Which Of The Following Statements About Reinforcement Is True
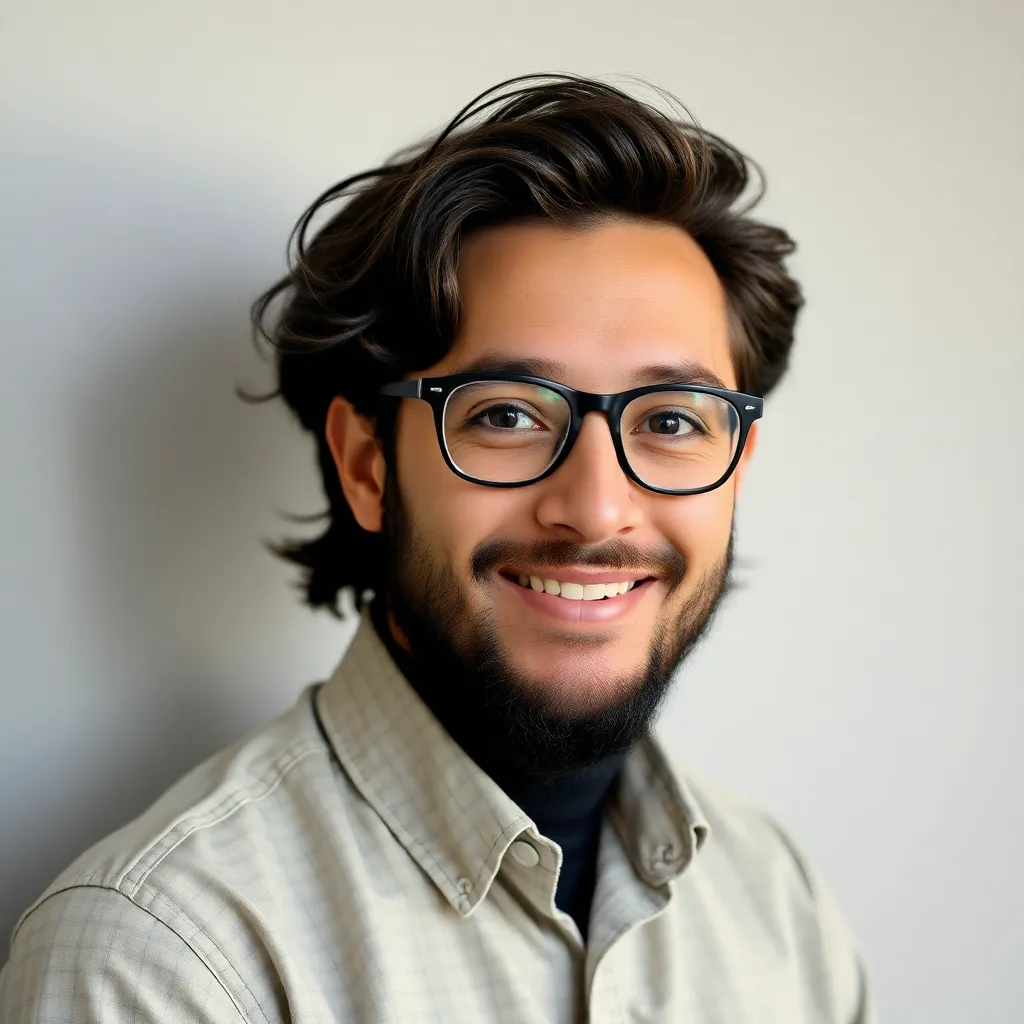
Breaking News Today
Apr 08, 2025 · 6 min read

Table of Contents
Which of the following statements about reinforcement is true? A Comprehensive Guide
Reinforcement learning, a powerful subfield of machine learning, is revolutionizing how we approach problem-solving in diverse areas, from robotics and game playing to resource management and personalized medicine. Understanding the core principles of reinforcement is crucial for anyone venturing into this exciting field. This article will delve deep into the nuances of reinforcement learning, examining common statements and clarifying which hold true, providing a comprehensive understanding of this impactful area.
Understanding the Fundamentals of Reinforcement Learning
Before we dissect the truth behind various statements about reinforcement, let's establish a firm foundation. Reinforcement learning differs significantly from supervised and unsupervised learning. Instead of relying on labeled data or pattern discovery, it focuses on an agent interacting with an environment.
- Agent: This is the learner and decision-maker, striving to achieve a specific goal.
- Environment: This is the external world the agent interacts with. The environment provides feedback to the agent based on its actions.
- Rewards: These are numerical signals indicating the desirability of the agent's actions. Positive rewards encourage the agent to repeat actions, while negative rewards discourage them.
- State: This represents the current situation or condition of the environment.
- Action: This is the choice the agent makes within a given state.
The agent's objective is to learn an optimal policy, a strategy that maximizes its cumulative reward over time. This learning process involves trial and error, with the agent continuously refining its actions based on the feedback it receives from the environment.
Debunking Common Misconceptions: True and False Statements about Reinforcement
Let's analyze several statements frequently encountered regarding reinforcement learning and determine their validity.
Statement 1: Reinforcement learning requires a large, pre-labeled dataset for training.
FALSE. Unlike supervised learning, which heavily relies on labeled datasets, reinforcement learning primarily utilizes interactions with the environment. The agent learns through experience, receiving feedback in the form of rewards. While some approaches might leverage pre-existing knowledge or datasets to initialize the learning process, it's not a strict requirement. The agent's experience, the rewards it receives, and the states it visits are the primary data sources driving learning.
