and applying probability distributions is essential in various fields:
- Statistical Inference: Probability distributions are the foundation of hypothesis testing, confidence intervals, and other statistical inference methods.
- Machine Learning: Many machine learning algorithms rely on probability distributions to model data and make predictions.
- Risk Management: In finance, probability distributions are used to model the risk associated with investments and other financial decisions.
- Quality Control: Probability distributions are used to monitor and control the quality of products and services.
- Simulation: Probability distributions are used to generate random numbers that simulate real-world processes.
Conclusion
Determining if a set of probabilities represents a valid probability distribution boils down to checking two fundamental conditions: non-negativity and normalization. Understanding these conditions is crucial for any statistical analysis and application. This comprehensive guide has provided numerous examples and explanations to solidify your understanding of valid probability distributions and their crucial role across diverse fields. By mastering these concepts, you’ll be well-equipped to tackle more complex statistical problems and build robust analytical models. Remember to practice consistently to develop a strong grasp of these critical concepts. Understanding probability distributions is a cornerstone of statistical literacy and a gateway to unlocking powerful analytical tools.
Latest Posts
Latest Posts
-
Which Statement Accurately Describes A Developing Country
Apr 28, 2025
-
The Presidents Refusal To Spend Money Is Called
Apr 28, 2025
-
Cdl School Bus Test Questions And Answers Pdf
Apr 28, 2025
-
How To Turn A Blooket Into A Quizlet
Apr 28, 2025
-
Pertaining To The Interior Or Lining Of An Artery
Apr 28, 2025
Related Post
Thank you for visiting our website which covers about Which Of The Following Represents A Valid Probability Distribution . We hope the information provided has been useful to you. Feel free to contact us if you have any questions or need further assistance. See you next time and don't miss to bookmark.