Statement 2: The reward function is always explicitly defined and remains constant throughout the learning process.
FALSE. While a well-defined reward function is essential, it doesn't always have to be explicitly defined from the start. In some cases, the reward function might be implicitly defined through the design of the environment. Furthermore, the reward function can be dynamically adjusted during the learning process, allowing for more complex and adaptive learning scenarios. For instance, reward shaping techniques modify the reward function to guide the agent's learning more efficiently. This dynamic nature makes reinforcement learning exceptionally adaptable to complex problems.
Statement 3: Reinforcement learning guarantees finding the globally optimal solution.
FALSE. Finding the absolute best policy is often computationally infeasible, especially in complex environments with vast state and action spaces. Reinforcement learning algorithms typically aim to find a near-optimal policy—one that performs exceptionally well within a reasonable timeframe and computational budget. The complexity of the problem, the chosen algorithm, and the exploration-exploitation balance all impact the quality of the solution. Many algorithms utilize approximation techniques to navigate this challenge.
Statement 4: Exploration and exploitation are crucial for effective reinforcement learning.
TRUE. This is a cornerstone of reinforcement learning. Exploration involves trying out new actions and states to discover potentially rewarding paths. Exploitation involves repeatedly performing actions known to yield good rewards. A successful agent must strike a balance between these two strategies. Too much exploration can lead to suboptimal performance, while too much exploitation can prevent the discovery of even better strategies. Sophisticated algorithms address this trade-off using techniques like ε-greedy exploration or upper confidence bounds.
Statement 5: Reinforcement learning is only suitable for simple problems with well-defined rules.
FALSE. While early applications of reinforcement learning focused on simpler problems, its capabilities have expanded significantly. Modern reinforcement learning algorithms can handle incredibly complex environments, such as those encountered in robotics, game playing (e.g., AlphaGo), and resource management. The ability to handle partial observability, continuous state spaces, and noisy environments enhances its applicability to various real-world scenarios.
Statement 6: Reinforcement learning is solely based on trial and error; no prior knowledge can be incorporated.
FALSE. While trial and error is fundamental, prior knowledge can significantly accelerate the learning process and improve performance. Techniques such as transfer learning and curriculum learning allow agents to leverage knowledge gained from previous tasks or simpler versions of the current task. This incorporation of prior knowledge can drastically reduce the learning time and improve the final solution's quality. This capability makes reinforcement learning particularly attractive in domains with substantial prior understanding.
Statement 7: All reinforcement learning algorithms converge to the same optimal policy.
FALSE. Different reinforcement learning algorithms have varying strengths and weaknesses. They approach the problem of finding an optimal policy using different methods and have different computational demands. The choice of algorithm significantly influences the speed of convergence and the quality of the solution obtained. Factors like the nature of the environment, state and action spaces, and computational resources often dictate the most appropriate algorithm.
Statement 8: The agent in reinforcement learning always has complete information about the environment.
FALSE. Many real-world problems involve partially observable environments, where the agent doesn't have complete information about the current state. This adds significant complexity, requiring the agent to learn to make decisions based on incomplete information. Dealing with partial observability is a significant research area, and various techniques have been developed to address this challenge, including techniques that build internal models of the environment to infer unobserved states.
Advanced Concepts and Applications
Beyond the fundamental statements, several advanced concepts further enrich our understanding of reinforcement learning:
- Deep Reinforcement Learning: This combines the power of deep neural networks with reinforcement learning, enabling the learning of complex, high-dimensional policies. This combination has led to breakthroughs in game playing, robotics, and natural language processing.
- Hierarchical Reinforcement Learning: This breaks down complex tasks into smaller subtasks, making the learning process more manageable and efficient.
- Inverse Reinforcement Learning: This aims to infer the reward function from observing an expert's behavior. This is valuable when defining the reward function explicitly is difficult or impossible.
- Multi-Agent Reinforcement Learning: This deals with scenarios where multiple agents interact with each other and the environment, requiring coordination and cooperation or competition.
These advanced techniques extend reinforcement learning's capabilities to tackle increasingly complex and multifaceted problems.
Conclusion
Reinforcement learning is a powerful and versatile tool, capable of solving a wide range of problems across diverse fields. Understanding the nuances of this approach, distinguishing between true and false statements about its capabilities, and grasping its advanced concepts are key to effectively leveraging its potential. By appreciating the balance between exploration and exploitation, acknowledging the limitations of finding globally optimal solutions, and recognizing the importance of prior knowledge incorporation, we can unlock the transformative power of reinforcement learning in diverse real-world applications. The ongoing research and development in this field continually expand its horizons, promising even more impressive advancements in the future.
Latest Posts
Latest Posts
-
Respiratory Physiology Is Primarily The Study Of
Apr 18, 2025
-
The Human Arterial And Venous Systems Are Diagrammed
Apr 18, 2025
-
Nosotras Ganas Mirar Television
Apr 18, 2025
-
A Gas Condenses To A Liquid Releasing Heat
Apr 18, 2025
-
The Amount Of Matter In An Object
Apr 18, 2025
Related Post
Thank you for visiting our website which covers about Which Of The Following Statements About Reinforcement Is True . We hope the information provided has been useful to you. Feel free to contact us if you have any questions or need further assistance. See you next time and don't miss to bookmark.